Combining Dimensionality Reduction Methods with Neural Networks for Realized Volatility Forecasting
Social Science Research Network(2021)
摘要
The application of artificial neural networks to finance has recently received a great deal of attention from both investors and researchers, particularly as a forecasting tool. However, when dealing with a large number of predictors, these methods may overfit the data and provide poor out-of-sample forecasts. Our paper addresses this issue by employing two different approaches to predict realized volatility. On the one hand, we use a two-step procedure where several dimensionality reduction methods, such as Bayesian Model Averaging (BMA), Principal Component Analysis (PCA), and Least Absolute Shrinkage and Selection Operator (Lasso), are employed in the initial step to reduce dimensionality. The reduced samples are then combined with artificial neutral networks. On the other hand, we implement two single-step regularized neural networks that can shrink the input weights to zero and effectively handle high-dimensional data. Our findings on the volatility of different stock asset prices indicate that the reduced models outperform the compared models without regularization in terms of predictive accuracy.
更多查看译文
关键词
Realized volatility,Artificial neural network,Machine-learning,PCA Method,Bayesian model averaging,C13,C14,G10
AI 理解论文
溯源树
样例
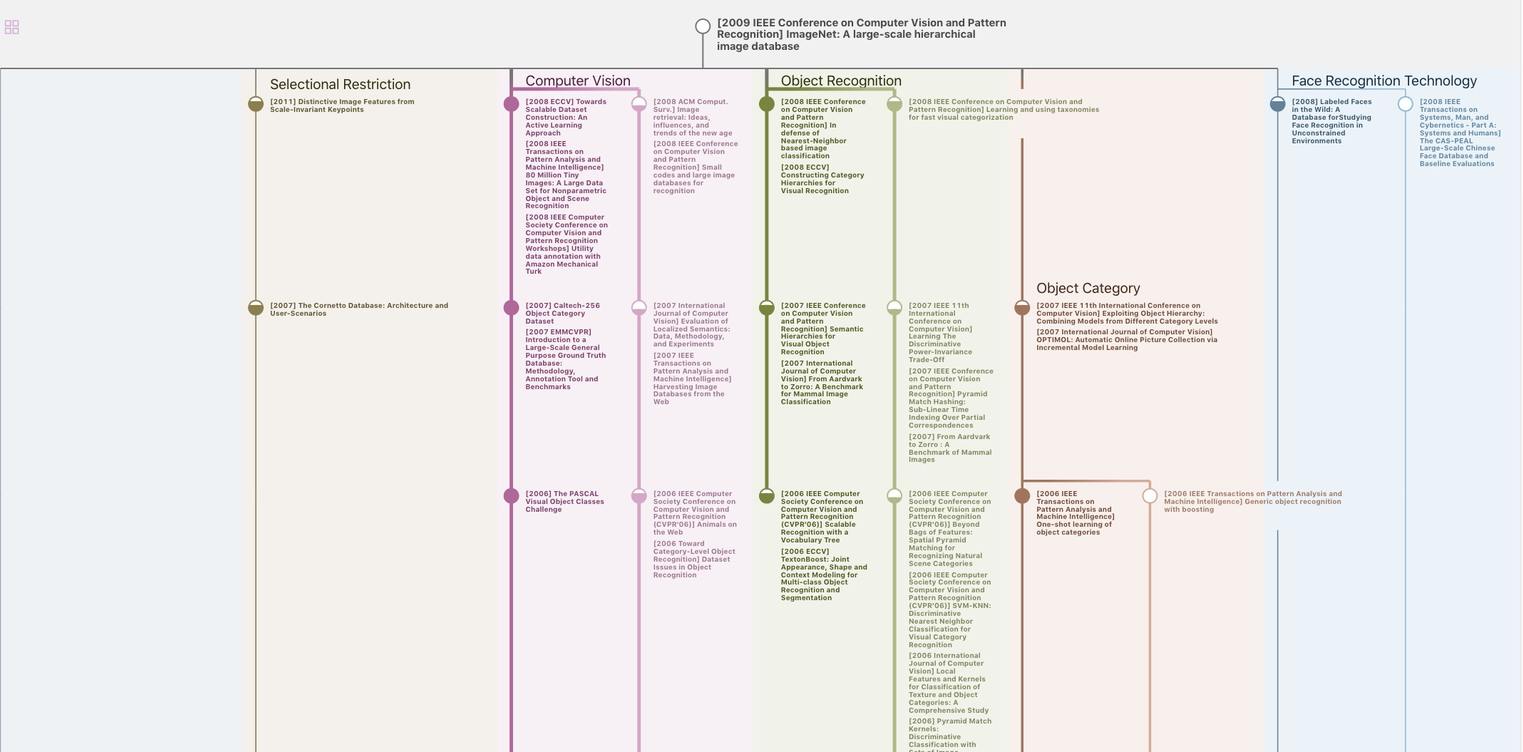
生成溯源树,研究论文发展脉络
Chat Paper
正在生成论文摘要