Use of a BP Neural Network and Meteorological Data for Generating Spatiotemporally Continuous LAI Time Series
IEEE TRANSACTIONS ON GEOSCIENCE AND REMOTE SENSING(2022)
摘要
Spatiotemporally continuous long-term leaf area index (LAI) products are urgently needed to monitor environmental changes. The current filter- or curve-fitting-based time series reconstructive algorithms fail to reconstruct the LAI time series with many continuous missing values or missing values in key phenological periods, which are common issues in high-spatial-resolution LAI time series. In this article, a meteorological data-driven backpropagation neural network (MBPNN) was proposed to reconstruct discontinuous LAI profiles with a two-step process using vegetation phenological information. As the basis of the strong dependence of vegetation growth on meteorological conditions, a reasonable growth trajectory of reconstructed LAI can be guaranteed by the algorithm even though if many observed values are missing. Validations for reconstructed LAI were conducted both spatially and temporally based on reference maps and field-measured long-term observations. The results showed that the LAI predicted by the MBPNN had a similar accuracy (RMSE = 0.4076) as the Landsat LAI inversions (RMSE = 0.4083) and a similar reconstructed trajectory as the field-measured LAI series even though over 100 days of continuous data were missing (RMSE = 0.1620). A comparison with the Harmonic ANalysis of Time Series (HANTS) algorithm showed that the accuracy of MBPNN was more stable regardless of the size/position of the missing data, and the proposed method performed much better when the data were continuously missing for 50 days or more.
更多查看译文
关键词
Time series analysis,Remote sensing,Earth,Artificial satellites,Vegetation mapping,MODIS,Image reconstruction,Landsat data,leaf area index (LAI),meteorological data,phenology,propagation neural network,reconstruction,time series
AI 理解论文
溯源树
样例
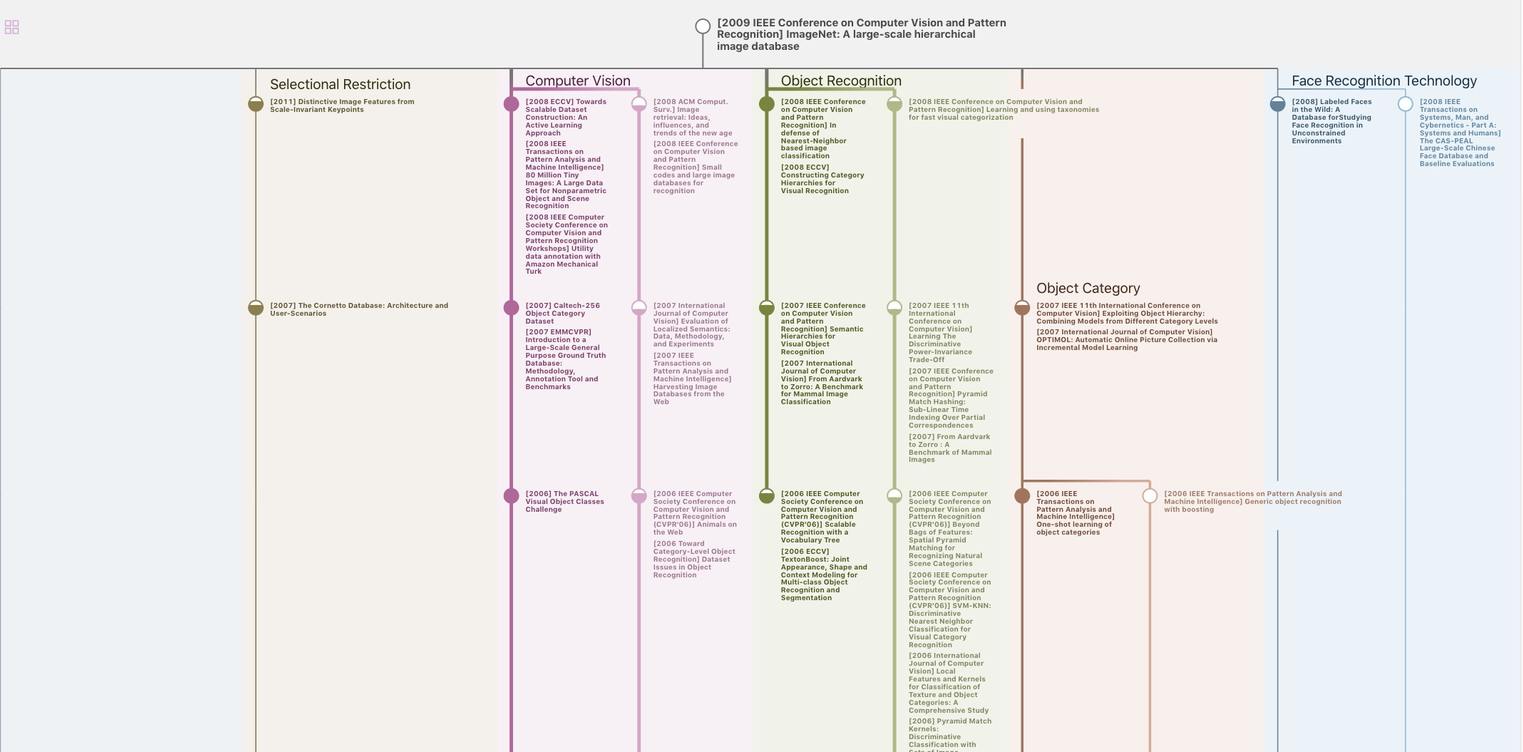
生成溯源树,研究论文发展脉络
Chat Paper
正在生成论文摘要