Graph-Embedded Convolutional Neural Network for Image-Based EEG Emotion Recognition
IEEE Transactions on Emerging Topics in Computing(2022)
摘要
Emotion recognition from electroencephalograph (EEG) signals has long been essential for affective computing. In this article, we evaluate EEG emotion recognition by converting EEG signals from multiple channels into images such that richer spatial information can be considered and the question of EEG-based emotion recognition can be converted into image recognition. To this end, we propose a novel method to generate continuous images from discrete EEG signals by introducing offset variables following a Gaussian distribution for each EEG channel to alleviate the biased electrode coordinates during image generation. In addition, a novel graph-embedded convolutional neural network (GECNN) method is proposed to combine the local convolutional neural network (CNN) features with global functional features to provide complementary emotion information. In GECNN, the attention mechanism is applied to extract more discriminative local features. Simultaneously, dynamical graph filtering explores the intrinsic relationships between different EEG regions. The local and global functional features are finally fused for emotion recognition. Extensive experiments in subject-dependent and subject-independent protocols are conducted to evaluate the performance of the proposed GECNN model on four datasets, i.e., SEED, SDEA, DREAMER, and MPED.
更多查看译文
关键词
EEG emotion recognition,graph convolutional neural network,EEG image generation,graph embedded convolutional neural network
AI 理解论文
溯源树
样例
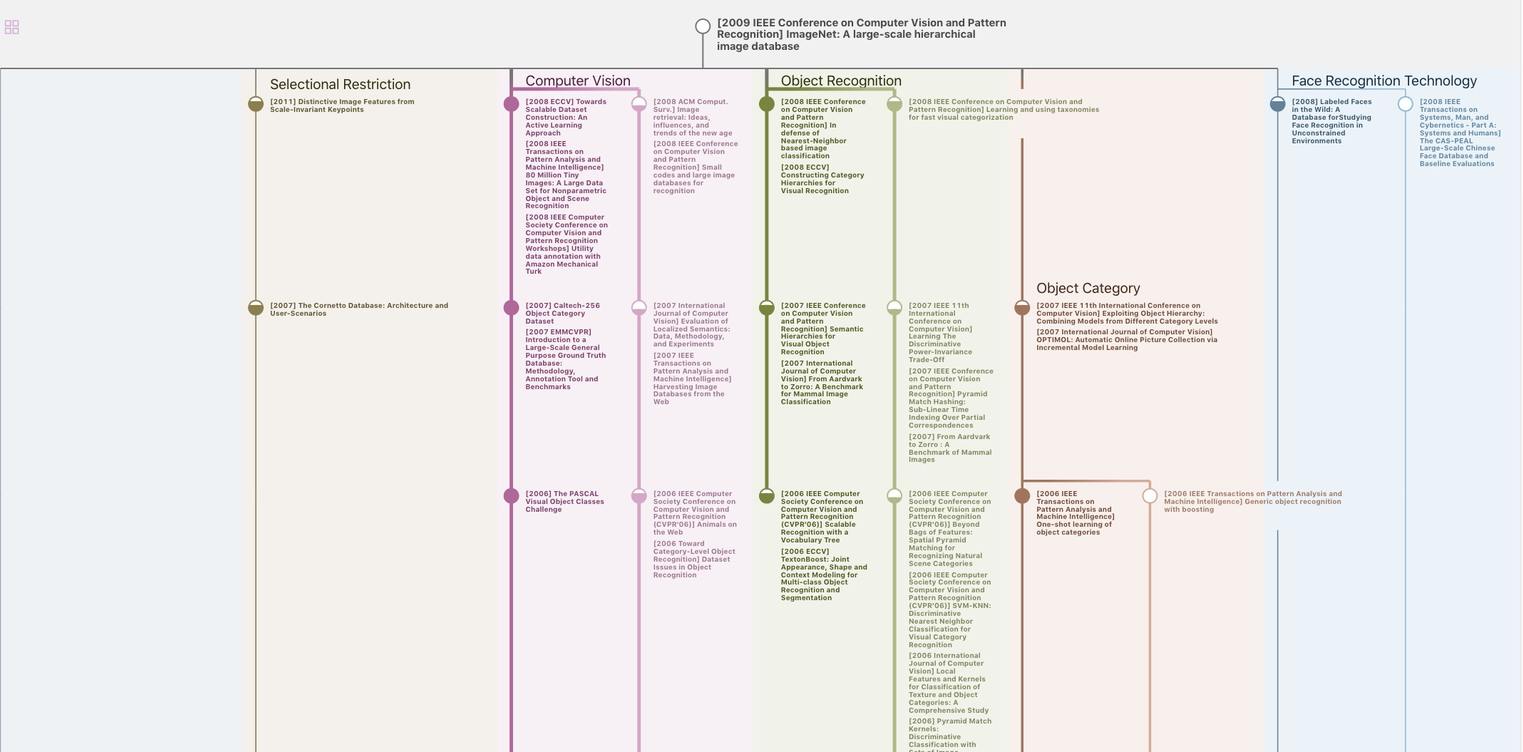
生成溯源树,研究论文发展脉络
Chat Paper
正在生成论文摘要