One-Shot Learning-Based SAR Ship Classification Using New Hybrid Siamese Network
IEEE GEOSCIENCE AND REMOTE SENSING LETTERS(2022)
摘要
Synthetic aperture radar (SAR) ship classification research mainly focuses on large ships with distinctive features. Also, ship types are expanding nowadays. Traditional standard image classification techniques are not adequate for the effective classification of SAR ships due to their fewer available data in some classes (unbalanced data). We are introducing a one-shot learning-based deep learning model built from scratch to classify 16 ship classes to solve these. It generates embeddings of size 20 and uses distance measure for classification. Also, we propose a preprocessing stage (PP-stage) and feature fusion (FF-stage) technique to improve classification accuracy. Experimental results on the OpenSARShip dataset for ship classification reveal that the accuracy is superior to the other state-of-the-art SAR image classifiers.
更多查看译文
关键词
Marine vehicles,Training,Synthetic aperture radar,Radar polarimetry,Deep learning,Network architecture,Task analysis,Deep learning,marine target classification,synthetic aperture radar (SAR),SAR ship classification
AI 理解论文
溯源树
样例
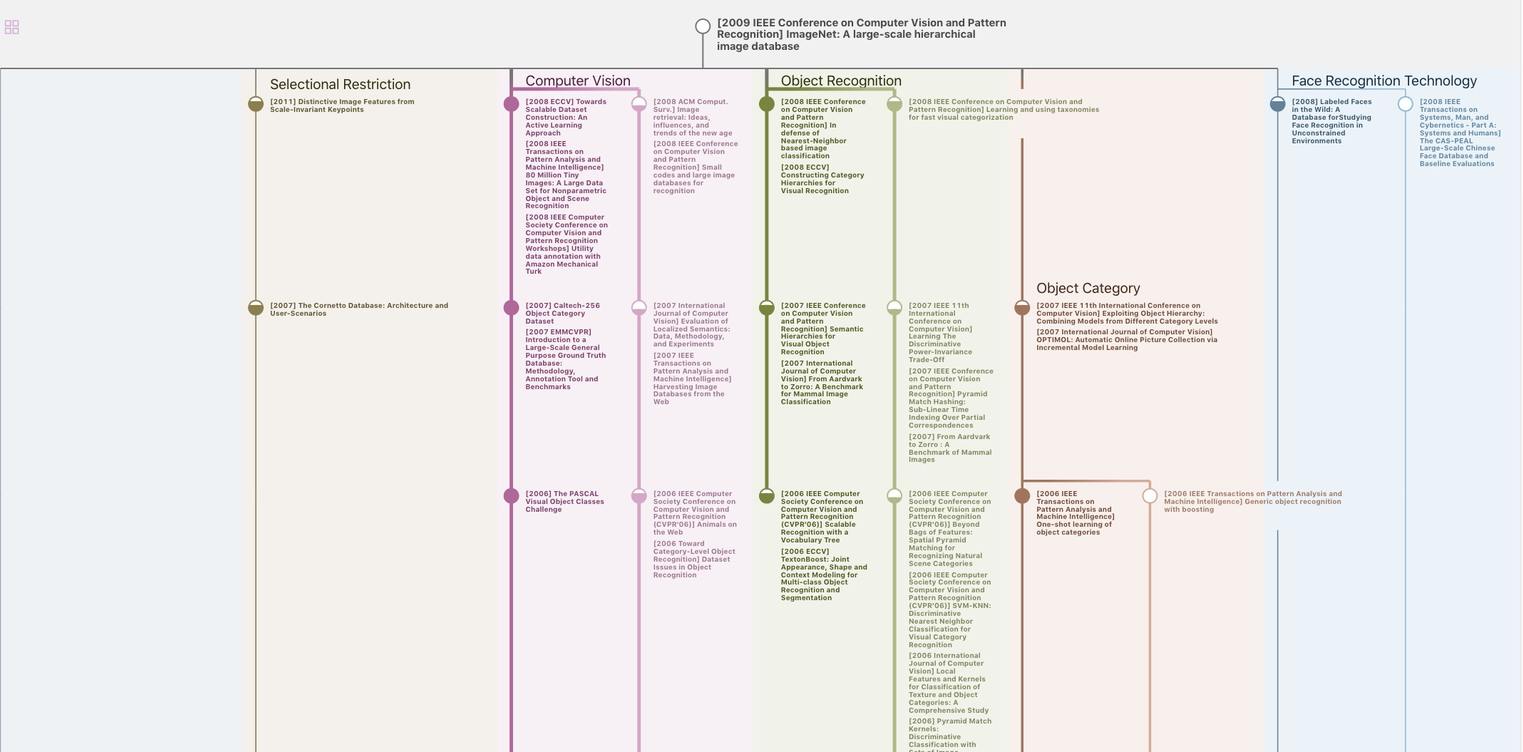
生成溯源树,研究论文发展脉络
Chat Paper
正在生成论文摘要