Computational Framework for Machine-Learning-Enabled 13C Fluxomics.
ACS Synthetic Biology(2021)
摘要
13C metabolic flux analysis (MFA) has emerged as a powerful tool for synthetic biology. This optimization-based approach suffers long computation time and unstable solutions depending on the initial guess. Here, we develop a machine-learning-based framework for 13C fluxomics. Specifically, training and test data sets are generated by metabolic network decomposition and flux sampling, in which flux ratios at metabolic nodes and simulated labeling patterns of metabolites are used as training targets and features, respectively. To improve prediction accuracy and simplify the model, automated processes are developed for flux ratio selection based on solvability and feature screening based on importance. We found that predictive performance can be significantly improved using both amino acids and central carbon metabolites in comparison with amino acids alone. Together with measured external fluxes, the predicted flux ratios determine the mass balance system, yielding global flux distributions. This approach is validated by flux estimation using both simulated and experimental data in comparison with canonical 13C MFA. The approach represents a reliable fluxomics method readily applicable to high-throughput metabolic phenotyping, which highlights the advances of intelligent learning algorithms in synthetic biology, specifically in the Test and Learn stage of the Design-Build-Test-Learn cycle.
更多查看译文
关键词
machine-learning-enabled machine-learning-enabled,<sup>13</sup>c
AI 理解论文
溯源树
样例
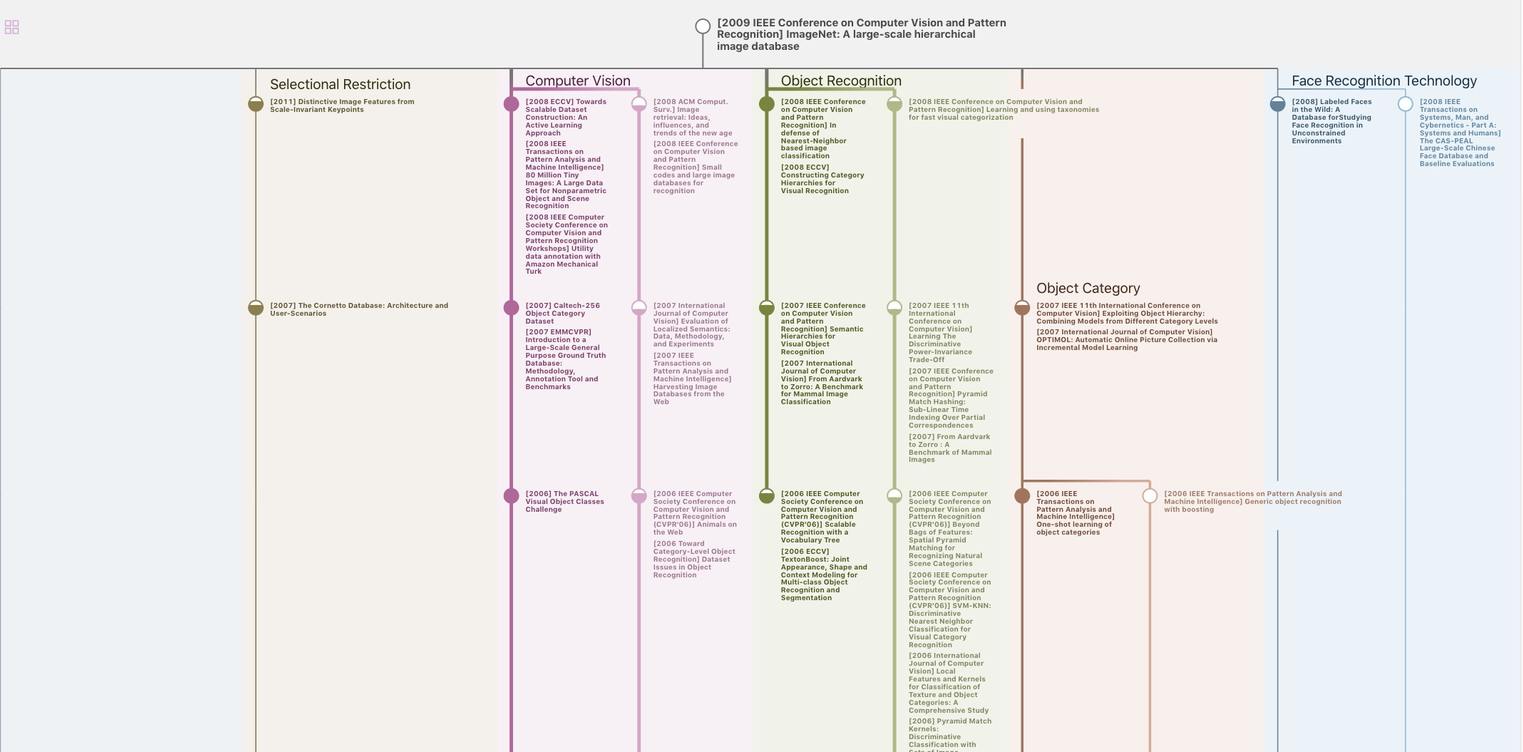
生成溯源树,研究论文发展脉络
Chat Paper
正在生成论文摘要