Fault Detection Utilizing Convolution Neural Network on Timeseries Synchrophasor Data from Phasor Measurement Units
IEEE Transactions on Power Systems(2021)
摘要
— An end-to-end supervised learning method is proposed for fault detection in the electric grid using Big Data from multiple Phasor Measurement Units (PMUs). The approach consists of preprocessing steps aimed at reducing data noise and dimensionality, followed by utilization of six classification models considered for detecting faults. Three of the models were variants of Convolutional Neural Network (CNN) architectures that consider a single type of measurement (voltage, current or frequency) at all PMUs or all types together also at all PMUs. CNN based models were compared to traditional methods of Logistic Regression (LR), Multi-layer Perceptron (MLP) and Support Vector Machine (SVM). Evaluation was conducted on two-year data measured by PMUs at 37 locations in a large electric grid. The response variable for classification were extracted from the grid-wide outage event log. Experiments show that CNN-based models outperformed traditional methods on one year out-of-sample outage detection over the entire grid.
更多查看译文
关键词
Big data applications,event detection,machine learning,phasor measurement units,power system faults,dimensionality reduction,smart grids,time series analysis,neural networks,convolutional neural networks
AI 理解论文
溯源树
样例
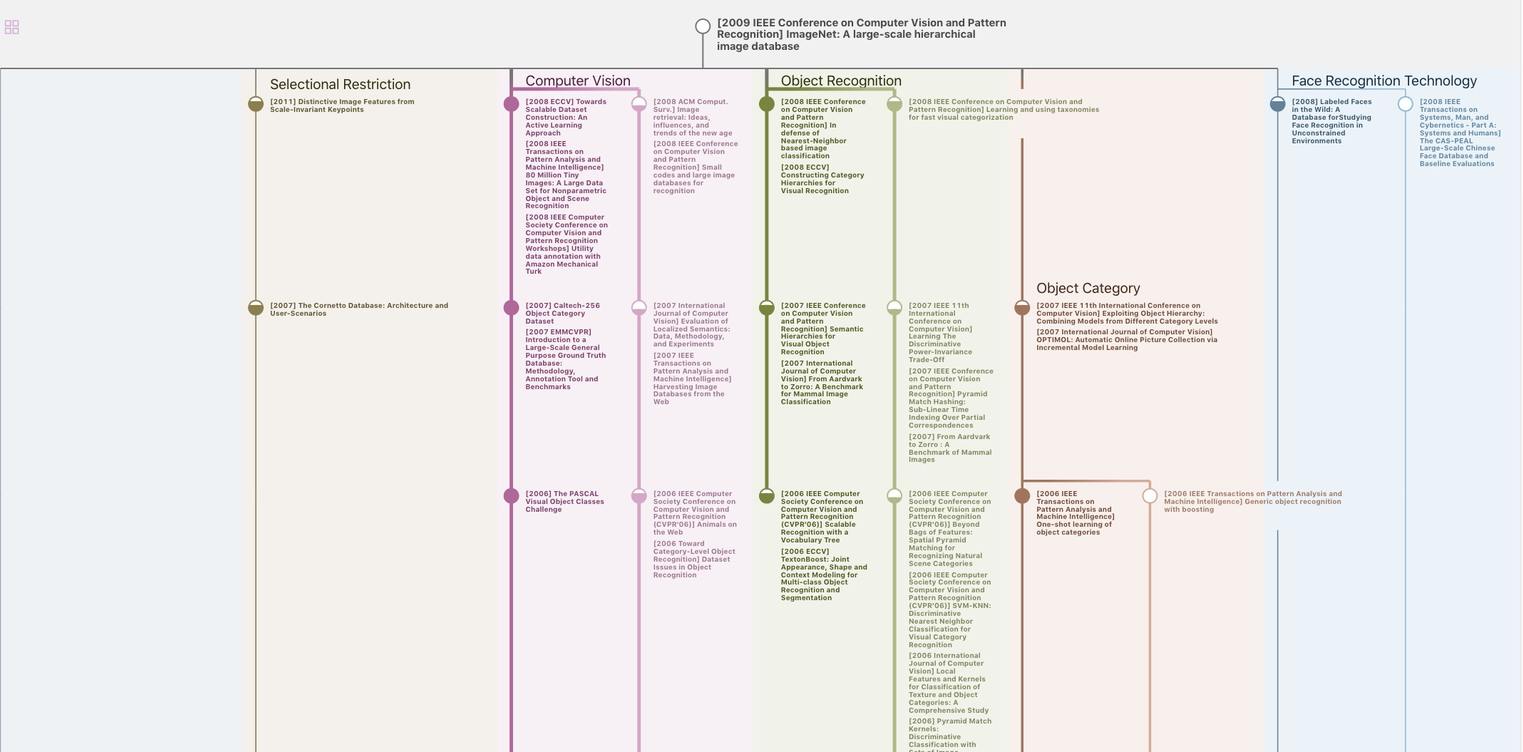
生成溯源树,研究论文发展脉络
Chat Paper
正在生成论文摘要