Synthetic Aperture Radar Image Compression Based on a Variational Autoencoder
IEEE geoscience and remote sensing letters(2022)
摘要
Given the uniqueness of synthetic aperture radar (SAR) images, traditional optical image compression algorithms cannot fully exploit their redundant information. To improve SAR image compression in terms of rate–distortion performance and visual perception, an end-to-end SAR image compression convolutional neural network (CNN) model based on a variational autoencoder is proposed. The proposed CNN model consists of a main autoencoder and a hyper autoencoder. To reduce dependencies in latent space, a joint transform of linear CNN and nonlinear generalized divisive normalization (GDN) activation is applied in the main autoencoder. Moreover, residual blocks are combined with the transforms to boost the efficiency of feature learning and make use of subpixels to improve the quality of reconstructed images. Instead of a fixed entropy model, a conditioned entropy model that works with a hyperprior network is used to learn the distribution of latents, which helps to further improve the compression quality. During training, the model is optimized by evaluating the rate–distortion performance. The experimental results show that the proposed method can achieve better distortion performance than JPEG, JPEG2000, and the available CNN-based method in terms of objective evaluation criteria and human vision perception quality.
更多查看译文
关键词
Image coding,Entropy,Radar polarimetry,Decoding,Synthetic aperture radar,Transform coding,Training,Autoencoder,convolutional neural network (CNN),image compression,subpixel,synthetic aperture radar (SAR)
AI 理解论文
溯源树
样例
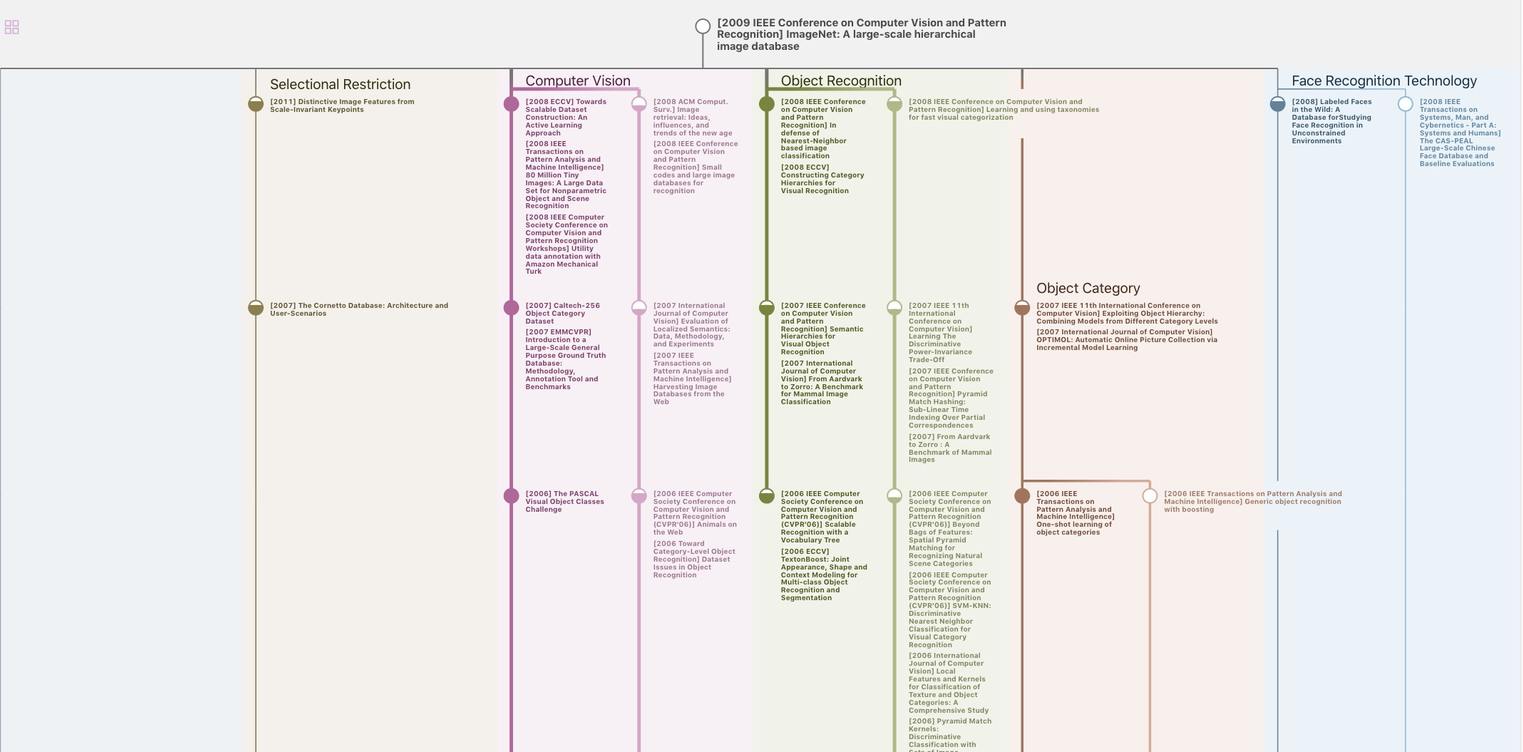
生成溯源树,研究论文发展脉络
Chat Paper
正在生成论文摘要