Multimodal Hierarchical Attention Neural Network: Looking for Candidates Behaviour Which Impact Recruiter's Decision
IEEE Transactions on Affective Computing(2023)
摘要
Automatic analysis of job interviews has gained in interest amongst academic and industrial research. The particular case of asynchronous video interviews allows to collect vast corpora of videos where candidates answer standardized questions in monologue videos, enabling the use of deep learning algorithms. On the other hand, state-of-the-art approaches still face some obstacles, among which the fusion of information from multiple modalities and the interpretability of the predictions. We study the task of predicting candidates performance in asynchronous video interviews using three modalities (verbal content, prosody and facial expressions) independently or simultaneously, using data from real interviews which take place in real conditions. We propose a sequential and multimodal deep neural network model, called Multimodal HireNet. We compare this model to state-of-the-art approaches and show a clear improvement of the performance. Moreover, the architecture we propose is based on attention mechanism, which provides interpretability about which questions, moments and modalities contribute the most to the output of the network. While other deep learning systems use attention mechanisms to offer a visualization of moments with attention values, the proposed methodology enables an in-depth interpretation of the predictions by an overall analysis of the features of social signals contained in these moments.
更多查看译文
关键词
Interviews,Databases,Face recognition,Feature extraction,Visualization,Neural networks,Deep learning,Nonverbal signals,employment,human resources,job interviews,neural nets,deep learning,multimodal systems,interpretability
AI 理解论文
溯源树
样例
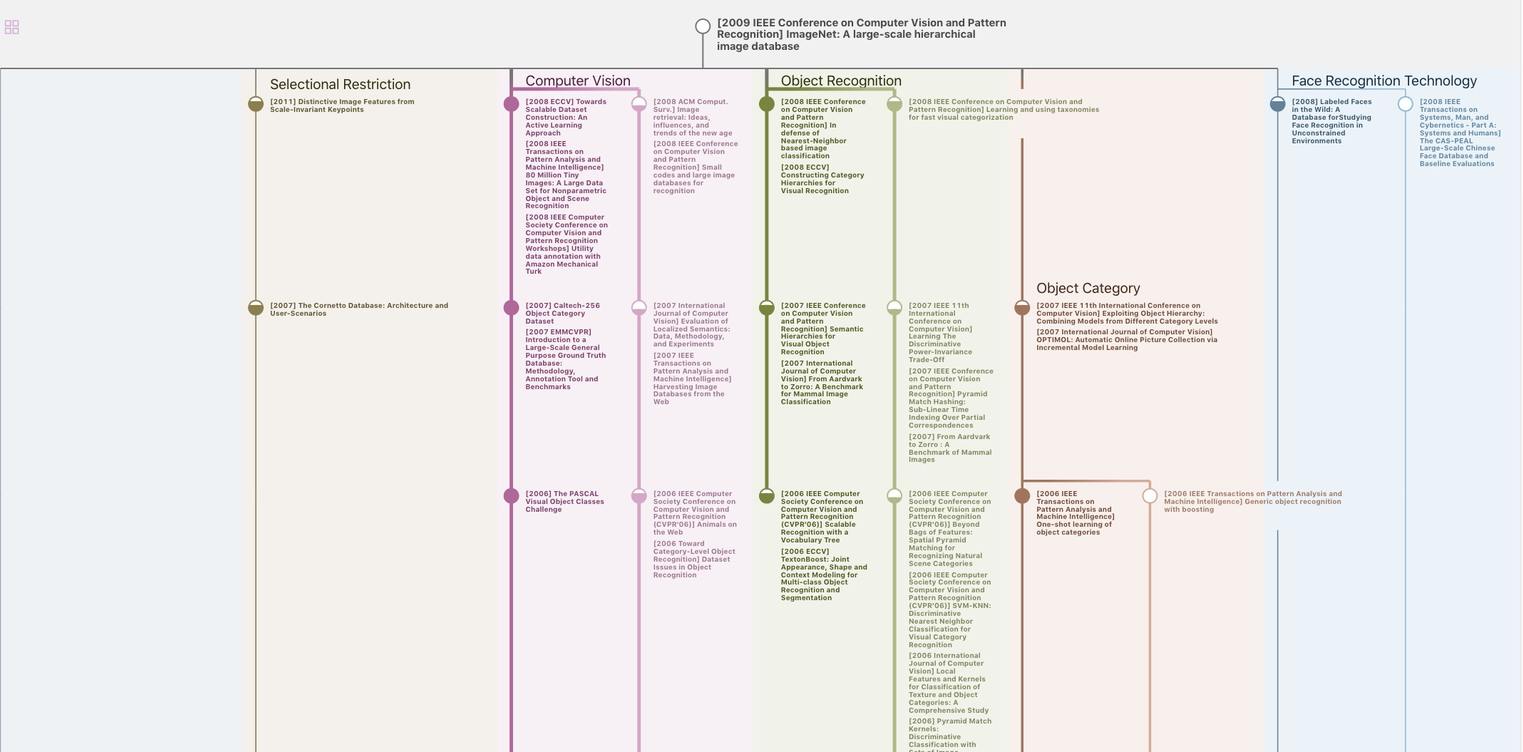
生成溯源树,研究论文发展脉络
Chat Paper
正在生成论文摘要