Dual Branch Autoencoder Network for Spectral-Spatial Hyperspectral Unmixing
IEEE GEOSCIENCE AND REMOTE SENSING LETTERS(2022)
摘要
Spatial information can play a supporting role in spectral unmixing. In this letter, we propose a dual branch autoencoder network to incorporate spatial-contextual information for spectral-spatial unmixing. The two branches leverage different architectures to efficiently extract spatial information and spectral information. In the first branch, we use fully connected layers to extract spectral information, where the neuron in each layer can capture all spectral features. In the second branch, 2-D convolution is adopted to exploit spatial features, which does not require hand-crafted assumptions compared with conventional methods. Then the extracted features are concatenated and propagated to generate the abundance and reconstruct the pixel. Moreover, to solve the drawbacks of the existing reconstruction functions, we propose a new function termed squared sine distance to improve the convergence quality of the proposed network. Experimental results reveal the effectiveness of our proposed method on both synthetic data and real-world data.
更多查看译文
关键词
Feature extraction,Image reconstruction,Convolution,Convergence,Data mining,Hyperspectral imaging,Veins,Dual branch autoencoder (DBA),hyperspectral image (HSI),spectral unmixing (SU),spectral-spatial model,squared sine distance (SSD)
AI 理解论文
溯源树
样例
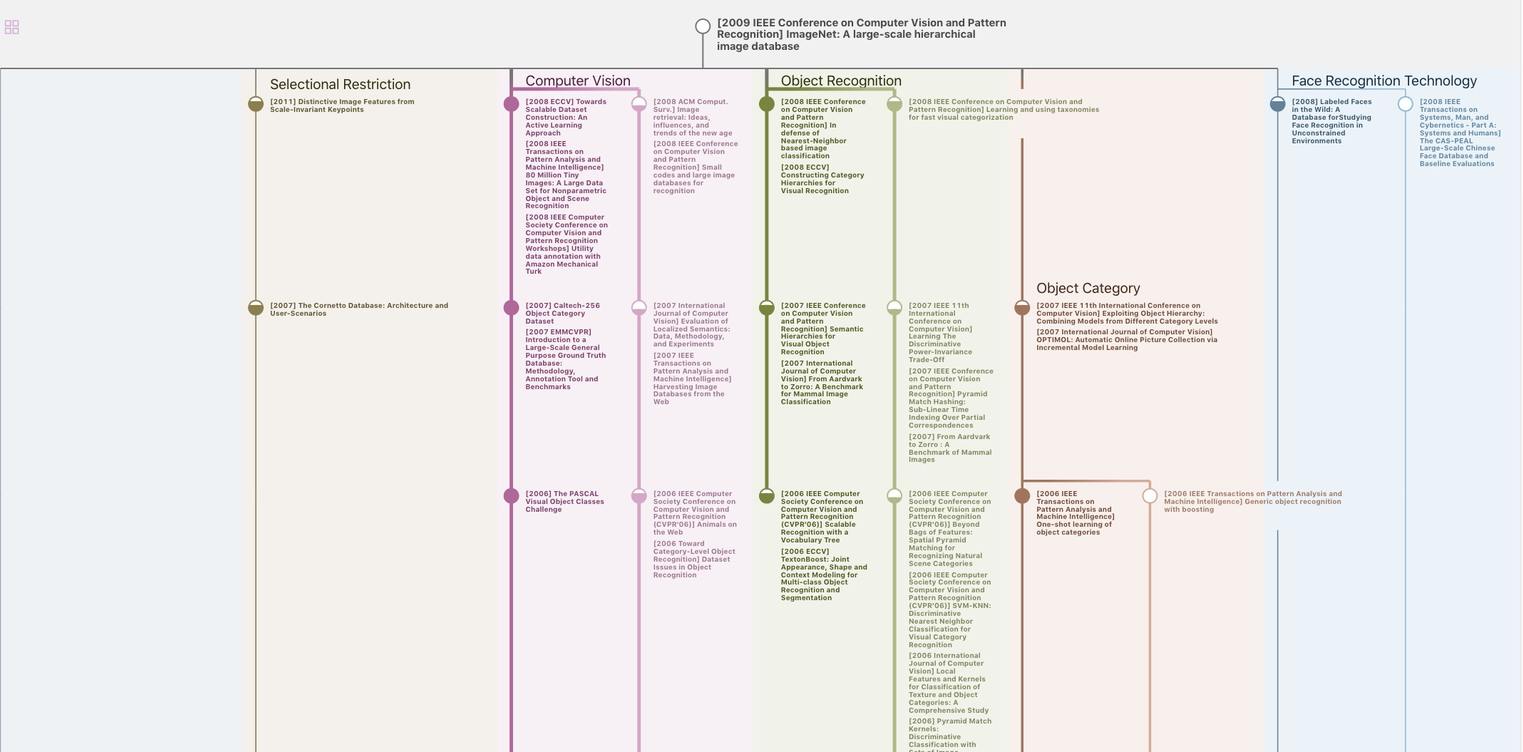
生成溯源树,研究论文发展脉络
Chat Paper
正在生成论文摘要