Methods For High Resolution Programming In Lithuim Niobate Memristors For Neuromorphic Hardware
2017 INTERNATIONAL JOINT CONFERENCE ON NEURAL NETWORKS (IJCNN)(2017)
摘要
Memristor crossbars are capable of implementing learning algorithms in a much more energy and area efficient manner compared to traditional systems. However, the programmable nature of memristor crossbars must first be explored on a smaller scale to see which memristor device structures are most suitable for applications in reconfigurable computing. In this paper, we demonstrate the programmability of memristor devices with filamentary switching based on LiNbOx, a new resistive switching oxide. We have performed several characterization experiments that demonstrate the high resolution programmability of these devices. Once the optimal programming voltages and conductivity ranges for these memristor devices are determined, a method is presented to determine how many unique states can be achieved in these devices. Parameters obtained from this analysis can greatly strengthen memristor device models that implement realistic stochastic switching behavior. We show that 11 unique states can be stored in this device with 95% confidence, but many more states are achievable using feedback write techniques or in-situ learning. Therefore, we have determined that Lithium Niobate memristors are strong candidates for use in neuromorphic computing.
更多查看译文
关键词
memristor, device, convolution, neural network, deep network
AI 理解论文
溯源树
样例
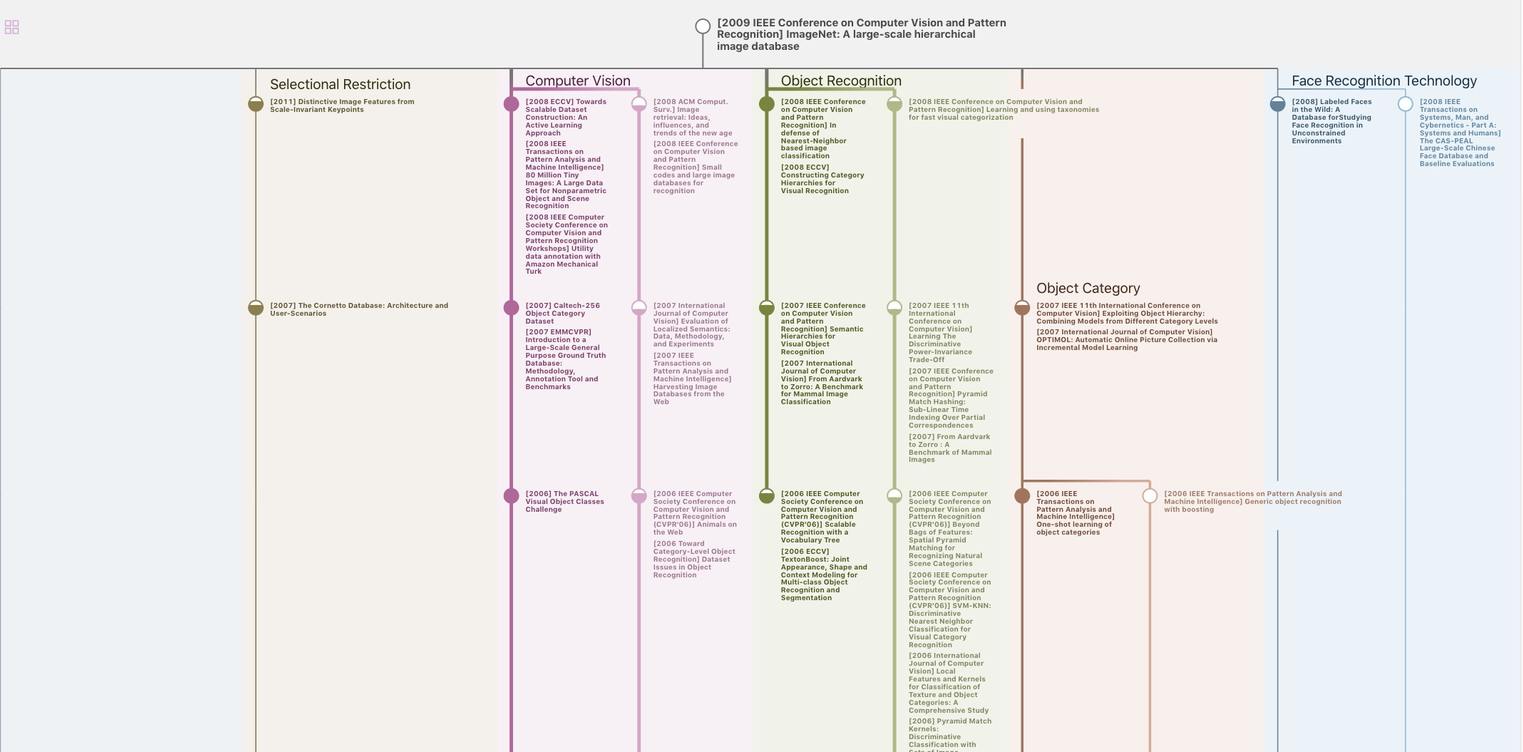
生成溯源树,研究论文发展脉络
Chat Paper
正在生成论文摘要