Deep Learning based Drone Classification using Radar Cross Section Signatures at mmWave Frequencies
IEEE Access(2021)
摘要
This paper presents drone classification at millimeter-wave (mmWave) radars using the deep learning (DL) technique. The adoption mmWave technology in radar systems enables better resolution and aid in detecting smaller drones. Using radar cross-section (RCS) signature enables us to detect malicious drones and suitable action can be taken by respective authorities. Existing drone classification converts the RCS signature into images and then performs drone classification using a convolution neural network (CNN). Converting every signature into an image induces additional computation overhead; further CNN model is trained considering fixed learning rate. Thus, when using CNN-based drone classification under a highly dynamic environment exhibit poor classification accuracy. This paper present an im- proved long short-term memory (LSTM) by introducing a weight optimization model that can reduce computation overhead by not allowing the gradient to not flow through hidden states of the LSTM model. Further, present adaptive learning rate optimizing (ALRO) model for training the LSTM model. Experiment outcome shows LSTM-ALRO achieves much better drone detection accuracies when compared with the existing CNN-based drone classification model.
更多查看译文
关键词
Drones, Adaptation models, Computational modeling, Optimization, Radar cross-sections, Mathematical models, Logic gates, Convolutional neural network, drone detection, micro doppler signature (MDS), unmanned aerial vehicle, UAV, radar cross-section, millimeter-wave
AI 理解论文
溯源树
样例
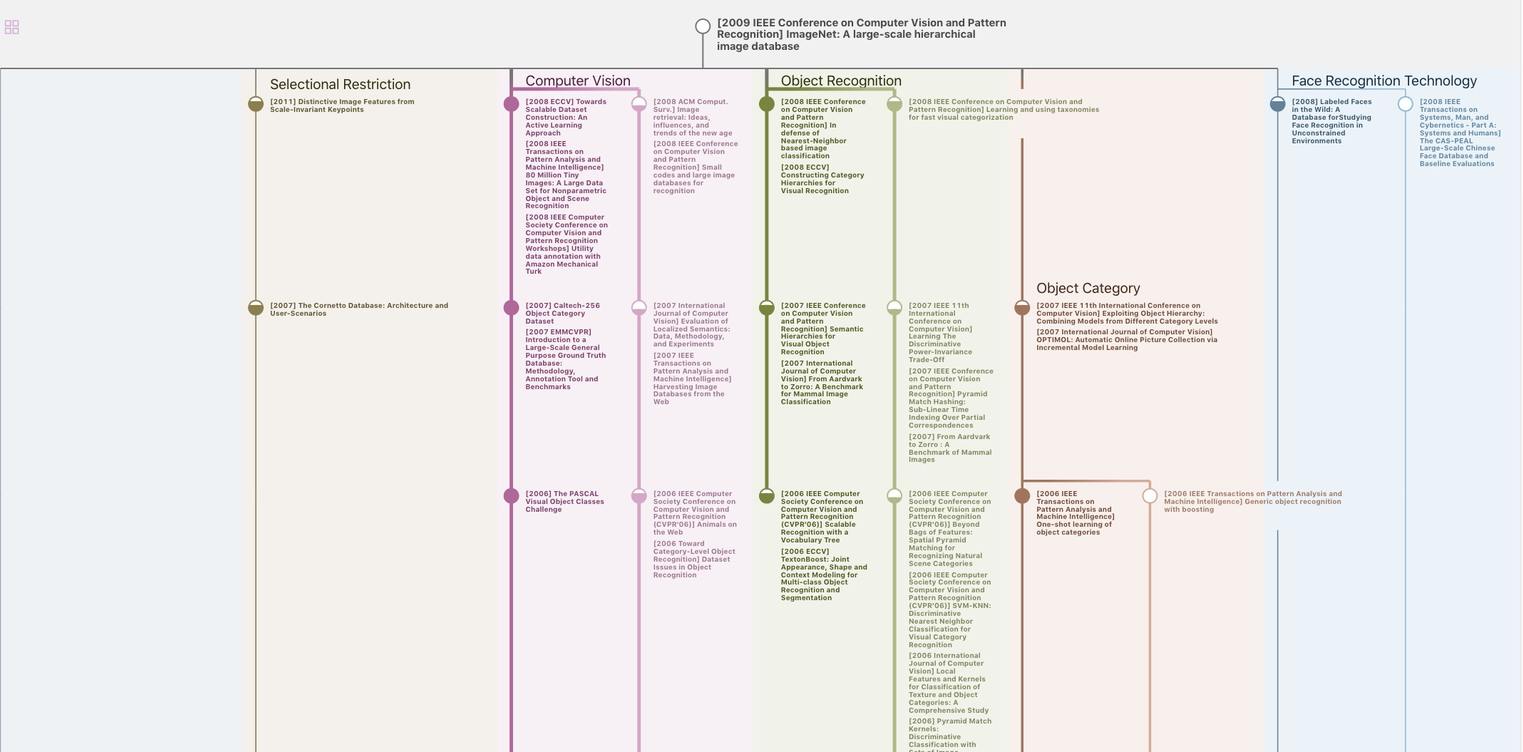
生成溯源树,研究论文发展脉络
Chat Paper
正在生成论文摘要