Evaluation of three potential machine learning algorithms for predicting the velocity and turbulence intensity of a wind turbine wake
RENEWABLE ENERGY(2022)
摘要
In this paper, three machine learning (ML) algorithms, Support Vector Regression (SVR), Artificial Neural Networks (ANN), and Extreme Gradient Boosting (XGBoost), are validated to estimate the velocity and turbulence intensity of a wind turbine's wake at distinct downstream distances. To this end, a series of high-fidelity numerical simulations for the NREL Phase VI wind turbine is carried out to generate training and test datasets for the three machine learning algorithms. The predicted wake velocity and turbulence intensity from the ML models are also contrasted with significant existing analytical wake models. Machine learning algorithms estimate velocity and turbulence intensity in the wake in a way commensurate to the Computational Fluid Dynamics (CFD) simulations while running at a similar pace as low-fidelity wake models. The results demonstrate that machine learning-based algorithms can predict velocity and turbulence intensity better with higher precision than the traditional analytical wake models. (C) 2021 Elsevier Ltd. All rights reserved.
更多查看译文
关键词
Wake velocity,Turbulence intensity,Support vector regression (SVR),Artificial neural networks (ANN),eXtreme gradient boosting (XGBoost)
AI 理解论文
溯源树
样例
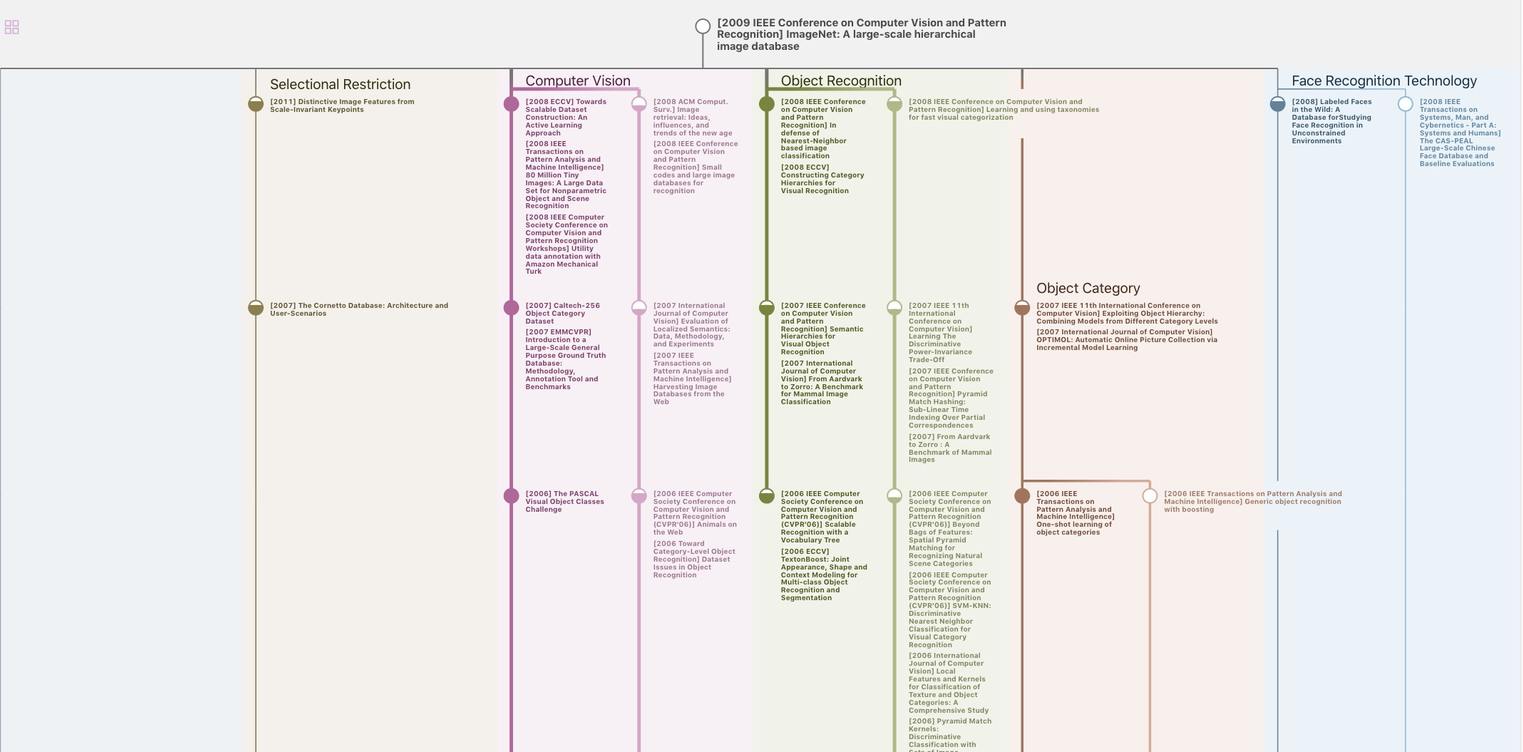
生成溯源树,研究论文发展脉络
Chat Paper
正在生成论文摘要