A 65-nm Energy-Efficient Interframe Data Reuse Neural Network Accelerator for Video Applications
IEEE Journal of Solid-State Circuits(2022)
摘要
An energy-efficient convolutional neural network (CNN) accelerator is proposed for the video application. Previous works exploited the sparsity of differential (Diff) frame activation, but the improvement is limited as many Diff-frame data is small but non-zero. Processing of irregular sparse data also leads to low hardware utilization. To solve these problems, two key innovations are proposed in this article. First, we implement a hybrid-precision inter-frame-reuse architecture which takes advantage of both low bit-width and high sparsity of Diff-frame data. This technology can accelerate 3.2
$\times $
inference speed with no accuracy loss. Second, we design a conv-pattern-aware processing array that achieves the 2.48
$\times $
–14.2
$\times $
PE utilization rate to process sparse data for different convolution kernels. The accelerator chip was implemented in 65-nm CMOS technology. To the best of our knowledge, it is the first silicon-proven CNN accelerator that supports inter-frame data reuse. Attributed to the inter-frame similarity, this video CNN accelerator reaches the minimum energy consumption of 24.7
$\mu \text{J}$
/frame in the MobileNet-slim model, which is 76.3% less than the baseline.
更多查看译文
关键词
Activation sparsity,hybrid precision,interframe data reuse,neural network accelerator,video
AI 理解论文
溯源树
样例
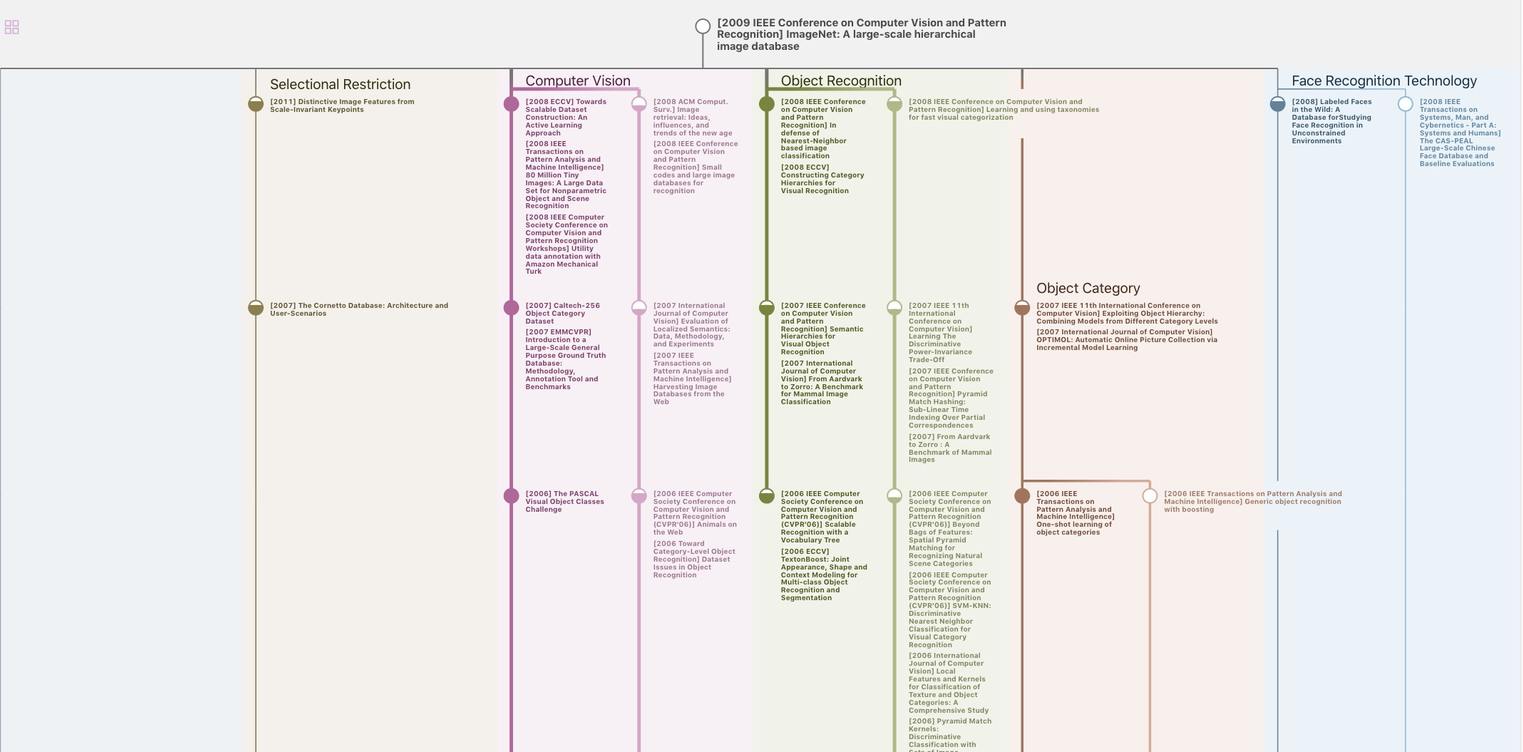
生成溯源树,研究论文发展脉络
Chat Paper
正在生成论文摘要