Theoretical Unification and Practical Integration of Conventional Methods and Genomic Selection in Plant Breeding
Crop Breeding, Genetics and Genomics(2019)
摘要
Following successful application in dairy cow breeding, genomic selection (GS) has become a hot topic among plant geneticists and breeders. GS and conventional methods have the same goal of identifying best genotypes for a given crop and region, and should follow the same principles, particularly in dealing with genotype-by-environment (GE). Dealing with GE includes dividing a target region into meaningful mega-environments (MEs) based on repeatable GE patterns and then selecting for each ME. This requires different GS models be developed for different MEs. Selection for each ME requires testing at multiple locations for multiple years to account for the unrepeatable GE in the ME and to estimate the genetic main effect, GP. For a ME with large GE, multiple and diverse cultivars should be selected and recommended. The number of locations, years, and replications within trials required to achieve a certain level of heritability (i.e., selection reliability) for a trait (e.g., yield) can be estimated from existing multi-location, multiyear variety trial data. Instead of direct selection for GP, conventional selections have to resort to indirect selection, negative selection (culling), and a lengthy breeding cycle. GS offers the possibility for direct selection and positive selection for GP and thereby overcoming random GE and shortening the breeding cycle. To achieve this, GS models must be able to predict GP for a breeding population and its prediction accuracy should be measured by r(GM,GP), GM being GS predicted breeding values. This definition of prediction accuracy is the essential connection between GS and phenotype-based selection. This implies that GS models must be developed and evaluated using phenotypic data from multiple locations and multiple years representing the target ME. Using a single training population for model development and a different population for model evaluation will allow estimation of r(GM,GP), but it can be costly and may have limited relevance to a breeding program. A pragmatic GS framework was proposed in this paper, which is to use data from yearly preliminary yield trials for model development, in which a large number of new breeding lines are tested at several locations. A GS model can be developed for each training population-by-trial (location-year) combination, and all available GS models can be used to make predictions, leading to a genotype-by-model two-way table of predictions for the current-year’s breeding population. This table can then be analyzed the way a breeder would do to a genotype-by-environment two-way table of a trait to make selection decisions. This table can also be used to evaluate and select models as a breeder would do for their test environments. Instead of r(GM,GP), “rate of success”, defined as the inverse of the number of genotypes that have to go through yield trials in order to identify a new cultivar, can be used to measure the prediction accuracy of GS. The prediction accuracy or rate of success determines the best pathway to integrate GS into a practical breeding program. GS prediction accuracy is expected to improve with time as more GS models are developed and as the breeding populations and the target ME are better represented in the GS models. A real multi-location, multi-year oat (Avena saliva L.) variety trial dataset and a practical oat breeding program were used to facilitate the discussions
更多查看译文
AI 理解论文
溯源树
样例
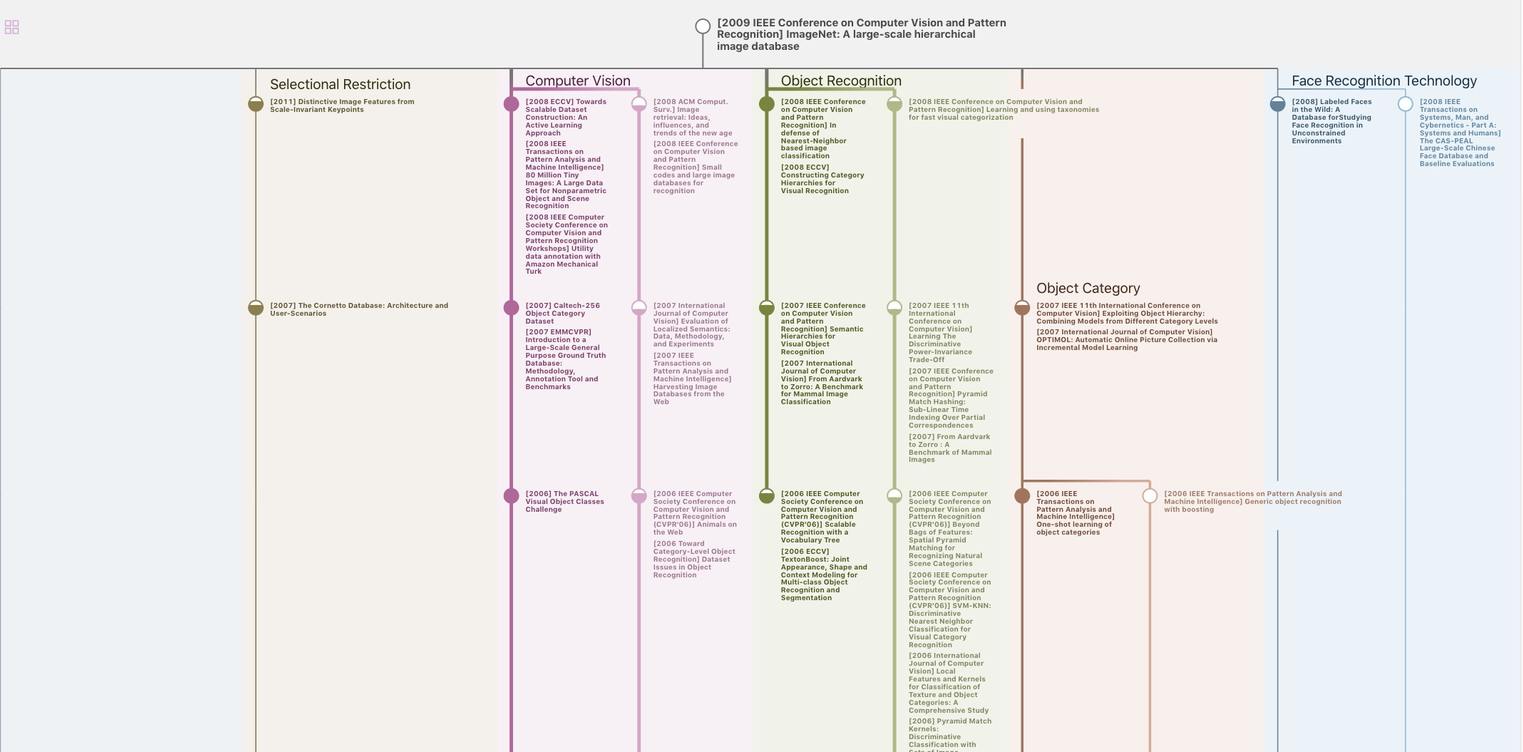
生成溯源树,研究论文发展脉络
Chat Paper
正在生成论文摘要