A Scalable Protocol Level Approach to Prevent Machine Learning Attacks on PUF-based Authentication Mechanisms for Internet-of-Medical-Things
IEEE Transactions on Industrial Informatics(2021)
摘要
The Internet-of-Things (IoT) is becoming a revolutionary paradigm, moving towards ubiquity in day to day life and used in several applications such as smart healthcare systems, industry 4.0, critical infrastructure, etc. As with any concept that relies on wireless communication, authentication is of paramount importance when it comes to security considerations. Devices in many IoT applications are severely constrained in terms of computational resources and are thus unable to utilize many modern cryptographic methods for security purposes. Physically-Unclonable-Functions (PUFs) propose to solve this issue by allowing devices to generate unique and secure digital fingerprints at extremely low computational cost. However, PUFs are vulnerable to Machine-Learning-based modeling-attacks that can mathematically clone the PUFs in order to impersonate them. To address these requirements, this paper introduces a new lightweight and practical anonymous authentication protocol for IoT that is resilient against machine learning attacks on PUFs.
更多查看译文
AI 理解论文
溯源树
样例
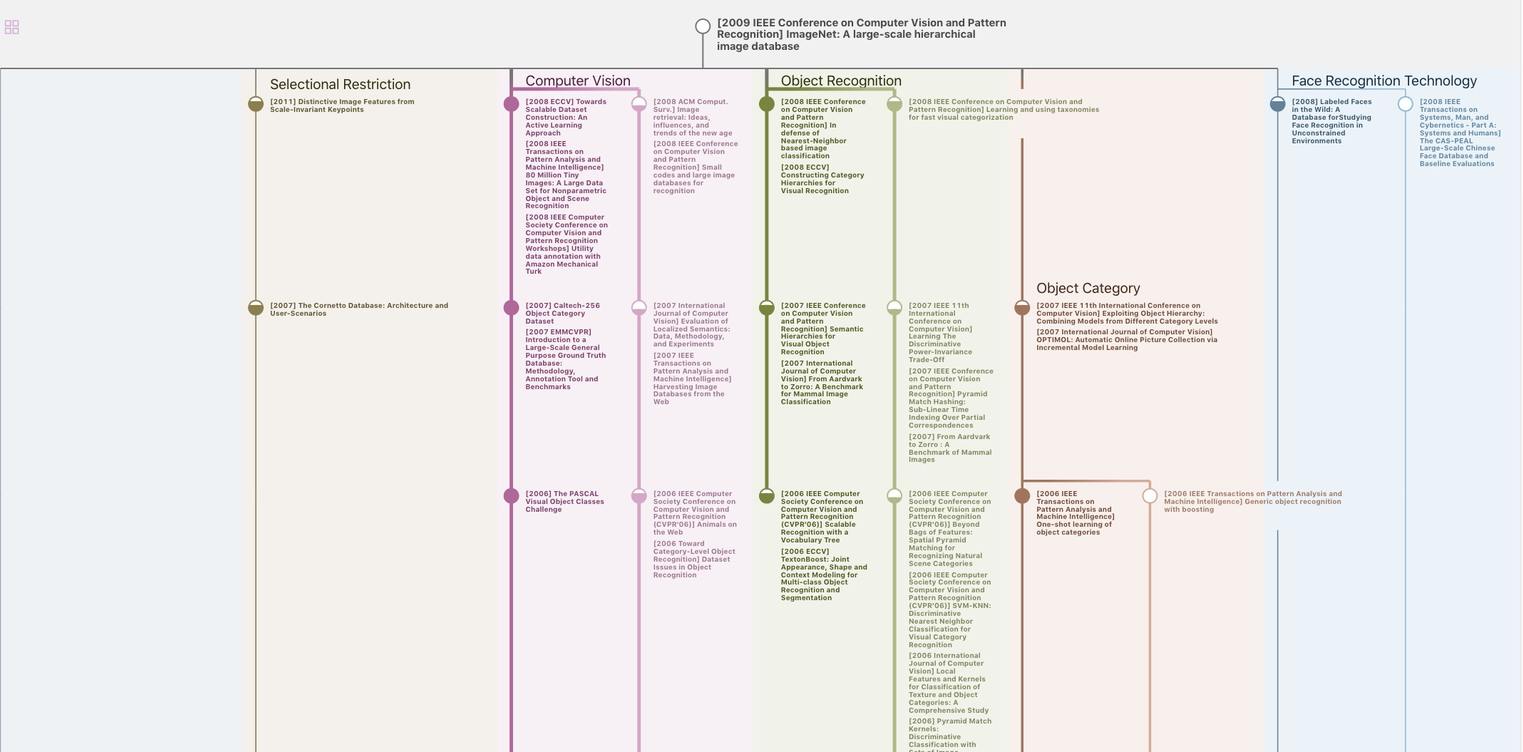
生成溯源树,研究论文发展脉络
Chat Paper
正在生成论文摘要