A Guiding Learning Model For Transformer Fault Diagnosis Combining Rule-Based Domain Knowledge And Extreme Learning Machine
2021 IEEE 4th International Electrical and Energy Conference (CIEEC)(2021)
摘要
Machine learning algorithms based on data mining have been continuously developed for half a century and have achieved good application effects in many fields including transformer fault diagnosis. However, mining rules only by data and using empirical risks to fit structural risks will have a certain negative impact on the generalization performance of the model and the security of the output results. When it is applied to transformer fault diagnosis, it is easy to diagnose serious fault as non-serious fault. In this paper, we introduce the characteristics of the domain experiential knowledge based approach and the machine learning model based approach, then a concept called guiding learning is introduced. On the basis of using Receiver operating characteristics curve (ROC) to verify and mine the relevant experience knowledge in transformer fault diagnosis field, combined with the extreme learning machine (ELM) model, a guiding learning model for transformer fault diagnosis is established. After that, the particle swarm optimization (PSO) algorithm is used to optimize the model, and in this process, the trust degree that changes with the number of iterations is introduced to better adapt to the training process of the model. By comparing with the ELM fault diagnosis model optimized by particle swarm optimization, it is proved that the established guiding learning model is superior to the traditional model in generalization performance and safety of output results. At the same time, the probability of serious diagnostic errors is dramatically reduced.
更多查看译文
关键词
transformer fault diagnosis,machine learning,domain knowledge,guiding learning,generalization performance,PSO optimization
AI 理解论文
溯源树
样例
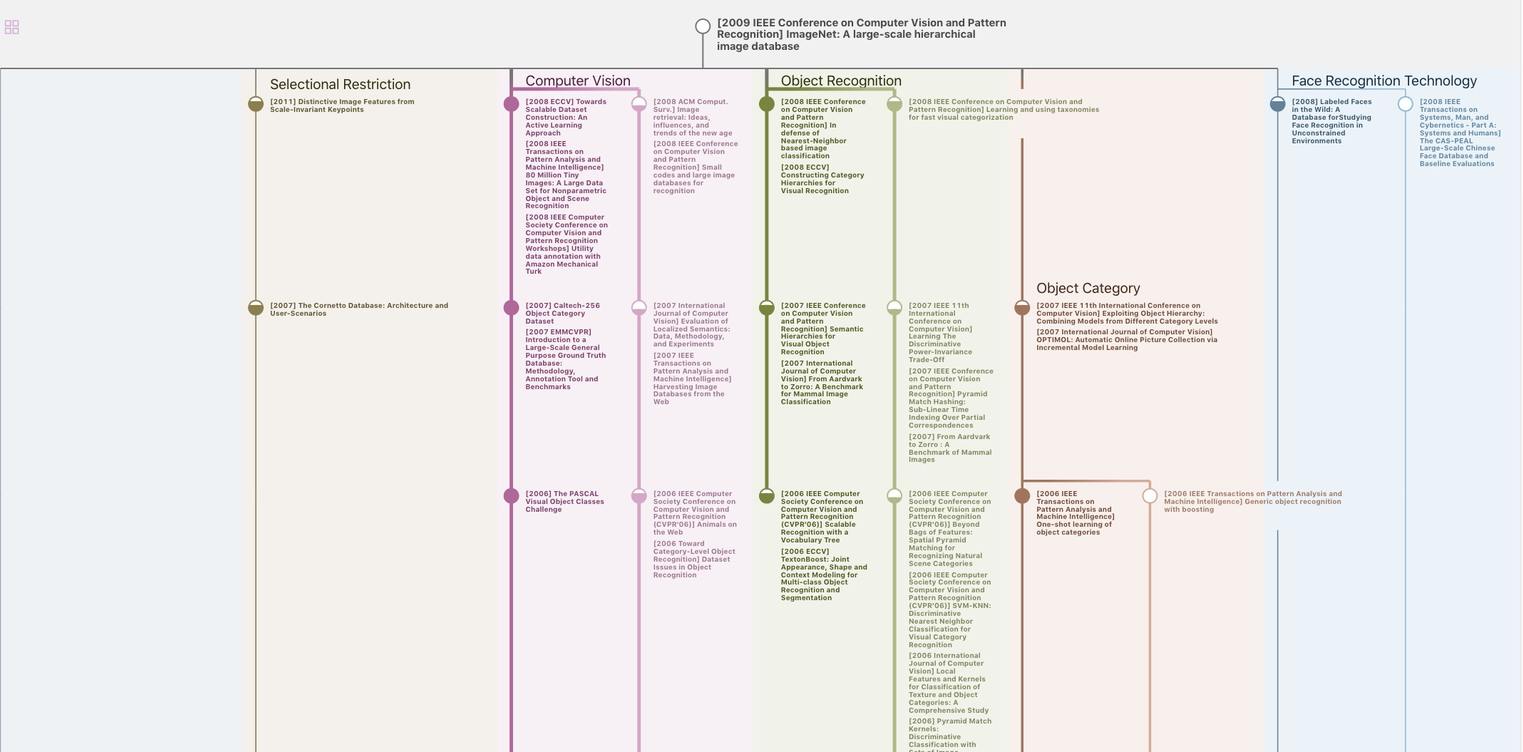
生成溯源树,研究论文发展脉络
Chat Paper
正在生成论文摘要