Deterministic policy gradient algorithms for semi-Markov decision processes
INTERNATIONAL JOURNAL OF INTELLIGENT SYSTEMS(2022)
摘要
A large class of sequential decision-making problems under uncertainty, with broad applications from preventive maintenance to event-triggered control can be modeled in the framework of semi-Markov decision processes (SMDPs). Unlike Markov decision processes (MDPs), SMDPs are underexplored in the online and reinforcement learning (RL) settings. In this paper, we extend the well-known deterministic policy gradient (DPG) theorem in MDPs to SMDPs under average-reward criterion. The existing stochastic policy gradient methods not only require, in general, a large number of samples for training, but they also suffer from high variance in the gradient estimation when applied to problems with deterministic optimal policy. Our DPG method can potentially remedy these issues. On the basis of this method and depending on the choice of a critic, different actor-critic algorithms can easily be developed in the RL setup. We present two example actor-critic algorithms. Both algorithms employ our developed policy gradient theorem for their actors, but use two different critics; one uses a simple SARSA update while the other one uses the same on-policy update but with compatible function approximators. We demonstrate the efficacy of our method both mathematically and via simulations.
更多查看译文
关键词
average reward,deterministic policy,policy gradient theorem,reinforcement learning,SMDP
AI 理解论文
溯源树
样例
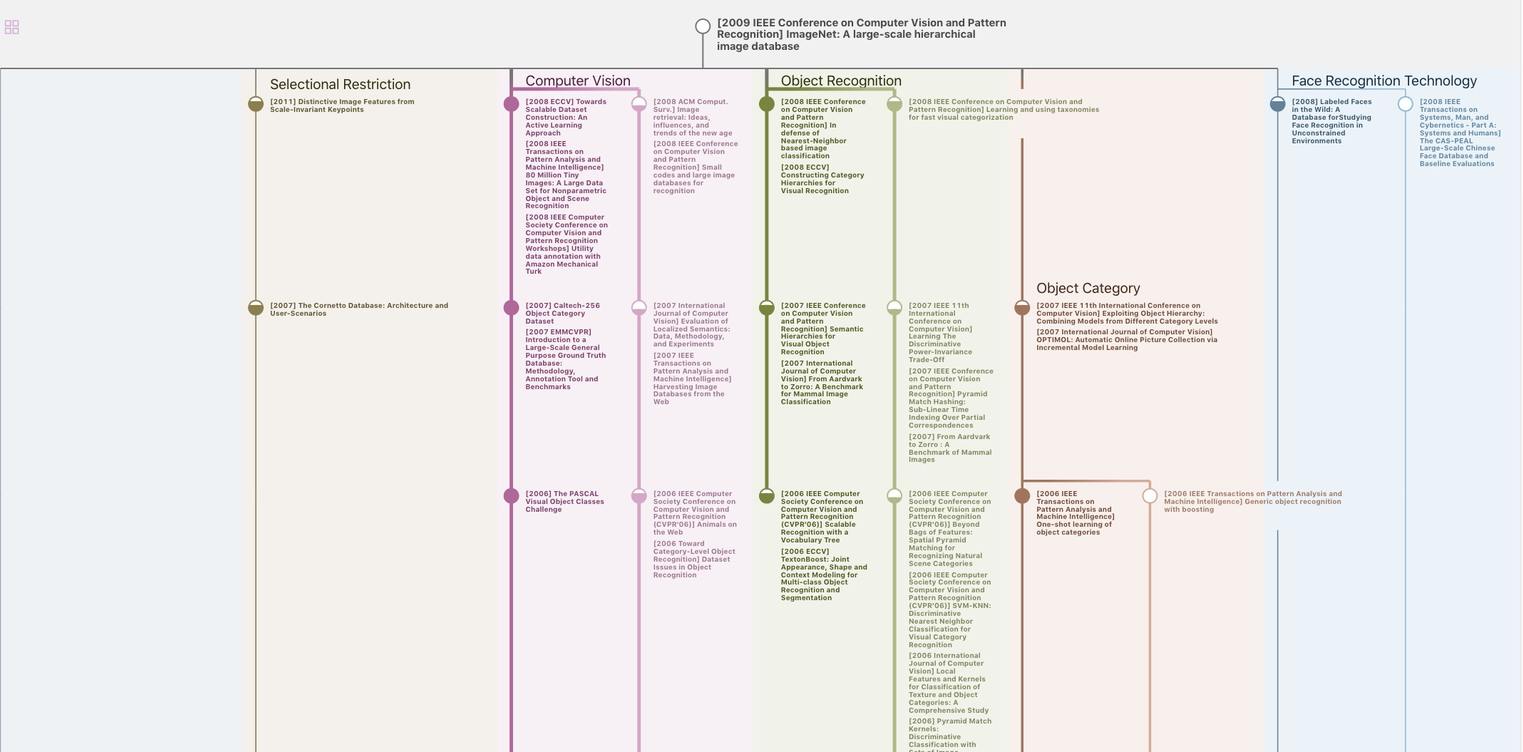
生成溯源树,研究论文发展脉络
Chat Paper
正在生成论文摘要