A CNN-RNN based approach for Simultaneous Detection, Identification and Classification of Intracranial Hemorrhage
international conference on communication information computing technology(2021)
摘要
Intracranial Hemorrhage (ICH) refers to bleeding inside the brain or skull and is considered a life-threatening condition as the accumulation of blood can lead to increased intracranial pressure, which can crush delicate brain tissues or limit its blood supply. ICH accounts for 10 to 20% of all strokes and requires immediate diagnosis and treatment. In this study, our main aim was to precisely detect and classify Intracranial Hemorrhage simultaneously. The use of Recurrent Neural Network (RNN) in analysing the sequential information obtained from slice-level classifications has been proved to be beneficial [1]. Our proposed architectures combined a Convolution Neural Network (CNN) and a Recurrent Neural Network (RNN) i.e. CNN with Long Short Term Memory (LSTM) and CNN with Gated Recurrent Unit (GRU). Using RNN would enable the previously ignored small features to be easily detected while simultaneously improving the model’s capability to distinguish between the subtypes of Intracranial Hemorrhage. Also, by performing windowing in preprocessing certain features not previously visible would become clearer. This led to the framework of three architectures: Xception, Xception-LSTM, and Xception-GRU. The dataset collected by the Radiological Society of North America is used to train these architectures (RSNA) [2]. The dataset consisted of 752,803 DICOM files out of which 10% of randomly selected samples were used for testing. The performance of these architectures is evaluated and compared using different parameters like Loss, Accuracy, AUC-ROC score, Precision, Sensitivity, Specificity, and F1 Score. We found out Xception GRU outperformed the other two architectures in almost all the parameters having achieved the loss of 0.0659. Furthermore, we have also performed a detailed analysis of per-class results using the same parameters. We believe that the use of CNN-RNN architecture increases the accuracy of ICH detection, identification and classification which would help reduce diagnosis errors
更多查看译文
关键词
Intracranial Hemorrhage,Image Processing,Convolutional Neural networks,Recurrent Neural Networks,Classification,Deep Learning,CNN-RNN
AI 理解论文
溯源树
样例
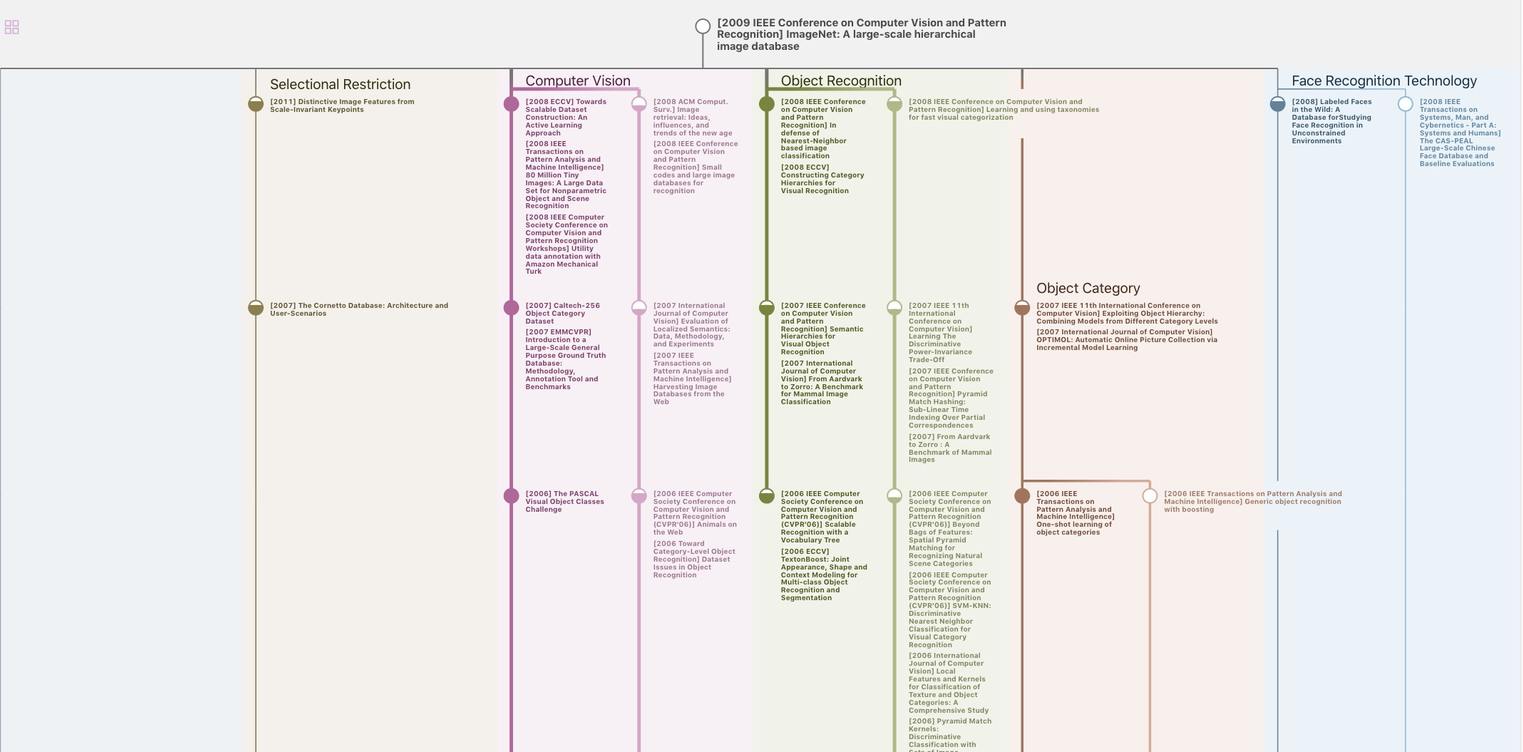
生成溯源树,研究论文发展脉络
Chat Paper
正在生成论文摘要