Fusion of Shallow and Deep features for Gait Recognition Through Multiple Walking Views
chinese control conference(2021)
摘要
Gait recognition has attracted widespread attention in recent years, as the gait of humans has a strong discriminative ability even under low-quality image sequences captured at a distance. This paper describes a gait recognition algorithm by combining shallow and deep features through multiple walking views. For each walking sequence, the binary silhouettes are characterized with width features as shallow parameters, including arm silhouette widths and leg silhouette widths, which can implicitly reflect the temporal changes of silhouette shape. In addition, using deep transfer learning algorithm, deep gait features can be represented as the spatial variation of pixel points in the human silhouette, which can represent the spatial changes among silhouette images. Both shallow and deep features can be used separately for gait recognition using Support Vector Machine classifier (SVM). They are fused on the decision level to improve the gait recognition performance. The fusion of two different kinds of features provides a comprehensive characterization of gait dynamics, which is not sensitive to the walking views variation. CASIA-B dataset is used to perform the proposed method. A better performance of this method compared to the related work methods has been shown after experimental result in terms of rate accuracy of identification and classification.
更多查看译文
关键词
decision fusion,shallow features,deep features,transfer learning
AI 理解论文
溯源树
样例
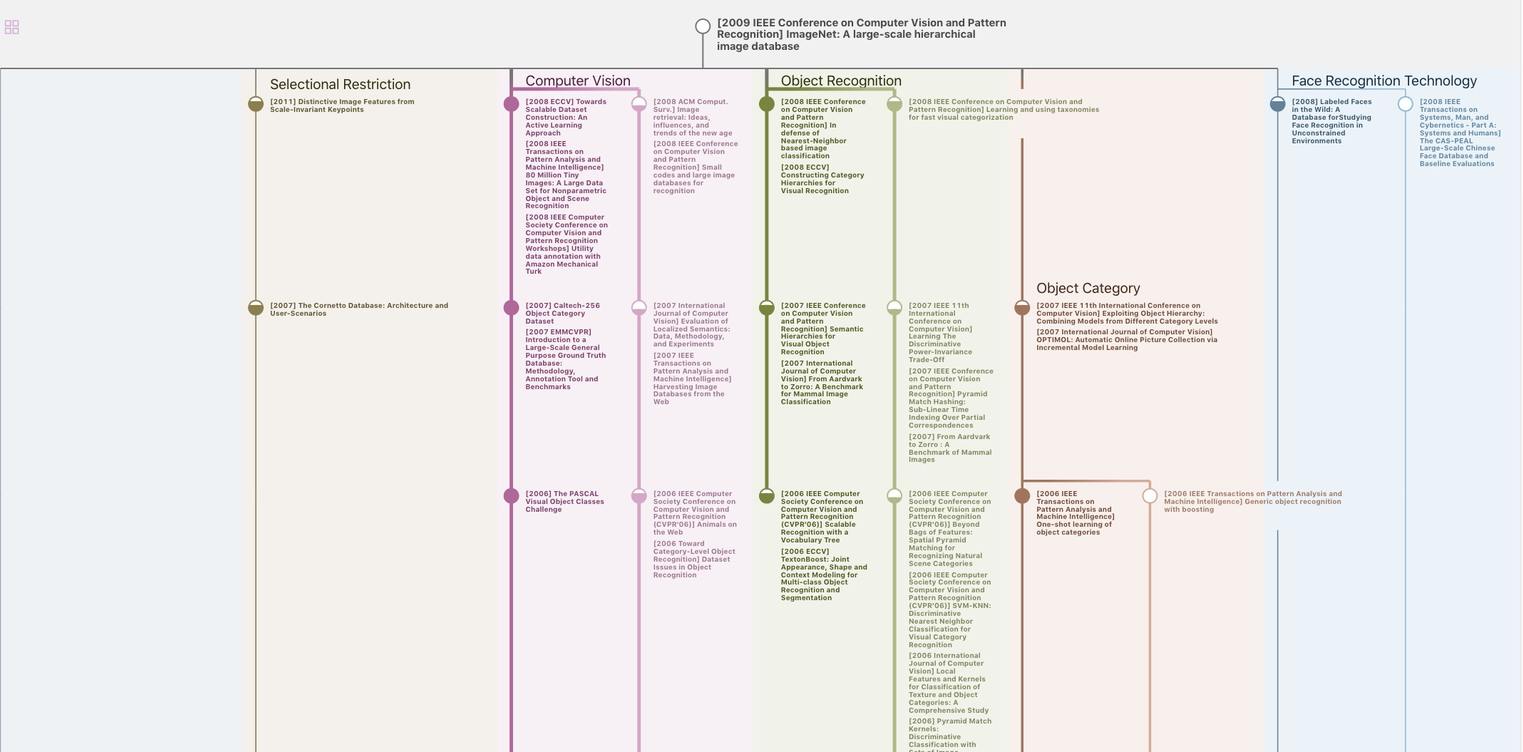
生成溯源树,研究论文发展脉络
Chat Paper
正在生成论文摘要