A Reinforcement Learning Approach for Enacting Cautious Behaviours in Autonomous Driving System: Safe Speed Choice in the Interaction With Distracted Pedestrians
IEEE TRANSACTIONS ON INTELLIGENT TRANSPORTATION SYSTEMS(2022)
摘要
Driving requires the ability to handle unpredictable situations. Since it is not always possible to predict an impending danger, a good driver should preventively assess whether a situation has risks and adopt a safe behavior. Considering, in particular, the possibility of a pedestrian suddenly crossing the road, a prudent driver should limit the traveling speed. We present a work exploiting reinforcement learning to learn a function that specifies the safe speed limit for a given artificial driver agent. The safe speed function acts as a behavioral directive for the agent, thus extending its cognitive abilities. We consider scenarios where the vehicle interacts with a distracted pedestrian that might cross the road in hard-to-predict ways and propose a neural network mapping the pedestrian's context onto the appropriate traveling speed so that the autonomous vehicle can successfully perform emergency braking maneuvers. We discuss the advantages of developing a specialized neural network extension on top of an already functioning autonomous driving system, removing the burden of learning to drive from scratch while focusing on learning safe behavior at a highlevel. We demonstrate how the safe speed function can be learned in simulation and then transferred into a real vehicle. We include a statistical analysis of the network's improvements compared to the original autonomous driving system. The code implementing the presented network is available at https://githuh.com/tonegas/safe-speed-neural-network with MIT license and at https://zenodo.org/communities/dreams4cars.
更多查看译文
关键词
Vulnerable road users,neural networks,reinforcement learning,transfer learning,autonomous driving,intelligent speed adaptation
AI 理解论文
溯源树
样例
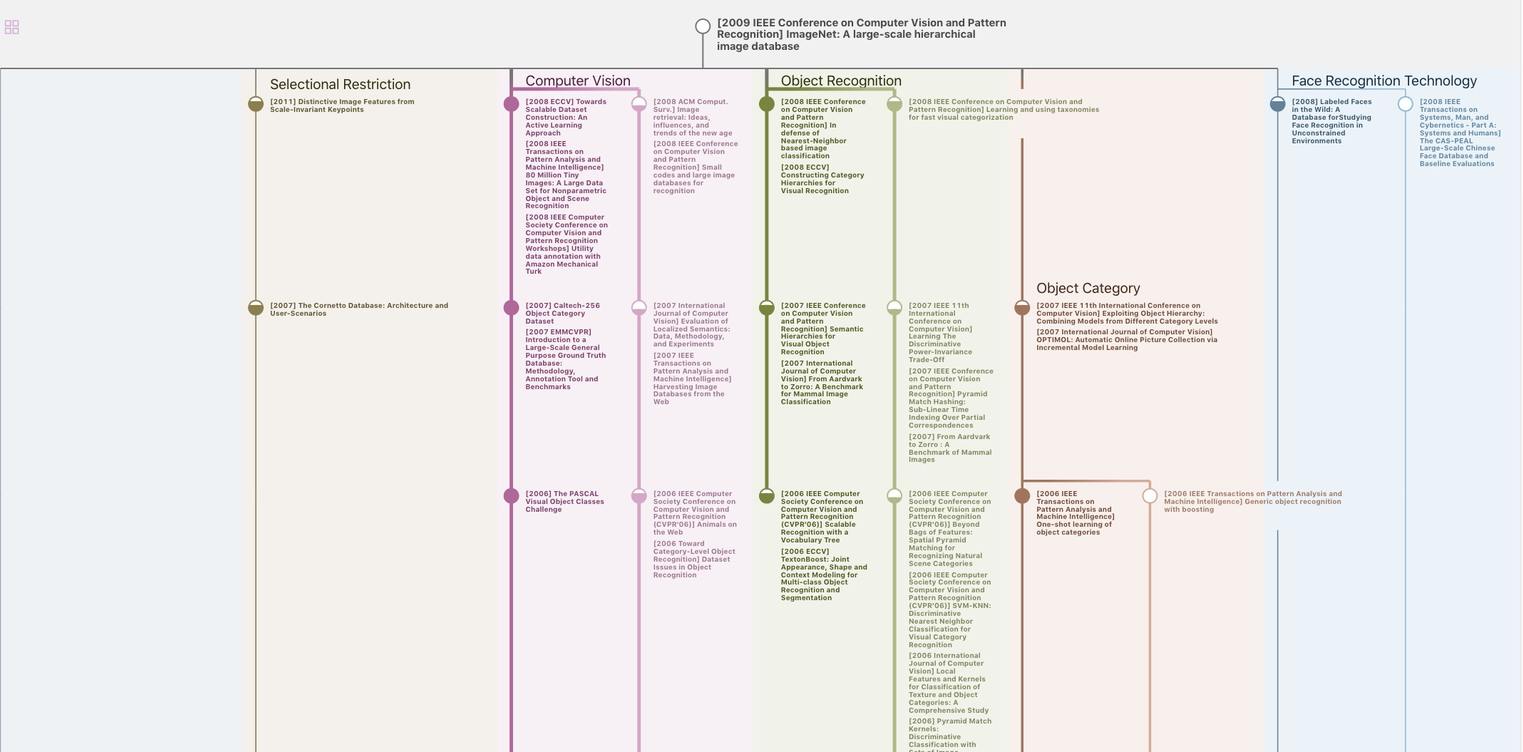
生成溯源树,研究论文发展脉络
Chat Paper
正在生成论文摘要