A Systematic Review of Prediction Models for Tuberculosis Treatment Outcomes
Social Science Research Network(2020)
摘要
Background: Tuberculosis (TB) outcome prediction models are important for informing clinical practice and TB management policies, but existing models have not been systematically reviewed.
Design/Methods: PubMed, Embase, Web of Science, and Google Scholar were searched for studies published January 1, 1995 - January 9, 2020. Studies that developed a model to predict pulmonary TB treatment outcomes were included. Study screening, data extraction, and quality assessment were conducted independently by two reviewers. Study quality was evaluated using the Prediction model Risk Of Bias Assessment Tool (PROBAST). The study was pre-registered on OSF (https://osf.io/rz3wp).
Findings: 14,739 articles were identified, 536 underwent full-text review, and 33 studies presenting 37 prediction models were included. Model outcomes included death (n=16, 43%), treatment failure (n=6, 16%), default (n=6, 16%) or a composite outcome (n=9, 25%). Most models (n=29, 78%) measured discrimination (median c-statistic=0.75; IQR: 0.68-0.84), and 17 (46%) reported calibration, often the Hosmer-Lemeshow test (n=13). Nineteen (51%) models were internally validated, and six (16%) were externally validated. Eighteen studies (54%) mentioned missing data, and of those half (n=9) used complete case analysis. The most common predictors included age, sex, extrapulmonary TB, body mass index (BMI), chest x-ray results, previous TB, and HIV. Risk of bias varied across studies, but all studies had high risk of bias in their analysis.
Interpretation: TB outcome prediction models are heterogeneous with disparate outcome definitions, predictors, and methodology. We do not recommend applying any in clinical settings without external validation, and encourage future researchers adhere to guidelines for developing and reporting of prediction models.
Funding Statement: This work was supported by the National Center for Advancing Translational Sciences [CTSA Award No. TL1TR000447 to L.S.P.].
Declaration of Interests: None declared.
更多查看译文
AI 理解论文
溯源树
样例
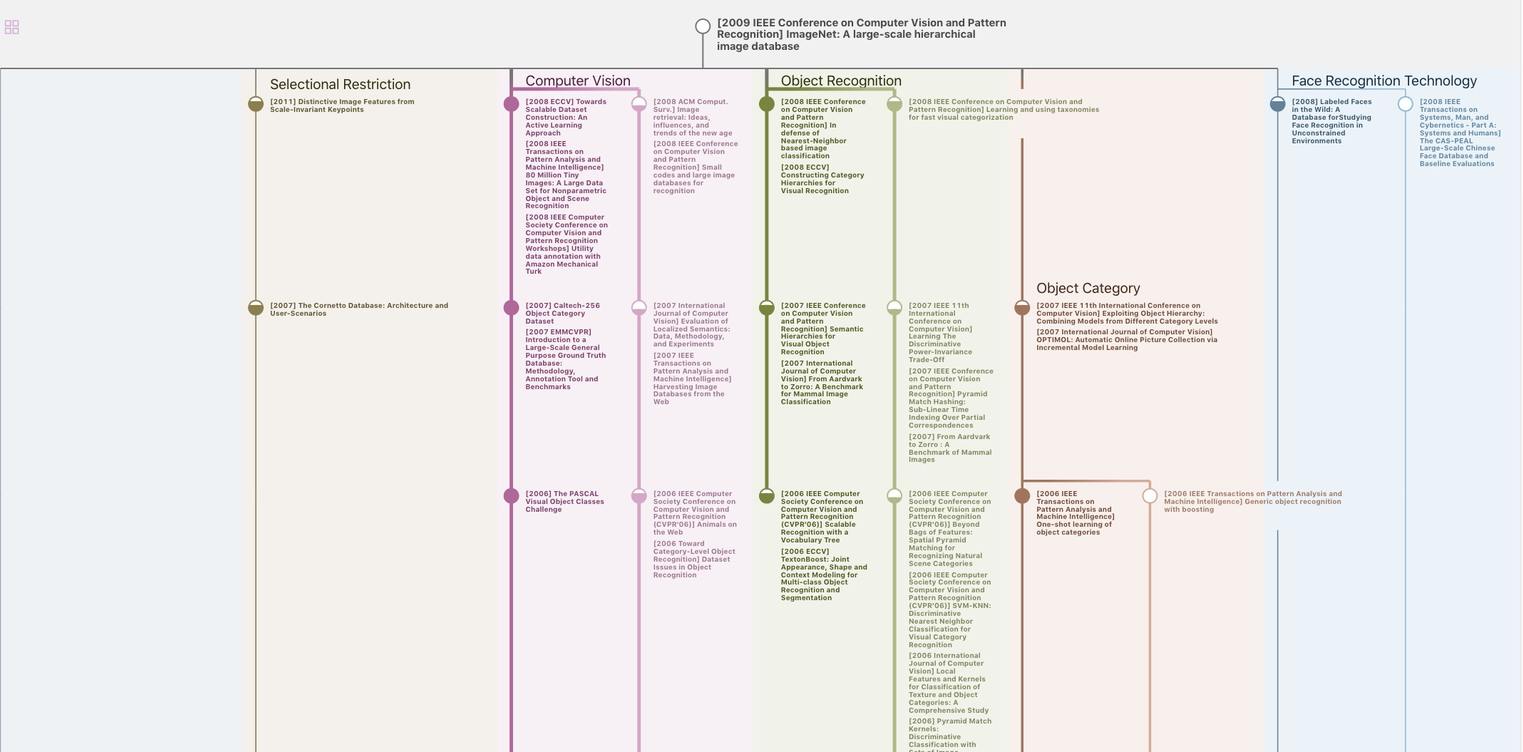
生成溯源树,研究论文发展脉络
Chat Paper
正在生成论文摘要