SPN: Stable Prototypical Network for Few-Shot Learning-Based Hyperspectral Image Classification
IEEE geoscience and remote sensing letters(2022)
摘要
We tackle the problem of few-shot image classification in the context of remote sensing hyperspectral images (HSIs). Due to the difficulties in collecting a large number of labeled training samples, the few-shot classification techniques hold much prominence in remote sensing in general. One of the bottlenecks in designing few-shot learning (FSL) systems arises from the fact that the model is likely to overfit in the presence of few training samples and the complex spectral feature distributions of the land-cover classes. To this end, we introduce a stable prototypical network (SPN) for FSL by judiciously incorporating dropout and DropBlock-based regularizers jointly within the framework and averaging model parameters using the Monte Carlo approximation. Besides, a novel variance loss term to reduce the uncertainty of the network is considered together with the cross-entropy-based classification loss to train the model in an end-to-end manner. The experimental analysis on three benchmark HSI datasets confirms the SPN’s superior performance.
更多查看译文
关键词
Training,Feature extraction,Uncertainty,Data models,Monte Carlo methods,Deep learning,Neurons,DropBlock,few-shot learning (FSL),hyperspectral image (HSI) classification,uncertainty
AI 理解论文
溯源树
样例
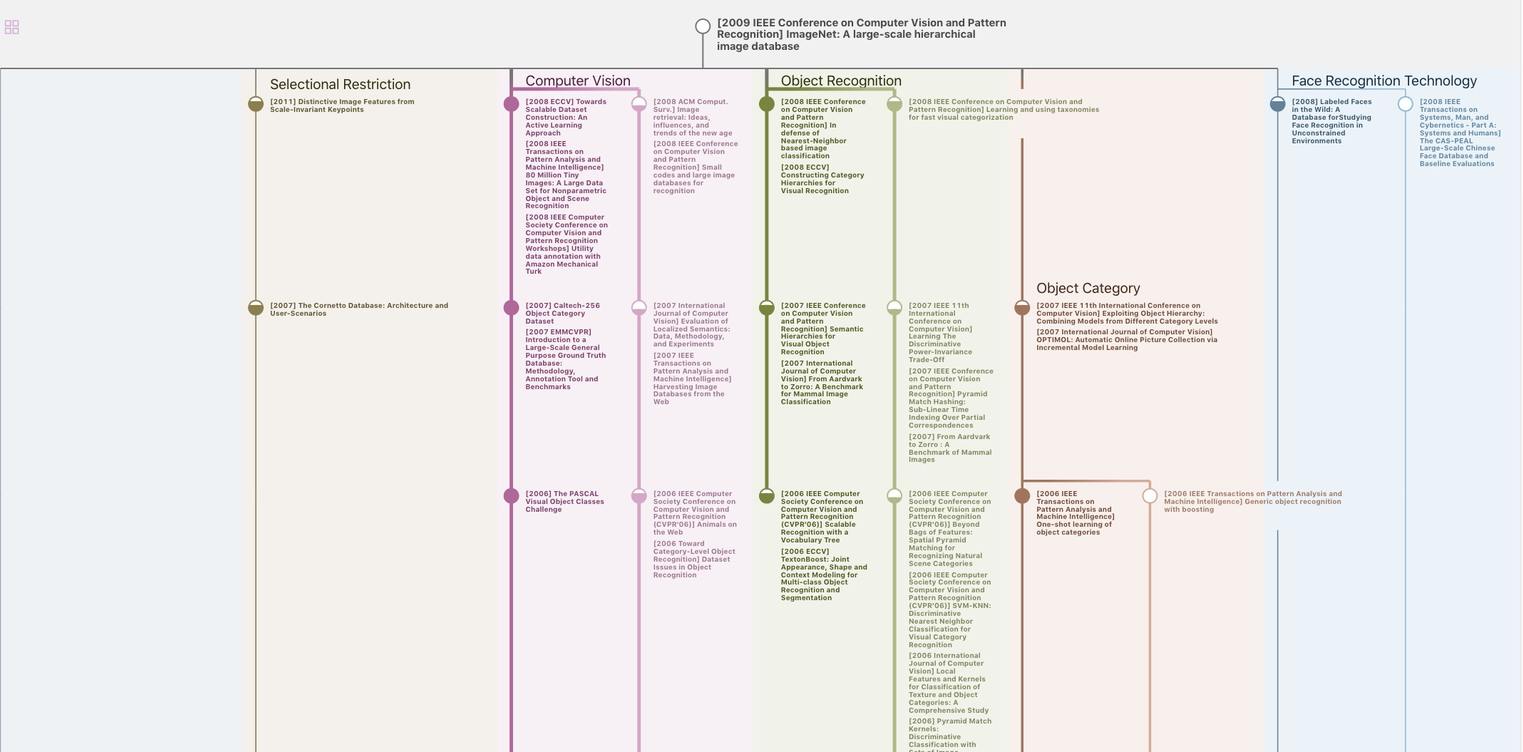
生成溯源树,研究论文发展脉络
Chat Paper
正在生成论文摘要