STPC-Net: Learn Massive Geo-Sensory Data as Spatio-Temporal Point Clouds
IEEE TRANSACTIONS ON INTELLIGENT TRANSPORTATION SYSTEMS(2022)
摘要
Nowadays, a large number of sensors are equipped on mobile or stationary platforms, which continuously generate geo-tagged and time-stamped readings (i.e., geo-sensory data) that contain rich information about the surrounding environment. These data have irregular space and time coordinates. To represent geo-sensory data, there have been extensive research efforts using time sequences, grid-like images, and graph signals. However, there still lacks a proper representation that can describe both the mobile and stationary geo-sensory data without the information-losing discretization in spatial and temporal dimensions. In this paper, we propose to represent massive geo-sensory data as spatio-temporal point clouds (STPC), and present STPC-Net, a novel deep neural network for processing STPC. STPC leverages the original irregular space-time coordinates, and STPC-Net captures intra-sensor and inter-sensor correlations from STPC. In this way, STPC-Net learns the key information of STPC, and overcomes challenges in data irregularity. Experiments using real-world datasets show that STPC-Net achieves state-of-the-art performance in different tasks on both mobile and stationary geo-sensory data. The source code is available at https://github.com/zhengchuanpan/STPC-Net.
更多查看译文
关键词
Three-dimensional displays,Sensor phenomena and characterization,Intelligent sensors,Convolution,Deep learning,Feature extraction,Task analysis,Geo-sensory data,spatio-temporal point clouds,STPC-Net,deep learning
AI 理解论文
溯源树
样例
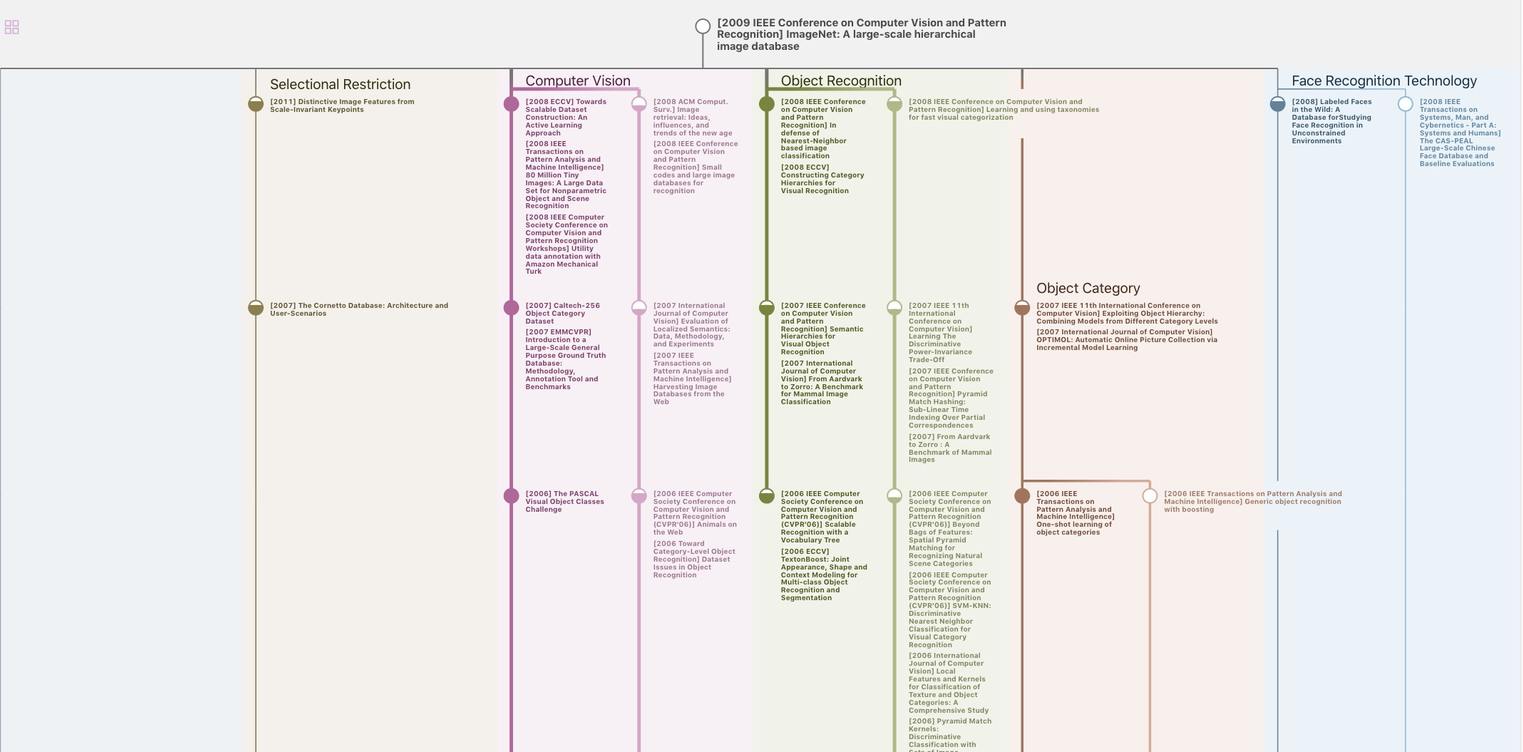
生成溯源树,研究论文发展脉络
Chat Paper
正在生成论文摘要