Manifold Learning and Clustering for Automated Phase Identification and Alignment in Data Driven Modeling of Batch Processes
Frontiers in chemical engineering(2020)
摘要
Processing data that originates from uneven, multi-phase batches is a challenge in data-driven modeling. Training predictive and monitoring models requires the data to be in the right shape to be informative. Only then can a model learn meaningful features that contain deterministic variability of the process. The presence of multiple phases in the data, which display different correlation patterns and have an uneven duration from batch to batch, reduces the performance of the data-driven modeling methods significantly. Therefore, phase identification and alignment is a critical step and can lead to an unsuccessful modeling exercise if not applied correctly. In this paper, a novel approach is proposed to perform unsupervised phase identification and alignment based on the correlation patterns found in the data. Phase identification is performed via manifold learning using t-Distributed Stochastic Neighbor Embedding (t-SNE), which is a state-of-the-art machine learning algorithm for non-linear dimensionality reduction. The application of t-SNE to a reduced cross-correlation matrix of every batch with respect to a reference batch results in data clustering in the embedded space. Models based on support vector machines (SVMs) are trained to, (i) reproduce the manifold learning obtained via t-SNE, and (ii) determine the membership of the data points to a process phase. Compared to previously proposed clustering approaches for phase identification, this is an unsupervised, non-linear method. The perplexity parameter of the t-SNE algorithm can be interpreted as the estimated duration of the shortest phase in the process. The advantages of the proposed method are demonstrated through its application on an \textit{in-silico} benchmark case study, and on real industrial data from two unit-operations in the large scale production of an active pharmaceutical ingredients (API). The efficacy and robustness of the method are evidenced in the successful phase identification and alignment obtained for these three distinct processes, displaying smooth, sudden and repetitive phase changes. Additionally, the low complexity of the method makes feasible its online implementation.
更多查看译文
关键词
manifold learning, clustering, t-distributed stochastic neighbor embedding, support vector machines, phase identification and alignment, batch processes, active pharmaceutical ingredients
AI 理解论文
溯源树
样例
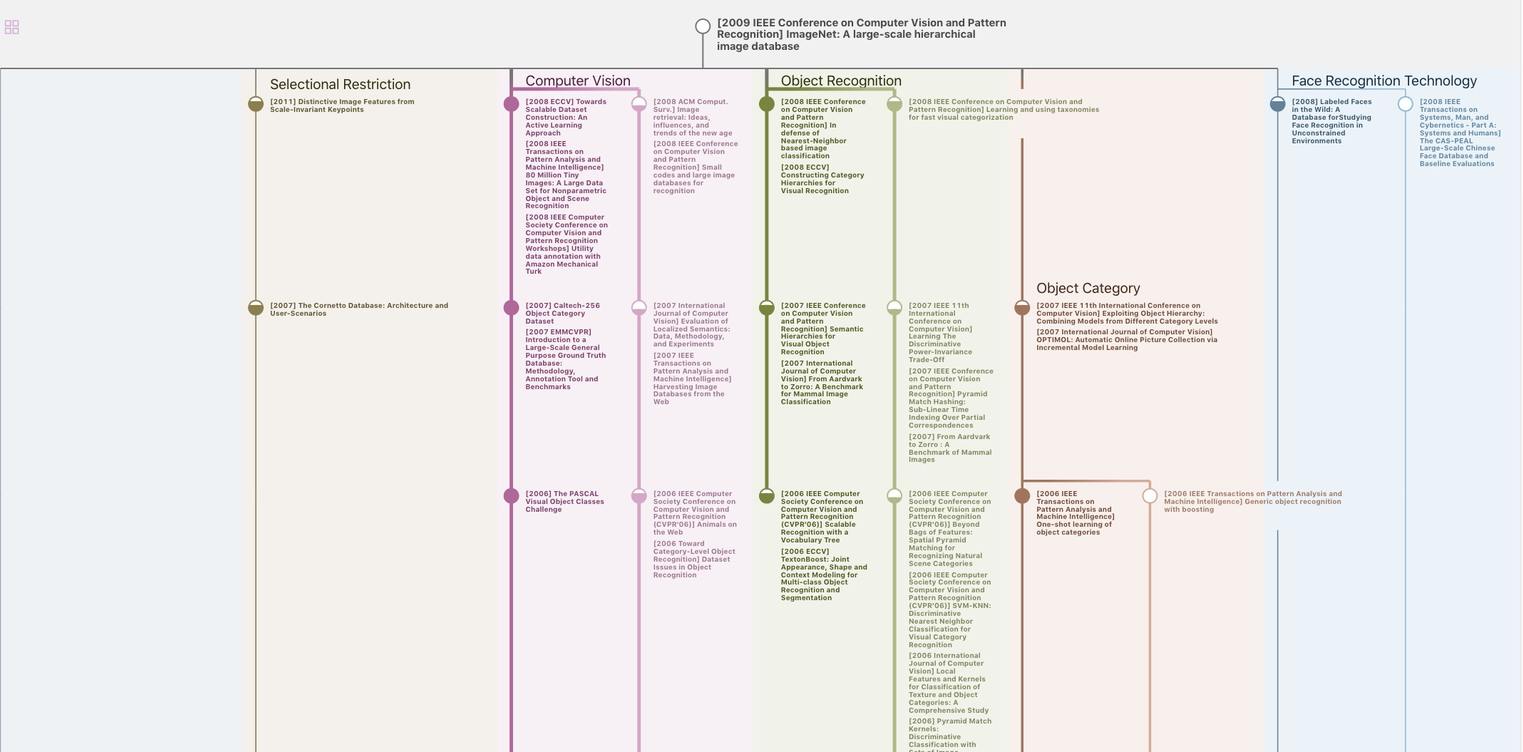
生成溯源树,研究论文发展脉络
Chat Paper
正在生成论文摘要