Predicting Transition Metal Solute Diffusion in Metals by Merging First-Principles Calculations and Machine Learning
Social Science Research Network(2019)
摘要
The transition metal (TM) solute diffusion regulates many properties and phenomena of metal alloys. Decades of research has led to lots of knowledge of interrelationships between diffusion parameters and TM solutes properties. But none has provided a universal relationship to elucidate the underlying physics of TM solute diffusion in metals. This impedes the development of machine learning model to rapidly predict solute diffusion properties in metals. Using the database of TM solute diffusion in tungsten developed here and other existing databases, we identify the role of the atomic radii of matrix metals on the law of solute diffusion, and key properties controlling the TM solute diffusion. When the atomic radii of matrix metals are larger than most TM solutes, the solute diffusion is dominated by metallic bonds between the solute and matrix atoms, which can be measured by the melting points and unpair-d-electrons of TM solutes. While for the reverse case, the solute diffusion is controlled by solute-induced lattice distortions, and the Goldschmidt radii and bulk moduli of pure TMs are measures of the TM solute diffusion in this case. Based on these findings, we predict the solute migration energies using machine learning methods with the identified key properties as descriptors. Our predicted values show 0.09 eV fitting errors and 0.15 eV cross-validation errors compared to first-principles results, indicating a satisfactory predictive capability.
更多查看译文
AI 理解论文
溯源树
样例
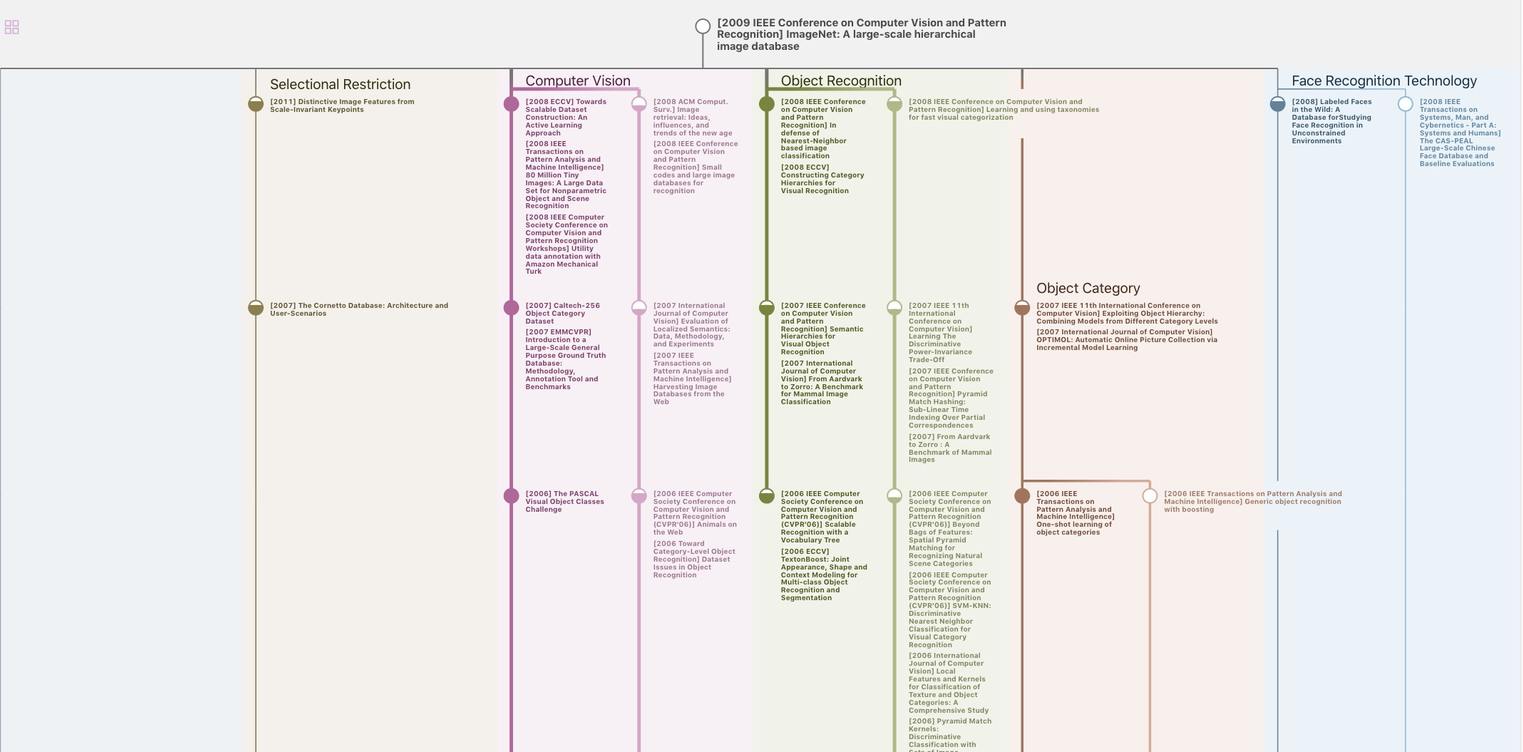
生成溯源树,研究论文发展脉络
Chat Paper
正在生成论文摘要