A Queueing-Theoretic Framework for Vehicle Dispatching in Dynamic Car-Hailing [technical report].
arXiv: Learning(2021)
摘要
With the rapid development of smart mobile devices, the car-hailing platforms (e.g., Uber or Lyft) have attracted much attention from both the academia and the industry. In this paper, we consider an important dynamic car-hailing problem, namely \textit{maximum revenue vehicle dispatching} (MRVD), in which rider requests dynamically arrive and drivers need to serve as many riders as possible such that the entire revenue of the platform is maximized. We prove that the MRVD problem is NP-hard and intractable. In addition, the dynamic car-hailing platforms have no information of the future riders, which makes the problem even harder. To handle the MRVD problem, we propose a queueing-based vehicle dispatching framework, which first uses existing machine learning algorithms to predict the future vehicle demand of each region, then estimates the idle time periods of drivers through a queueing model for each region. With the information of the predicted vehicle demands and estimated idle time periods of drivers, we propose two batch-based vehicle dispatching algorithms to efficiently assign suitable drivers to riders such that the expected overall revenue of the platform is maximized during each batch processing. Through extensive experiments, we demonstrate the efficiency and effectiveness of our proposed approaches over both real and synthetic datasets.
更多查看译文
关键词
vehicle dispatching,queueing-theoretic,car-hailing
AI 理解论文
溯源树
样例
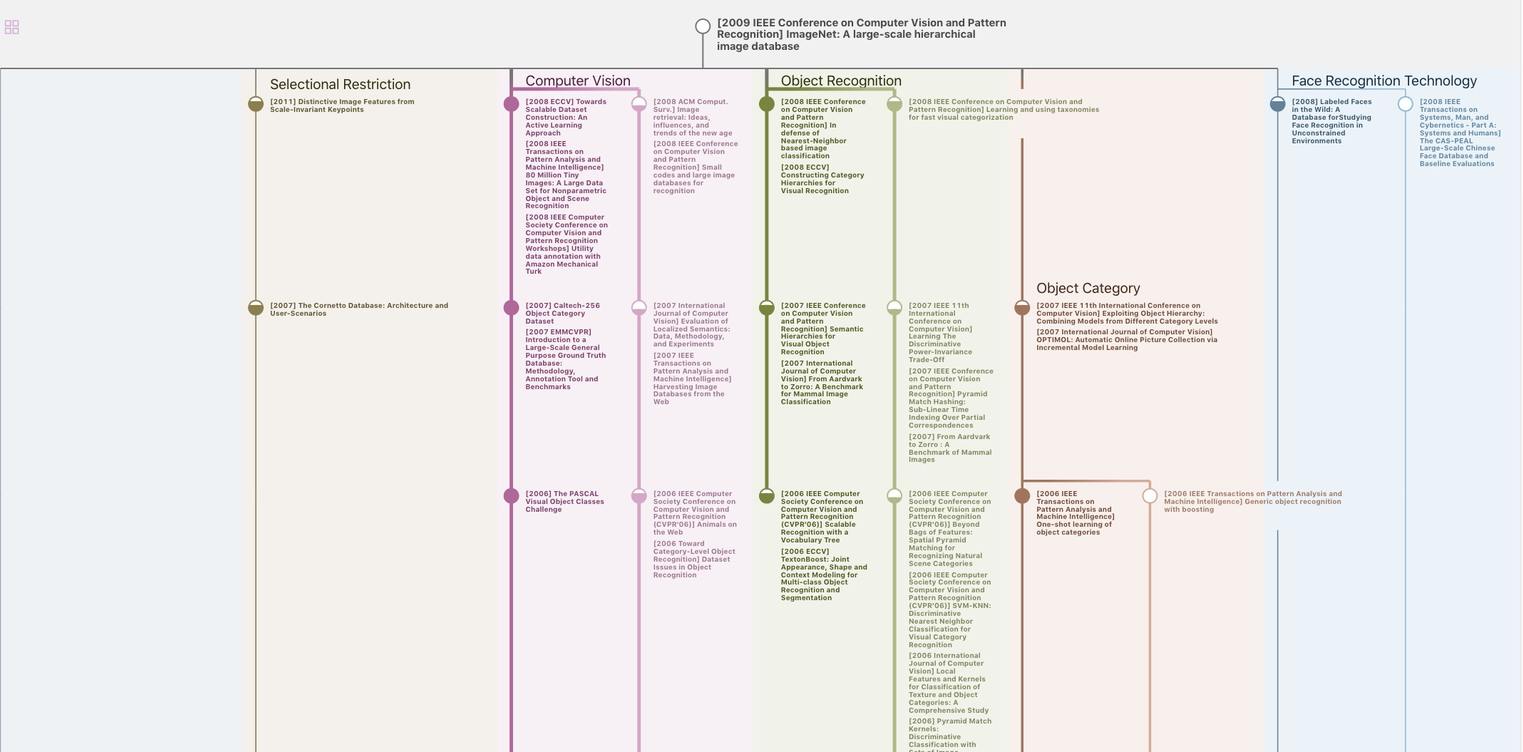
生成溯源树,研究论文发展脉络
Chat Paper
正在生成论文摘要