An Improved Convolutional Neural Network for Rolling Bearing Fault Diagnosis
ieee international conference on cyber technology in automation control and intelligent systems(2021)
摘要
Aiming at the problems of low accuracy of the existing fault diagnosis methods for rolling bearings, an improved convolution neural network model for rolling bearing fault diagnosis is proposed. By training the sub-convolution neural network model (sub-CNN) for a single fault, the sub-CNN is combined into a sub-CNN group, and the transfer learning method is used to transfer to the combiner. The combiner is composed of several fully connected layers. The proposed neural network model solves the problem that the existing end-to-end neural network model is not accurate and improves the accuracy of fault diagnosis. The experiment results show that the classification accuracy of the improved convolution neural network is 99.95%, and the F1 value is 0.9966. Compared with existing models, the highest classification accuracy of the proposed model is improved by 11.4%, which is demonstrated that the proposed model is higher than the existing models. The superiority of our model is proved.
更多查看译文
关键词
Rolling Bearing,Fault Diagnosis,Convolutional Neural Network (CNN),Transfer Learning
AI 理解论文
溯源树
样例
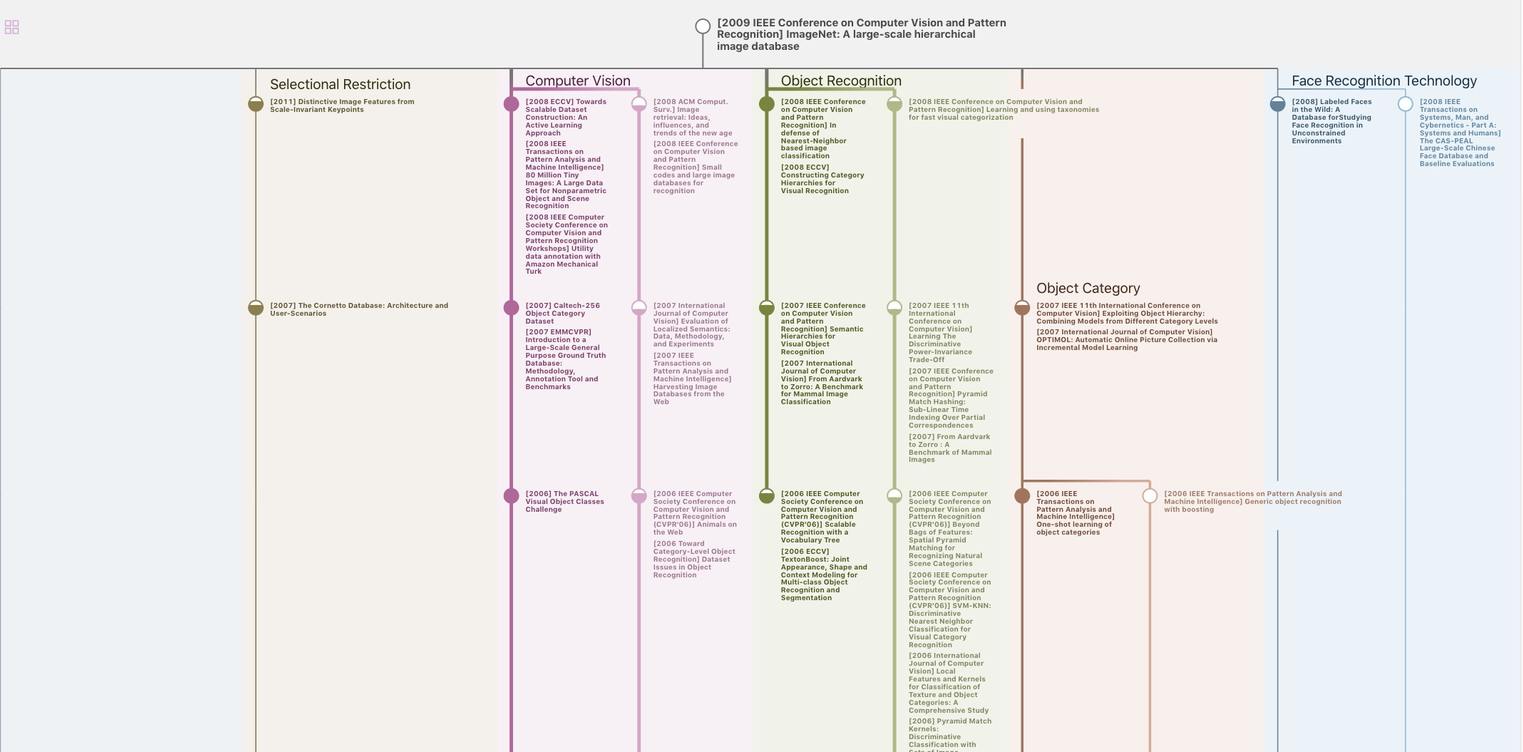
生成溯源树,研究论文发展脉络
Chat Paper
正在生成论文摘要