Prediction and Analysis of Geomechanical Properties of Jimusaer Shale Using a Machine Learning Approach
SPWLA 62nd Annual Online Symposium Transactions(2021)
摘要
Geomechanical properties are essential for safe drilling, successful completion, and exploration of both conven-tional and unconventional reservoirs, e.g. deep shale gas and shale oil. Typically, these properties could be calcu-lated from sonic logs. However, in shale reservoirs, it is time-consuming and challenging to obtain reliable log-ging data due to borehole complexity and lacking of in-formation, which often results in log deficiency and high recovery cost of incomplete datasets. In this work, we propose the bidirectional long short-term memory (BiL-STM) which is a supervised neural network algorithm that has been widely used in sequential data-based pre-diction to estimate geomechanical parameters. The pre-diction from log data can be conducted from two differ-ent aspects. 1) Single-Well prediction, the log data from a single well is divided into training data and testing data for cross validation; 2) Cross-Well prediction, a group of wells from the same geographical region are divided into training set and testing set for cross validation, as well. The logs used in this work were collected from 11 wells from Jimusaer Shale, which includes gamma ray, bulk density, resistivity, and etc. We employed 5 vari-ous machine learning algorithms for comparison, among which BiLSTM showed the best performance with an R-squared of more than 90% and an RMSE of less than 10. The predicted results can be directly used to calcu-late geomechanical properties, of which accuracy is also improved in contrast to conventional methods.
更多查看译文
关键词
jimusaer shale,geomechanical properties,machine learning approach,machine learning,prediction
AI 理解论文
溯源树
样例
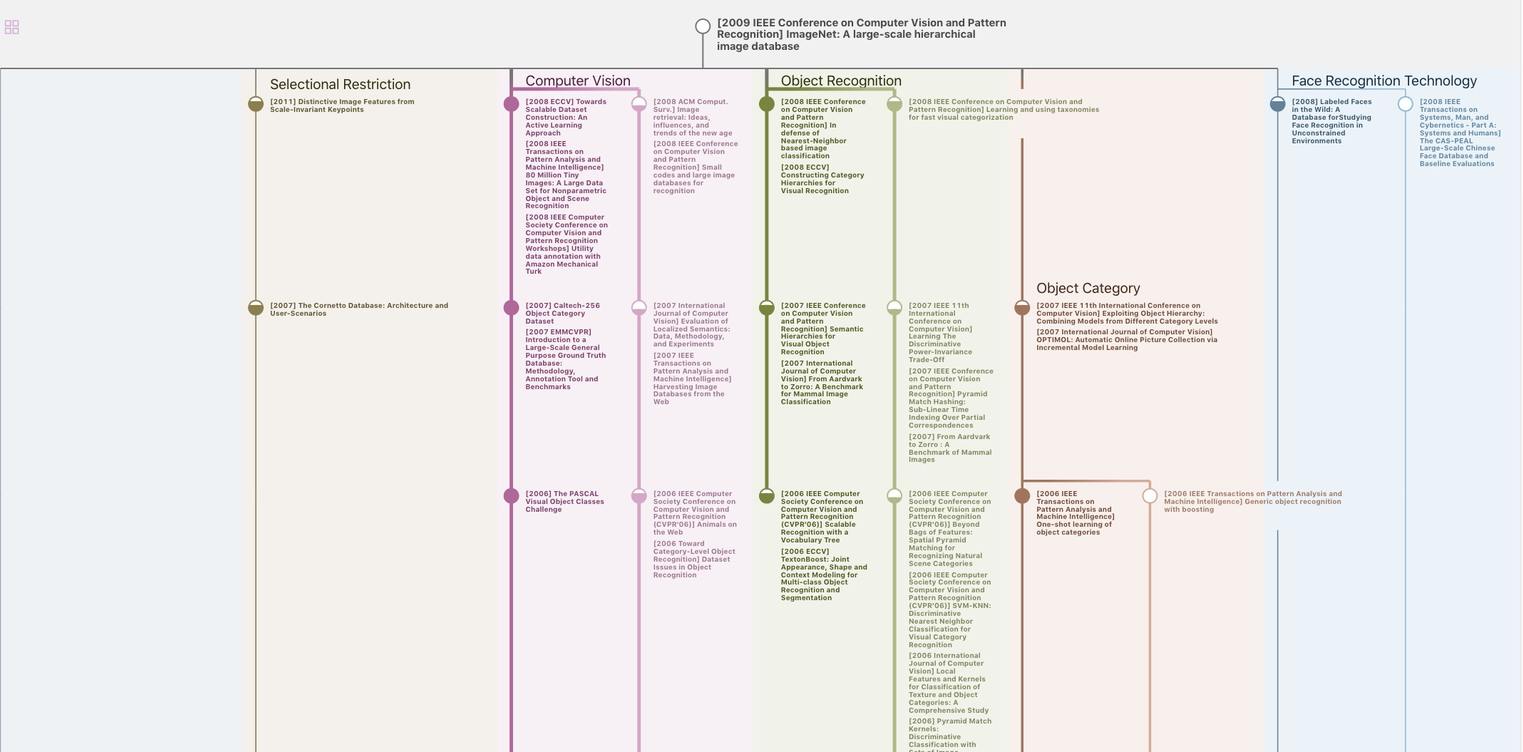
生成溯源树,研究论文发展脉络
Chat Paper
正在生成论文摘要