Multivariate correlation matrix-based deep learning model with enhanced heuristic optimization for short-term traffic forecasting
IEEE Transactions on Knowledge and Data Engineering(2021)
摘要
Accurately capturing the spatial correlations of traffic network significantly benefits short-term traffic forecasting. Some existing works represent spatial correlations in a simple one-dimensional space, but they cannot represent the real spatial correlations among sensors comprehensively. The other existing works represent the spatial correlations through grid-based method, but the local correlation of constructed spatial map is too superficial to extract deep spatial features effectively. Therefore, a novel deep learning model is proposed, which aims to represent the spatial correlations more effectively through a new correlation matrix structure. In the proposed model, the correlations among sensors are calculated from multiple perspectives to construct the speed, volume, and occupancy correlation matrices respectively. Then, considering that highly correlated sensors are close in the spatial dimension, an enhanced heuristic optimization algorithm is proposed to evolve these three correlation matrices into optimal ones by reorganizing the highly correlated sensors into each others neighborhood. Finally, the three optimal correlation matrices are combined to form a three-dimensional multivariate correlation matrix characterized by locally high correlation, which is beneficial to exploit the deep spatial features of traffic network. The experiments show that the proposed model has better accuracy and stability than other commonly used baseline models.
更多查看译文
关键词
Traffic forecasting,deep learning,genetic algorithm,convolutional long short-term memory,convolutional neural network
AI 理解论文
溯源树
样例
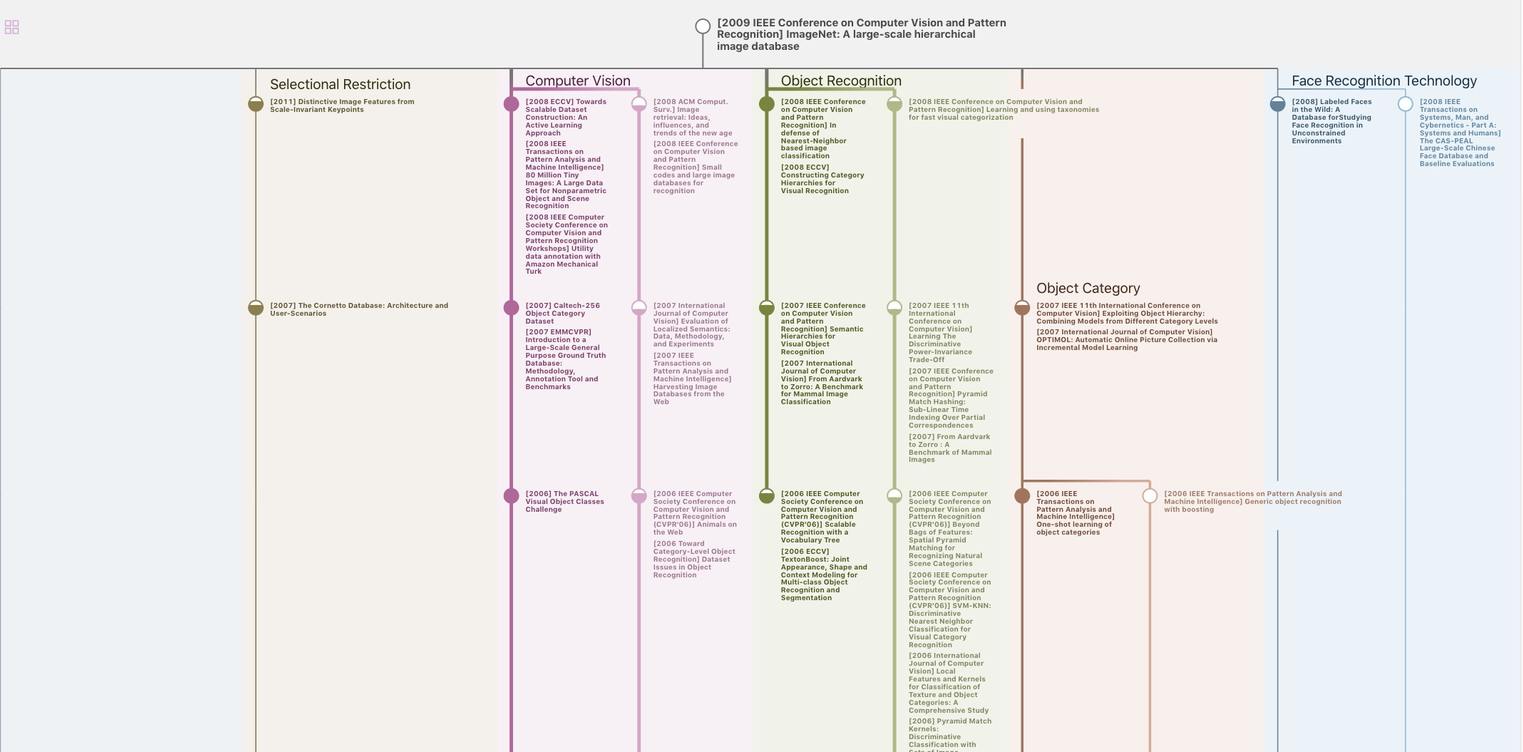
生成溯源树,研究论文发展脉络
Chat Paper
正在生成论文摘要