Forecasting Realized Volatility of Crude Oil Futures Prices based on Machine Learning
Social Science Research Network(2020)
摘要
We augment the HAR model with additional information channels to forecast realized volatility of WTI futures prices. These channels include stock markets, sentiment indices, commodity and FX markets, and text-based Google indices. We then apply four differing machine learning techniques to identify the most suitable endo- and exogenous factors which improve baseline model forecasts. We show that machine learning generated forecasts provide better forecasting quality and that portfolios which are constructed with these forecasts outperform their competing models. We find LASSO and SSVS to provide outperforming forecasts and portfolio weights. Analyzing the selection process, we show that variable choices vary across forecasting horizon. Variable selection produces clusters and provides evidence that there are structural changes with regard to the significance of information channels.
更多查看译文
AI 理解论文
溯源树
样例
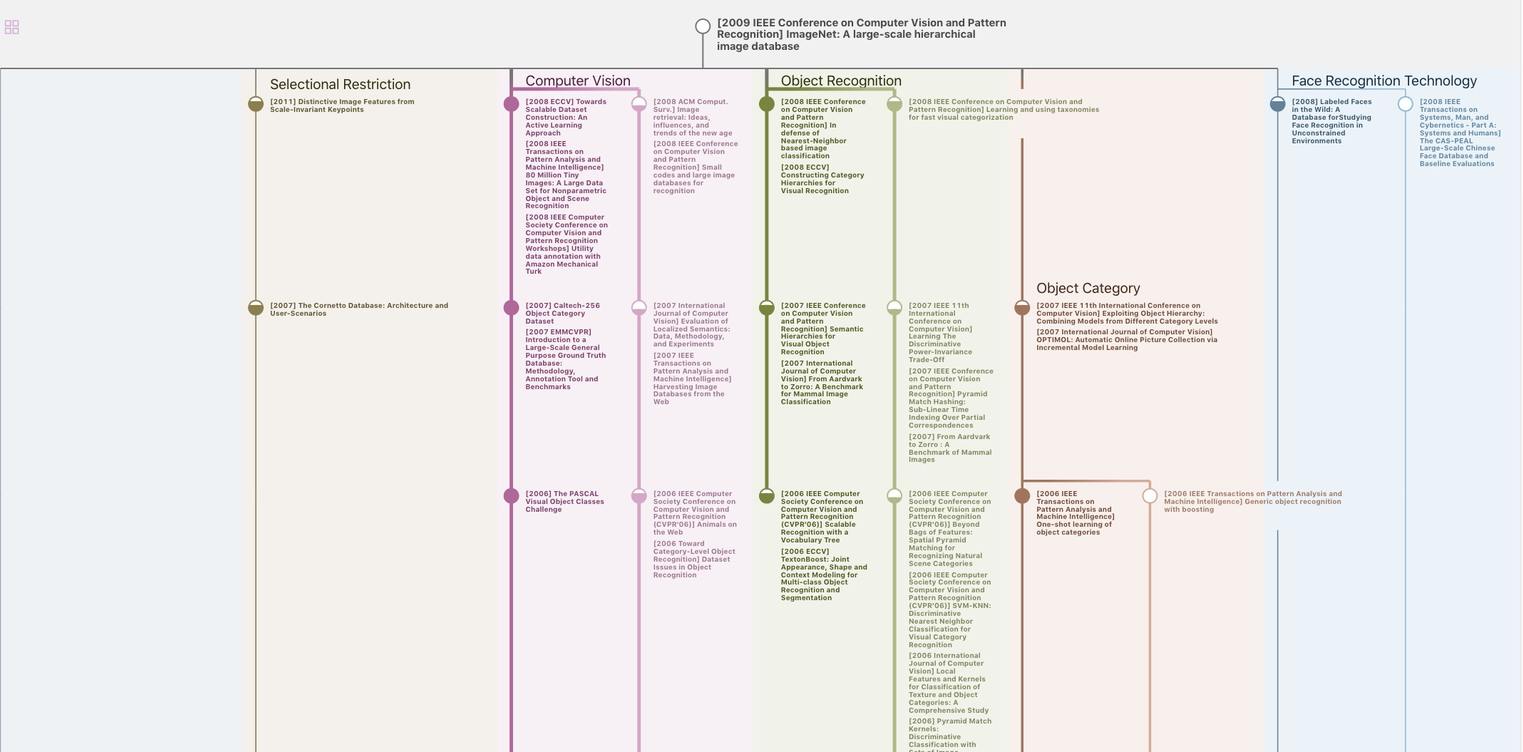
生成溯源树,研究论文发展脉络
Chat Paper
正在生成论文摘要