Object detection in crowded scenes via joint prediction
Defence Technology(2023)
摘要
Detecting highly-overlapped objects in crowded scenes remains a challenging problem, especially for one-stage detector. In this paper, we extricate YOLOv4 from the dilemma in a crowd by fine-tuning its detection scheme, named YOLO-CS. Specifically, we give YOLOv4 the power to detect multiple objects in one cell. Center to our method is the carefully designed joint prediction scheme, which is executed through an assignment of bounding boxes and a joint loss. Equipped with the derived joint-object augmentation (DJA), refined regression loss (RL) and Score-NMS (SN), YOLO-CS achieves competitive detection performance on CrowdHuman and CityPersons benchmarks compared with state-of-the-art detectors at the cost of little time. Furthermore, on the widely used general benchmark COCO, YOLO-CS still has a good performance, indicating its robustness to various scenes.
更多查看译文
关键词
Machine learning,Object detection,YOLOv4,Crowded scenes,Computer vision
AI 理解论文
溯源树
样例
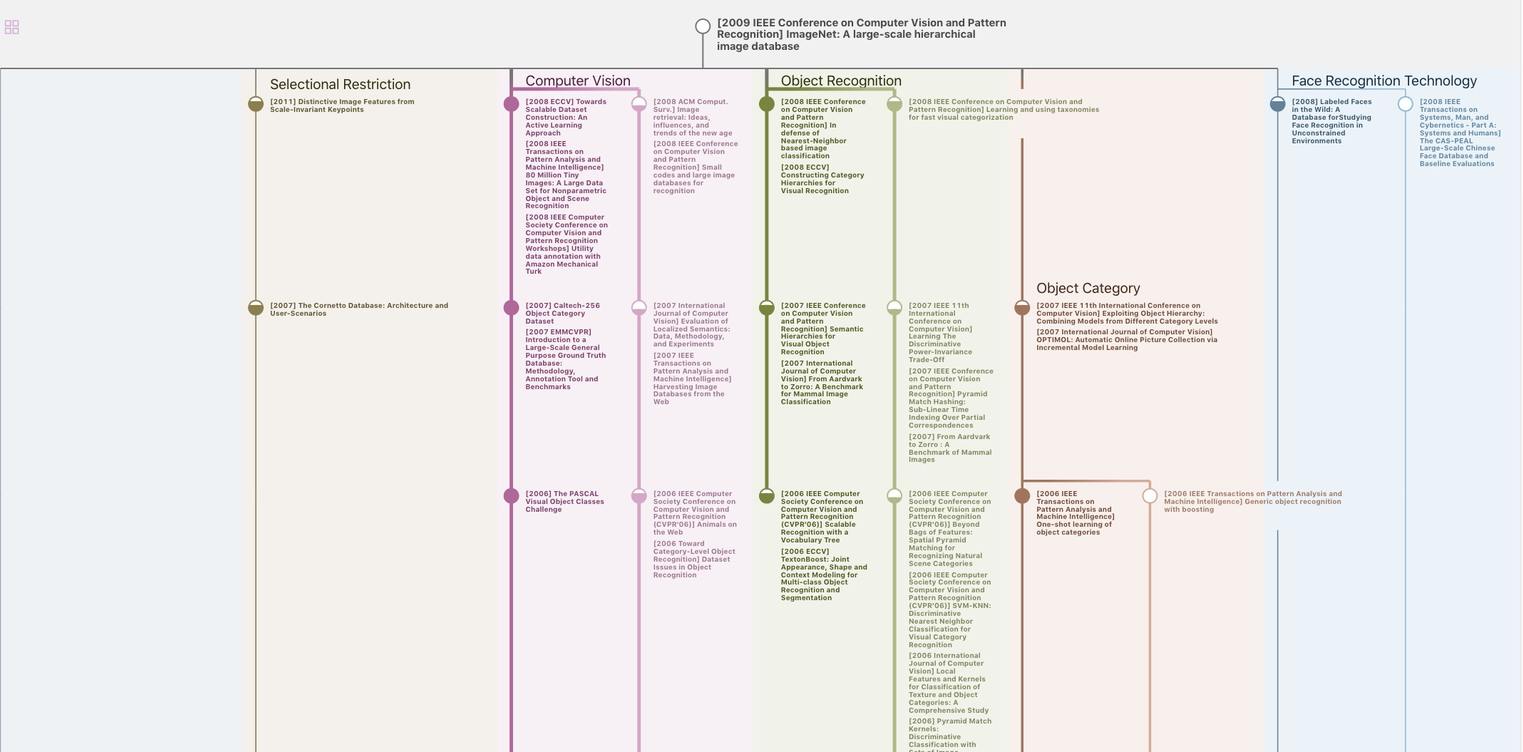
生成溯源树,研究论文发展脉络
Chat Paper
正在生成论文摘要