Development and Validation of a Non-Invasive Model for Predicting Liver Cirrhosis in Patients with Chronic Hepatitis B
Social Science Research Network(2020)
摘要
Background: Liver cirrhosis is closely related to the abnormal liver function and occurrence of liver cancer. Accurate non-invasive assessment of liver cirrhosis is of great significance for prevent disease progression and treatment decision-making. We aimed to develop and validate a non-invasive prediction model for liver cirrhosis in patients with chronic hepatitis B.
Methods: We enrolled 1350 patients with chronic hepatitis B to develop the prediction model, and clinical data was collected from July 2015 to May 2017. Least absolute shrinkage and selection operator (Lasso) regression was used for feature selection and binary logistic regression analysis was chosen to build prediction model. For convenient usage, prediction model was presented as a nomogram and evaluated for calibration, discrimination and clinical usefulness. We prospectively validated the model in an independent cohort contained 421 consecutive patients from April 2017 to November 2017.
Findings: The prediction model, which consists of 12 selection clinical characteristics, showed the strongest correlation with liver fibrosis stage (ρ = 0.53, P <.05). Compared with 2D-SWE, APRI, FIB-4, King’s Score, and Forns Index, the model presented the optimal discrimination and the best predictive performance with the highest AUC in the training cohort (0.8067, 95%CI 0.7834-0.8296, P <.05) and validation cohorts (0.7965, 95%CI 0.7547-0.8384, P <.05). Decision curve analysis demonstrated that nomogram based on the model was extremely useful for predicting cirrhosis in patients with chronic hepatitis B.
Interpretation: This study proposes a non-invasive prediction model that incorporates the clinical predictors which can be conveniently used in the individualized prediction of liver cirrhosis in patients with chronic hepatitis B.
Funding Statement: Supported by the National Natural Science Foundation of China (81372650; 81401929), Shanghai Rising-Star Program (16QA1401000) and National Science and Technology Major Project (2018ZX10723204-004).
Declaration of Interests: The authors declare no conflicts of interest relevant to this manuscript.
Ethics Approval Statement: This study protocol was approved by the Ethics Committee of Zhongshan Hospital, Fudan University, and informed consent was obtained from all patients.
更多查看译文
AI 理解论文
溯源树
样例
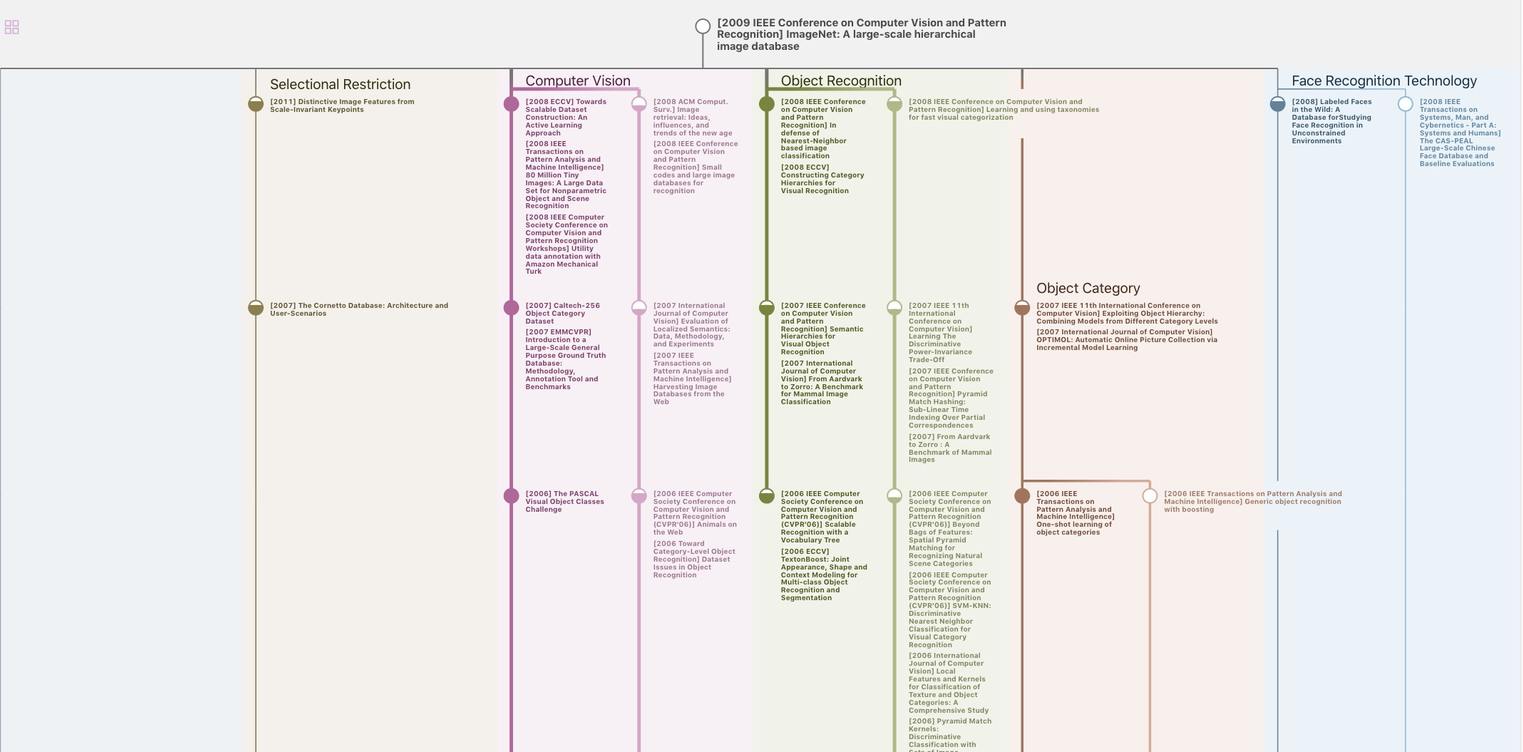
生成溯源树,研究论文发展脉络
Chat Paper
正在生成论文摘要