Data Clustering based on Gaussian Mixture Model and Expectation- Maximization Algorithm for Data-driven Structural Health Monitoring System
International Journal of Integrated Engineering(2021)
摘要
Data groups generated by a system often inherit dynamics characteristics unique in data distribution parameters. A degradation in structural health can affect the dynamic behavior hence the probability distribution parameters. Based on the probabilistic and Expectation-Maximization (EM) algorithm, Gaussian Mixture Model (GMM), one can cluster data groups that may overlap with different data groups based on different orientations and shapes. This article explores GMM probabilistic model applied on vibration data set generated by aircraft wing box structure for Structural Health Monitoring (SHM) application. In the data processing stage, the high dimensional data is transformed into lower dimensions using Kernel Principal Component Analysis (KPCA). KPCA transforms the continuous signal into discrete data, allowing the ellipsoids' fitting (clusters) on the data spread. Based on the baseline data set (undamaged structural condition) and several components (loading class and damage class), the fitting is performed using GMM driven by EM. This paper shows that GMM-EM based data clustering model is an effective clustering probability model in fitting the data density in the presence of operational variations. It highlights clustering of reduced vibration data using KPCA in the interest of SHM based on the baseline’s initial parameters.
更多查看译文
关键词
Gaussian mixture model, expectation maximization, structural health monitoring, kernel principal component analysis
AI 理解论文
溯源树
样例
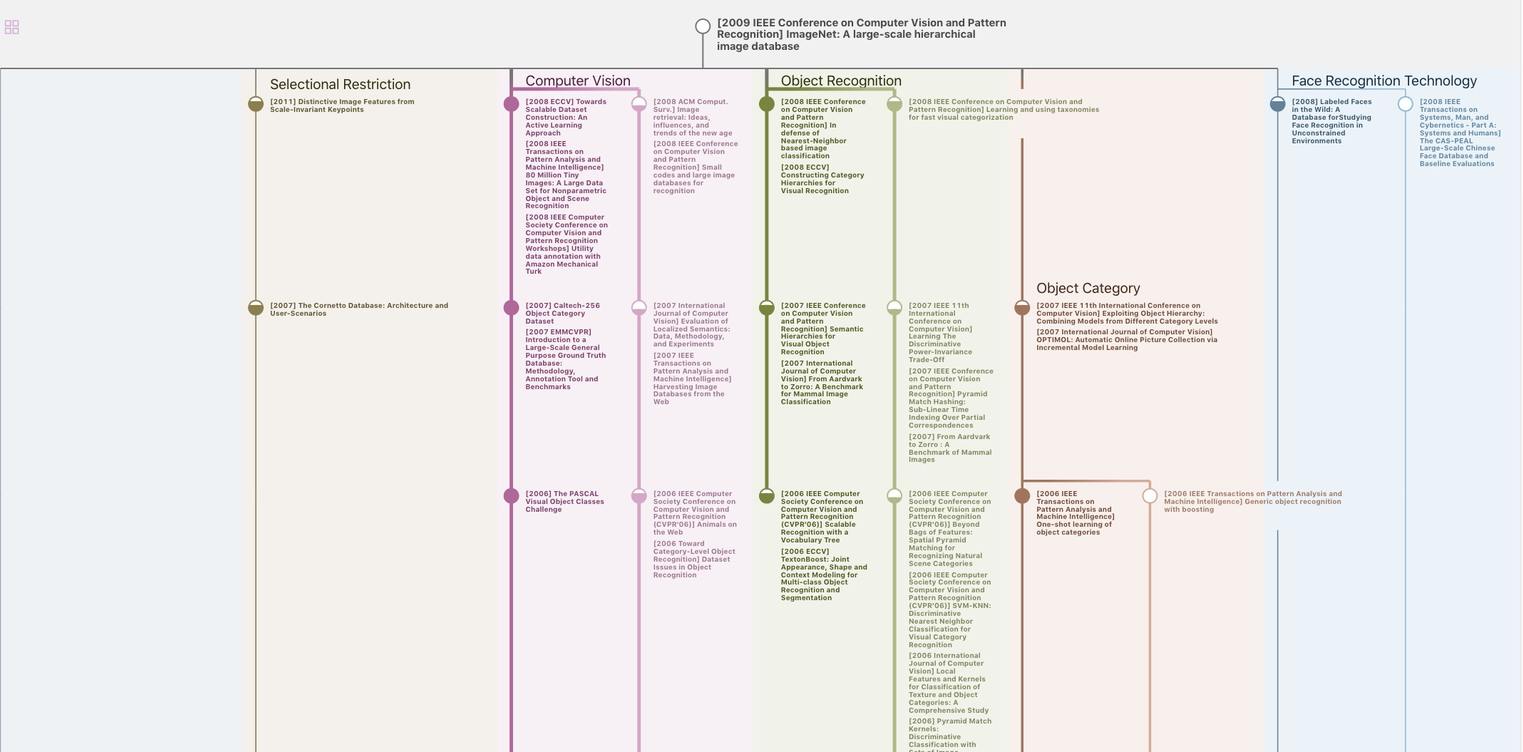
生成溯源树,研究论文发展脉络
Chat Paper
正在生成论文摘要