Time series prediction for EMS with machine learning
2019 International Conference on Engineering, Science, and Industrial Applications (ICESI)(2019)
摘要
One of the key purposes of an Energy Management System (EMS) is the optimisation of energy costs, which relies on accurate prediction of their components' behaviour in the short-term future. EMS operates various types of devices that consume energy. For each device, the short-term prediction of its parameters is required for effective EMS. A machine learning approach is proposed for predicting the behaviour of EMS devices. For this purpose, a Long Short-Term Memory (LSTM) Recurrent Neural Network (RNN) is used, where multivariate time-series data serve as input. For each device, a new model is trained with the corresponding measurements of the devices' parameters and local environment variables, which are provided as time-series with the same time-step. One of the time series is selected as the predicted output. In the experiments, the proposed approach was applied to train a model for predicting the temperature in a water heater, based on the time-series of water temperature and heater power consumption. The water temperature was estimated successfully for the short-term future, based on the input temperature and planned heater action. For the two-step prediction, the RMSE of 0.006 K was calculated between the predicted and measured temperatures.
更多查看译文
关键词
energy costs,short-term prediction,machine learning approach,EMS devices,multivariate time-series data,water temperature,two-step prediction,time series prediction,energy management system,long short-term memory recurrent neural network,LSTM,RNN,RMSE,energy consumption,heater power consumption,water heater,temperature measurement,temperature prediction
AI 理解论文
溯源树
样例
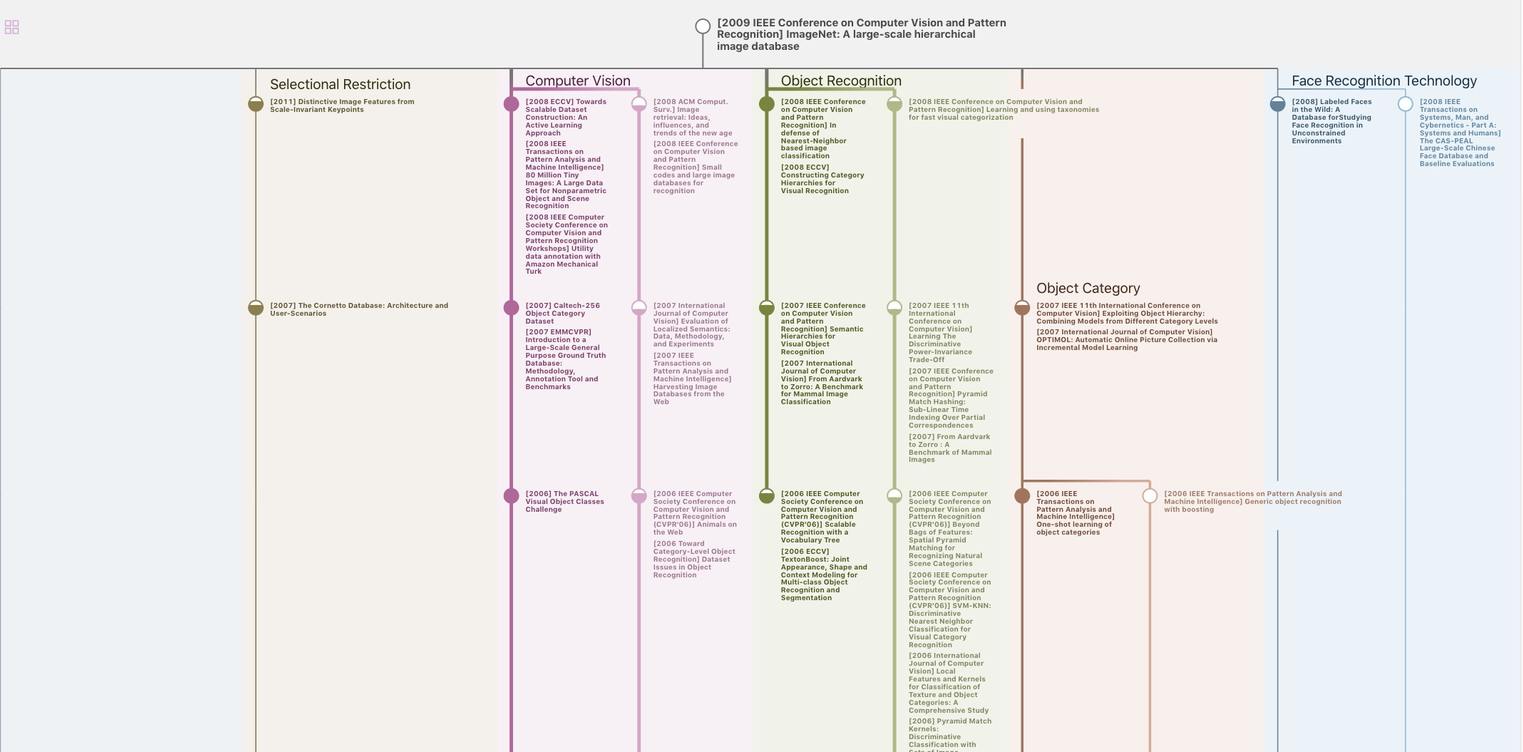
生成溯源树,研究论文发展脉络
Chat Paper
正在生成论文摘要