A Comparison of the Performance of 2D and 3D Convolutional Neural Networks for Subsea Survey Video Classification
OCEANS 2021: San Diego – Porto(2021)
摘要
Utilising deep learning image classification to automatically annotate subsea pipeline video surveys can facilitate the tedious and labour-intensive process, resulting in significant time and cost savings. However, the classification of events on subsea survey videos (frame sequences) by models trained on individual frames have been proven to vary, leading to inaccuracies. The paper extends previous work on the automatic annotation of individual subsea survey frames by comparing the performance of 2D and 3D Convolutional Neural Networks (CNNs) in classifying frame sequences. The study explores the classification of burial, exposure, free span, field joint, and anode events. Sampling and regularization techniques are designed to address the challenges of an underwater inspection video dataset owing to the environment. Results show that a 2D CNN with rolling average can outperform a 3D CNN, achieving an Exact Match Ratio of 85% and F1-Score of 90%, whilst being more computationally efficient.
更多查看译文
关键词
Deep Learning, Subsea Inspection, Video Classification, Underwater Pipelines
AI 理解论文
溯源树
样例
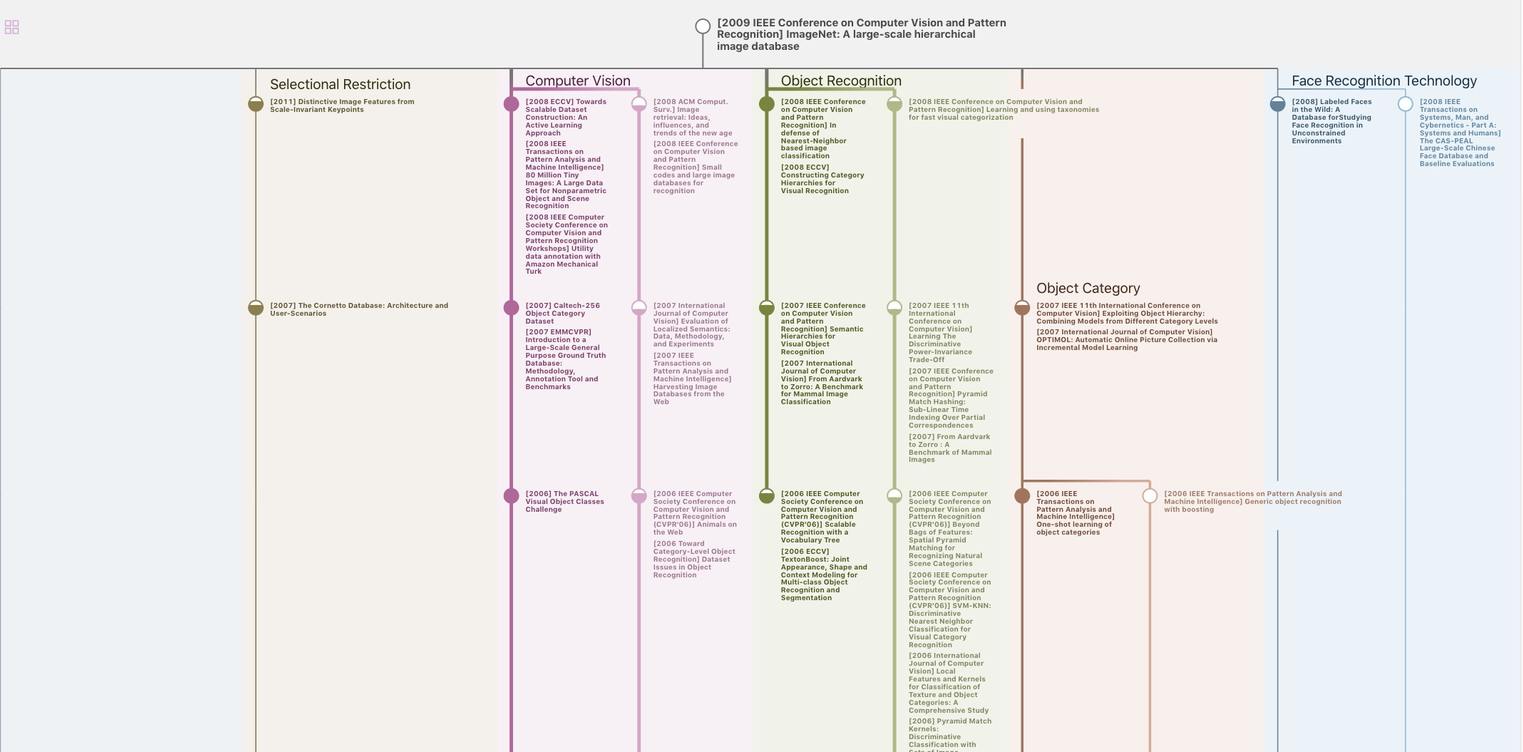
生成溯源树,研究论文发展脉络
Chat Paper
正在生成论文摘要