MEDNet: Multiexpert Detection Network With Unsupervised Clustering of Training Samples
IEEE TRANSACTIONS ON GEOSCIENCE AND REMOTE SENSING(2022)
摘要
For various remote sensing objects, the current detection framework based on a single detection pipeline fails to provide satisfactory detection accuracy. In order to further improve the object recognition ability of the detection model, this article introduces the effective ``multiexpert'' mechanism into the field of remote sensing object detection and then constructs a multiexpert detection network (MEDNet). In this model, we first construct multiple feature pyramids (MFPs) to replace the traditional single feature pyramid to enrich the semantic representation ability of the model. Then, we equip multiple detection experts (MDEs) to leverage multiple kinds of features from MFP to perform different semantic predictions. As the first CNN-based multiexpert detection model for remote sensing images, we tailor a loss distance-based k-experts clustering (LD-kEC) strategy to assign training samples to different detection experts in an unsupervised fashion. By this strategy, we can directly use the existing remote sensing dataset without expert labels for end-to-end training of our multiexpert model. The experimental results prove that the proposed multiexpert-based detector can indeed significantly improve the object detection performance for remote sensing images.
更多查看译文
关键词
Feature extraction,Remote sensing,Object detection,Training,Predictive models,Pipelines,Geospatial analysis,Loss distance-based k-experts clustering (LD-kEC),multiexpert detection network (MEDNet),multiple feature pyramids (MFPs),object detection,remote sensing images
AI 理解论文
溯源树
样例
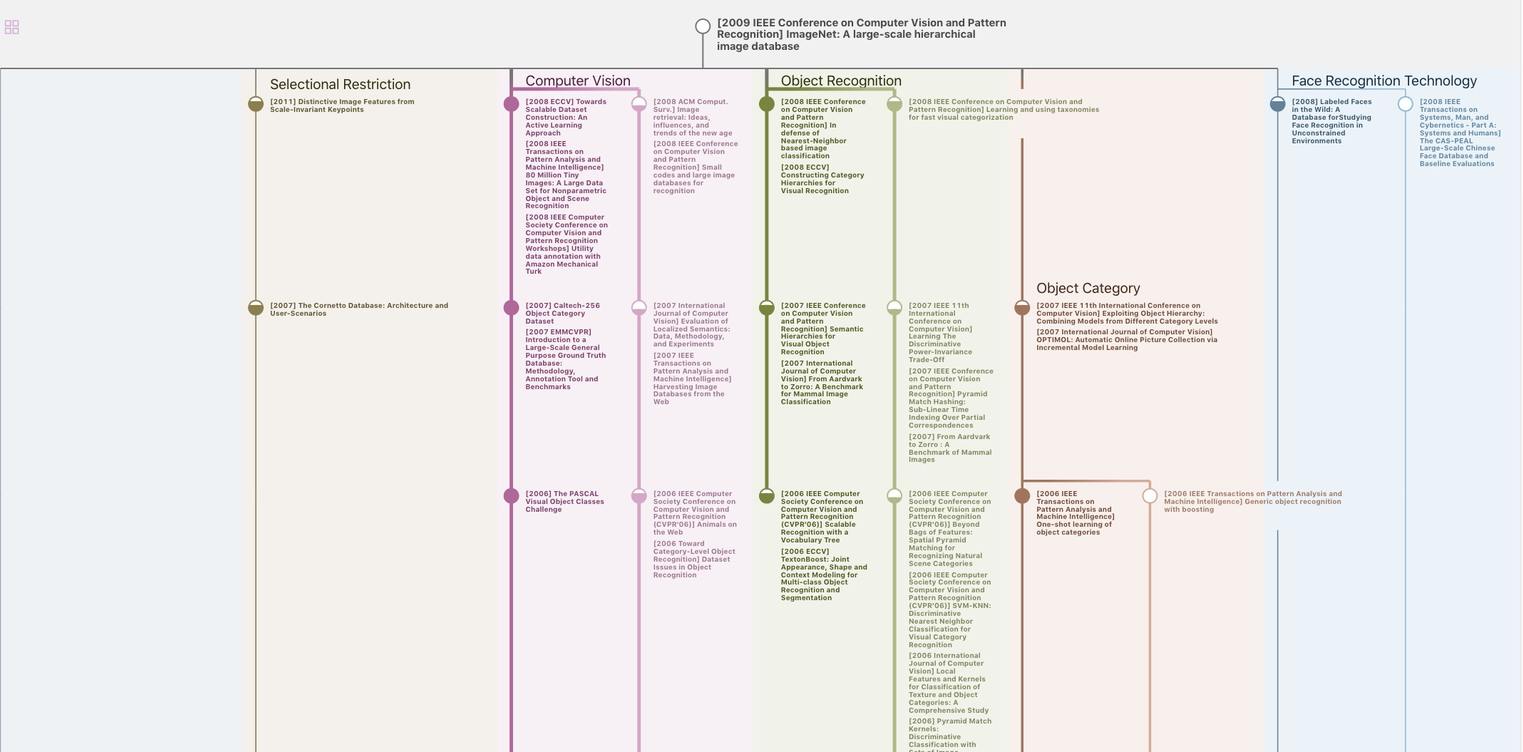
生成溯源树,研究论文发展脉络
Chat Paper
正在生成论文摘要