Predicting shock-waves in radiograph images using different deep learning models
Applications of Machine Learning 2021(2021)
摘要
We demonstrate and analyze a new methodology for detecting and characterizing shocks in high energy density physics (HEDP) experiments using simulated radiographs. Our method consists of simulating many variations of an HEDP experiment using a multi-physics modeling code developed at Los Alamos National Laboratory. These simulations are then used to produce synthetic radiographs that emulate the actual experimental data collection setup. Shock contours are defined by the peaks of the density derivative values obtained at each radial coordinate of an X-rayed cylindrical object, giving us a ground truth of shock position that would not be available directly from the observed radiograph. Convolutional neural networks are then trained on our simulations, mapping radiograph to shock structure. We investigate four different state-of-the-art deep convolutional neural networks, Xception, ResNet152, VGG19, and U-Net, for use in regressing the HEDP radiograph to the shock position. It is demonstrated that our neural network approach offers a highly accurate shock locator. We find that the different network architectures are better tuned for locating distinct shock characteristics, equivalent to detecting shock-waves at multiple scales. Differences are quantified by ranking the four architectures by their overall performance accuracy. The regression model based on the Xception architecture is found to yield the highest accuracy. In order to understand limitations of these techniques to external perturbations in the experimental setup we also apply our trained networks to shock location in the presence of Gaussian and Flatfield noise. We find that the network shock-locations are surprisingly robust to noise giving confidence that they will perform well on experimental data.
更多查看译文
关键词
radiation shock, radiographs, deep convolutional neural networks, regression
AI 理解论文
溯源树
样例
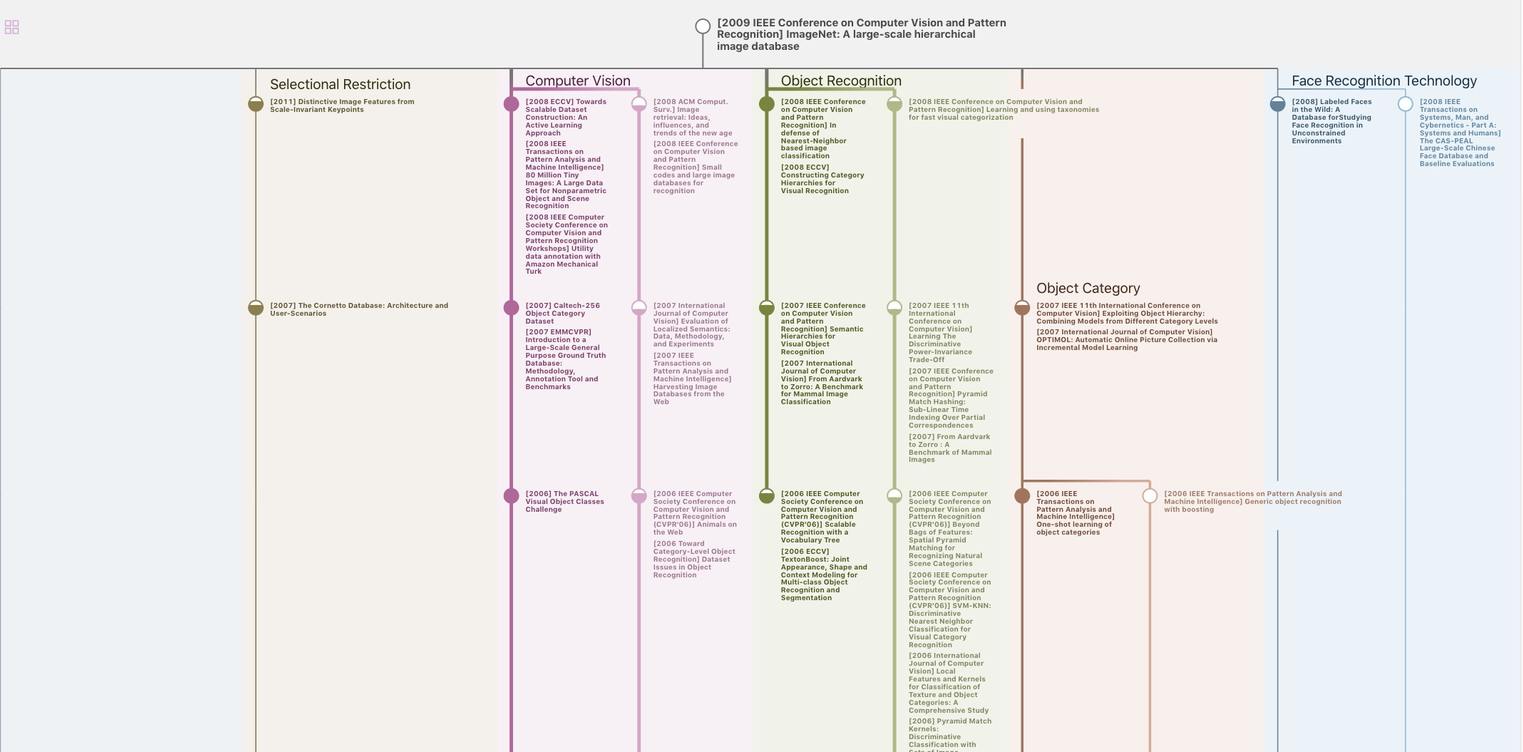
生成溯源树,研究论文发展脉络
Chat Paper
正在生成论文摘要