Multi-Level Temporal Dilated Dense Prediction for Action Recognition
IEEE TRANSACTIONS ON MULTIMEDIA(2022)
摘要
3D convolutional neural networks have achieved great success for action recognition. However, large variations of temporal dynamics have not been properly processed and low-level features have not been fully exploited in most existing works. To solve these two problems, we present a general and flexible framework, namely multi-level temporal dilated dense prediction network, which can incorporate with most of existing methods as backbone to improve the temporal modeling capacity. In the proposed method, a novel temporal dilated dense prediction block is designed to fully utilize temporal features with various temporal dilated rates for dense prediction while maintaining relatively low computational cost. To fuse information from low to high levels, our method combines the predictions from multiple such blocks inserted at different stages of the backbone network. In-depth analysis is given to show that short- to long-term temporal dependencies can be captured and multi-level spatio-temporal features are effectively fused for video action recognition by the proposed method. Experimental results demonstrate that our method achieves impressive performance improvement on four publicly available action recognition benchmarks including Charades, Kinetics, Something-Something-V1 and HMDB51.
更多查看译文
关键词
Feature extraction,Three-dimensional displays,Convolution,Image recognition,Task analysis,Solid modeling,Predictive models,Action Recognition,Temporal Dilated Dense Prediction,Multi-level Fusion,3D Convolutional Neural Network
AI 理解论文
溯源树
样例
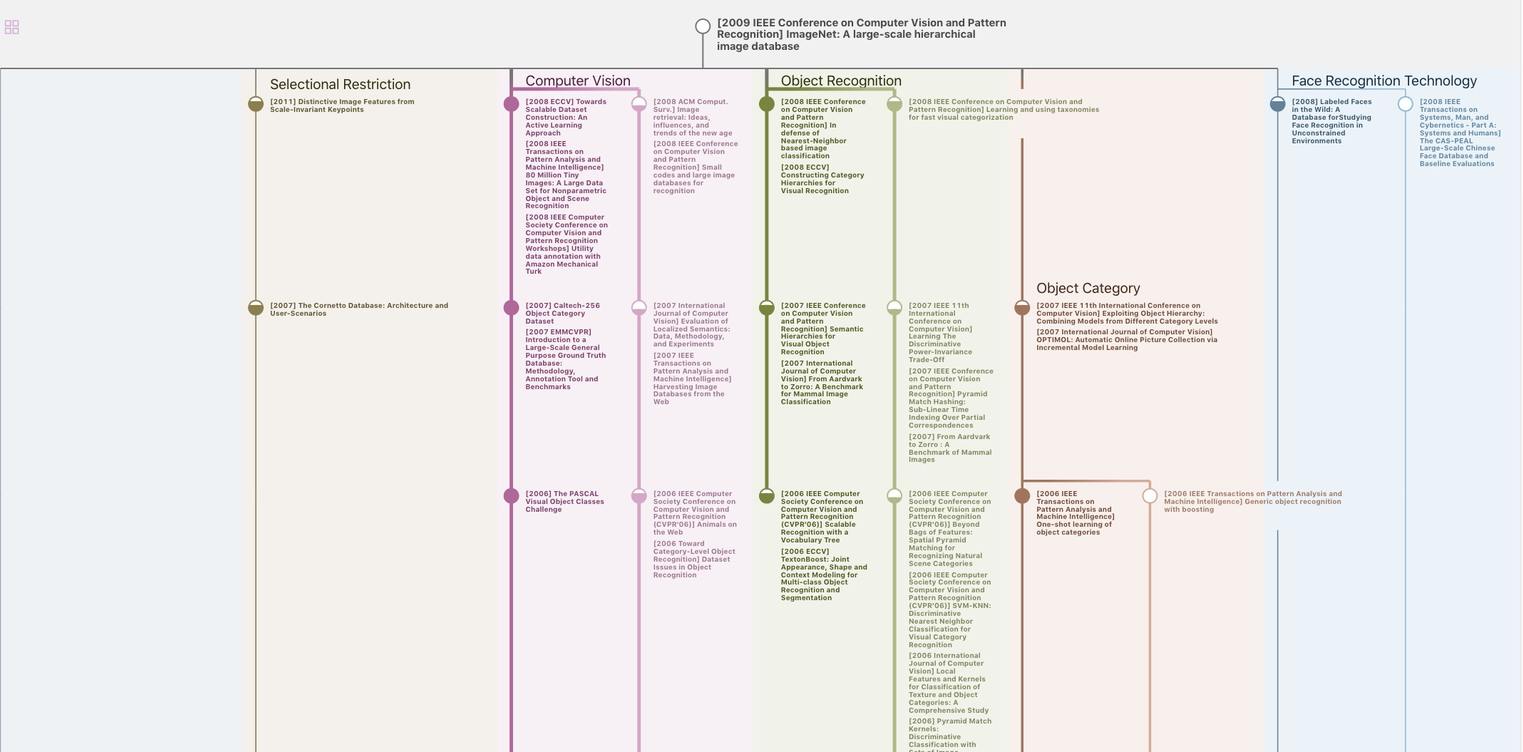
生成溯源树,研究论文发展脉络
Chat Paper
正在生成论文摘要