Support Matrix Regression for Learning Power Flow in Distribution Grid with Unobservability
IEEE transactions on power systems(2022)
摘要
Increasing renewable penetration in distribution grids calls for improved monitoring and control, where power flow (PF) model is the basis for many advanced functionalities. However, unobservability makes the traditional way infeasible to construct PF analysis via admittance matrix for many distribution grids. While data-driven approaches can approximate PF mapping, direct machine learning (ML) applications may suffer from several drawbacks. First, complex ML models like deep neural networks lack the degradability and explainability to the true system model, leading to overfitting. There are also asynchronization issues among different meters without GPS chips. Last but not least, bad data is quite common in the distribution grids. To resolve these problems all at once, we propose a variational support matrix regression (SMR). It provides structural learning to (1) embed kernels to regularize physical form in observable area while achieving good approximation at unobservable area, (2) integrate temporal information into matrix regression for asynchronized data imputation, and (3) define support matrix for margins to be robust against bad data. We test the performance for mapping rule learning via IEEE test systems and a utility distribution grid. Simulation results show high accuracy, degradability from data-driven model to physical model, and robustness to data quality issues.
更多查看译文
关键词
Data models,Kernel,Power measurement,Voltage measurement,Measurement uncertainty,Mathematical model,Data integrity,Distribution grids,data-driven method,power flow mapping recovery,support matrix regression,unobservability
AI 理解论文
溯源树
样例
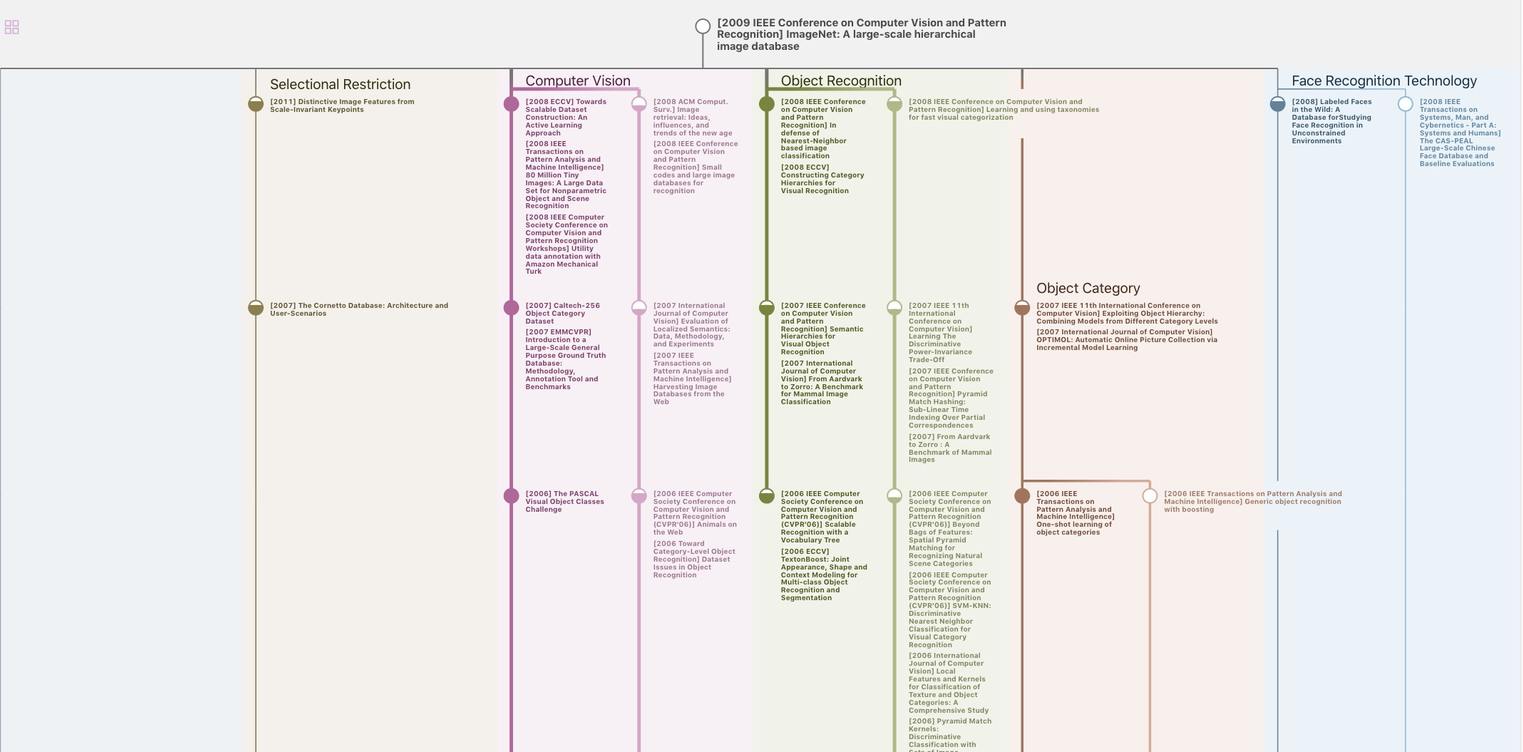
生成溯源树,研究论文发展脉络
Chat Paper
正在生成论文摘要