Facial Expression Animation by Landmark Guided Residual Module
IEEE Transactions on Affective Computing(2023)
摘要
We study the problem of facial expression animation from a still image according to a driving video. This is a challenging task as expression motions are non-rigid and very subtle to be captured. Existing methods mostly fail to model these subtle expression motions, leading to the lack of details in their animation results. In this paper, we propose a novel facial expression animation method based on generative adversarial learning. To capture the subtle expression motions, Landmark guided Residual Module (LRM) is proposed to model detailed facial expression features. Specifically, residual learning is conducted at both coarse and fine levels conditioned on facial landmark heatmaps and landmark points respectively. Furthermore, we employ a consistency discriminator to ensure the temporal consistency of the generated video sequence. In addition, a novel metric named Emotion Consistency Metric is proposed to evaluate the consistency of facial expressions in the generated sequences with those in the driving videos. Experiments on MUG-Face, Oulu-CASIA and CAER datasets show that the proposed method can generate arbitrary expression motions on the source still image effectively, which are more photo-realistic and consistent with the driving video compared with results of state-of-the-art methods.
更多查看译文
关键词
Facial expression animation,generative adversarial network (GAN),landmark guided residual module (lrm),emotion consistency metric (ECM)
AI 理解论文
溯源树
样例
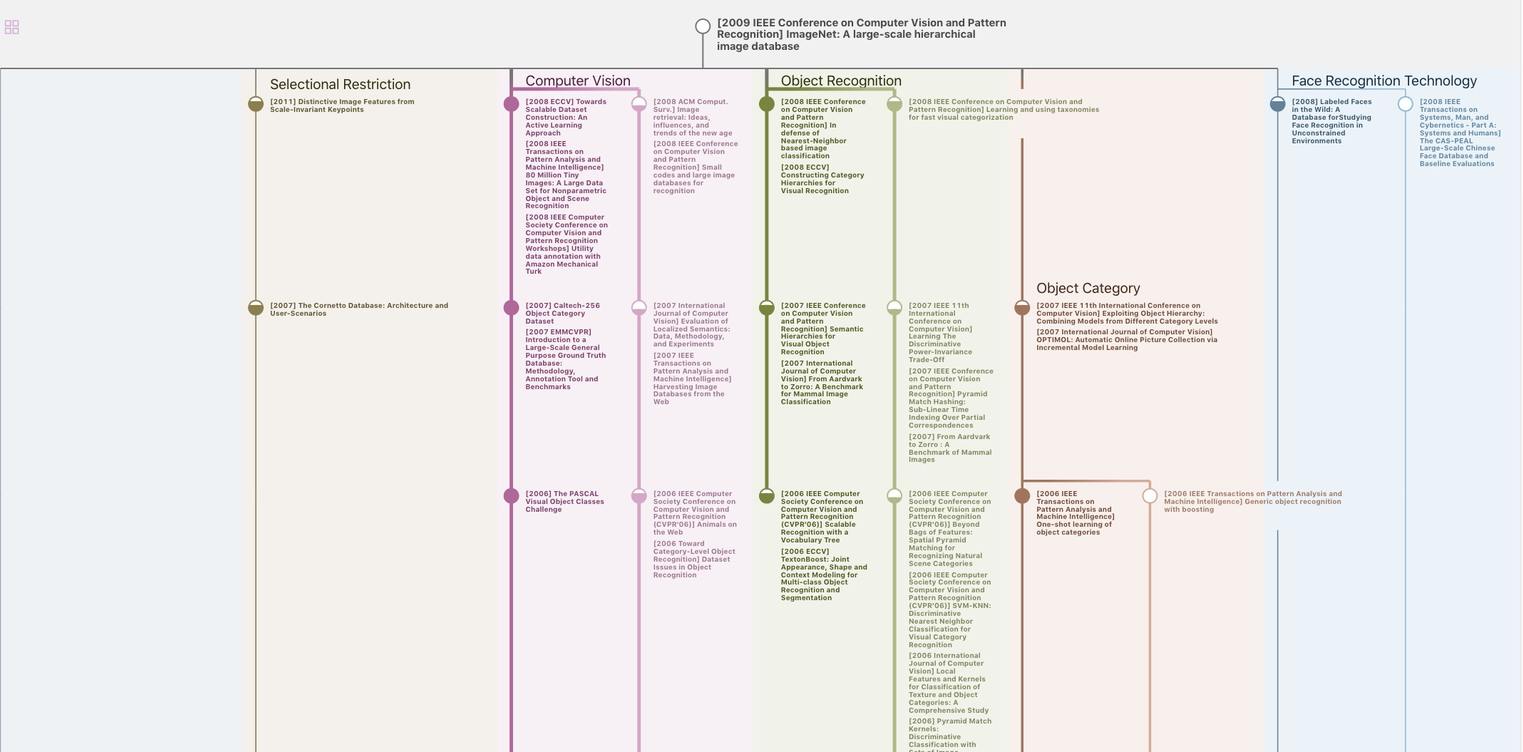
生成溯源树,研究论文发展脉络
Chat Paper
正在生成论文摘要