Clustering with Principal Component Analysis and Fuzzy Subtractive Clustering Using Membership Function Exponential and Hamming Distance
IOP Conference Series: Materials Science and Engineering(2021)
摘要
The problem of dimension reduction in multivariate data is how to obtain a smaller number of variables but still be able to maintain most of the information contained in the data. One method that can be used is principal component analysis (PCA). Principal component analysis (PCA) is a technique used to reduce the dimensions of data consisting of several dependent variables while maintaining the variance in the data. PCA can be used to stabilize measurements in statistical analysis, one of which is cluster analysis. Fuzzy clustering is a method of grouping based on membership values that include fuzzy sets as a basis for weighting for grouping. One method of fuzzy clustering is Fuzzy Subtractive Clustering (FSC). The method used in this study is PCA and FSC. The purpose of this study is to compare the most optimal cluster results using PCAFSC and FSC methods. The results obtained indicate that the clustering using the PCAFSC method is better than the FSC method.
更多查看译文
AI 理解论文
溯源树
样例
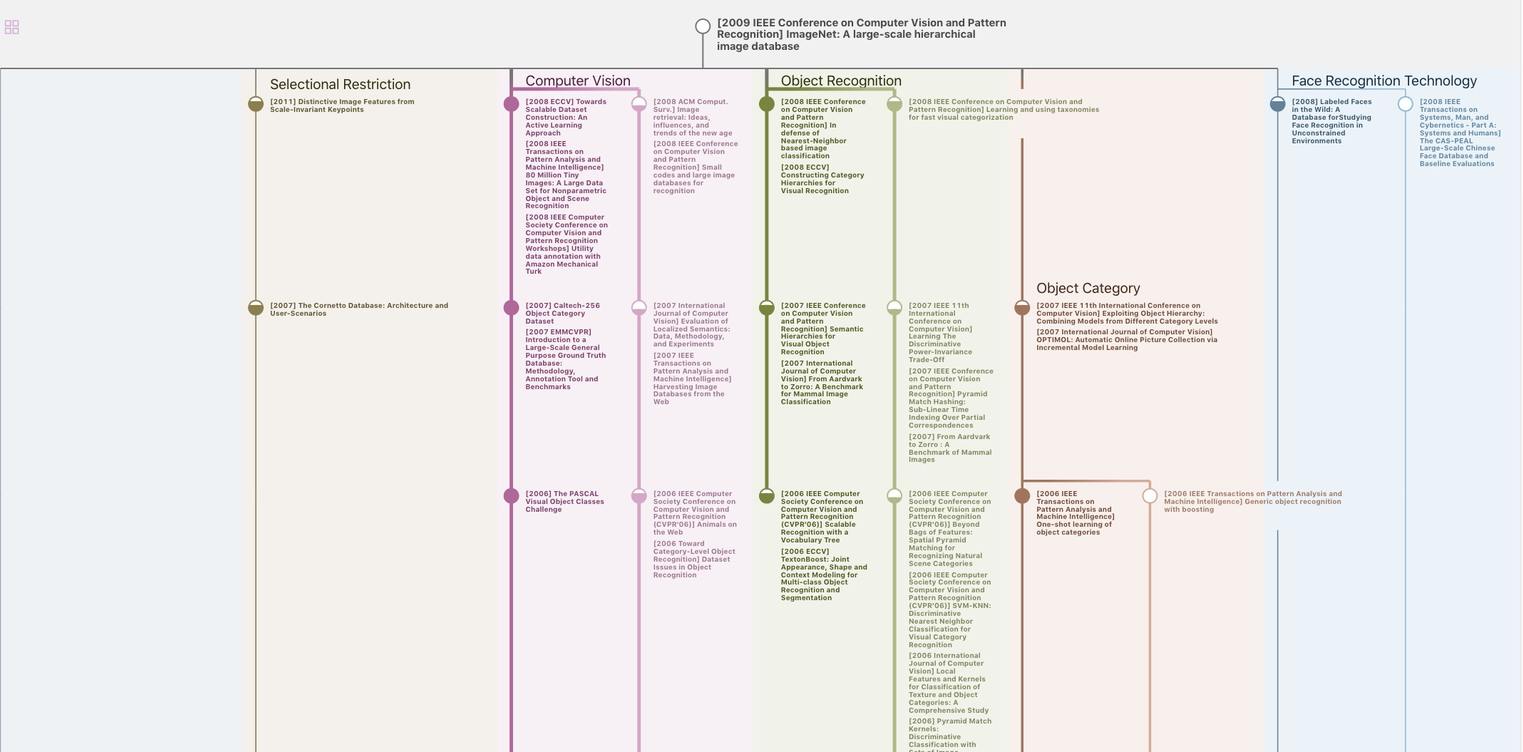
生成溯源树,研究论文发展脉络
Chat Paper
正在生成论文摘要