GradientFlow: Optimizing Network Performance for Large-Scale Distributed DNN Training
IEEE Transactions on Big Data(2019)
摘要
It is important to scale out deep neural network (DNN) training for reducing model training time. The high communication overhead is one of the major performance bottlenecks for distributed DNN training across multiple GPUs. Our investigations have shown that popular open-source DNN systems could only achieve 2.5 speedup ratio on 64 GPUs connected by 56 Gbps network. To address this problem, we propose a communication backend named GradientFlow for distributed DNN training, and employ a set of network optimization techniques. First, we integrate ring-based allreduce, mixed-precision training, and computation/communication overlap into GradientFlow. Second, we propose lazy allreduce to improve network throughput by fusing multiple communication operations into a single one, and design coarse-grained sparse communication to reduce network traffic by only transmitting important gradient chunks. When training AlexNet and ResNet-50 on the ImageNet dataset using 512 GPUs, our approach could achieve 410.2 and 434.1 speedup ratio respectively.
更多查看译文
关键词
Training,Graphics processing units,Computational modeling,Servers,Data models,Computer architecture,Bandwidth
AI 理解论文
溯源树
样例
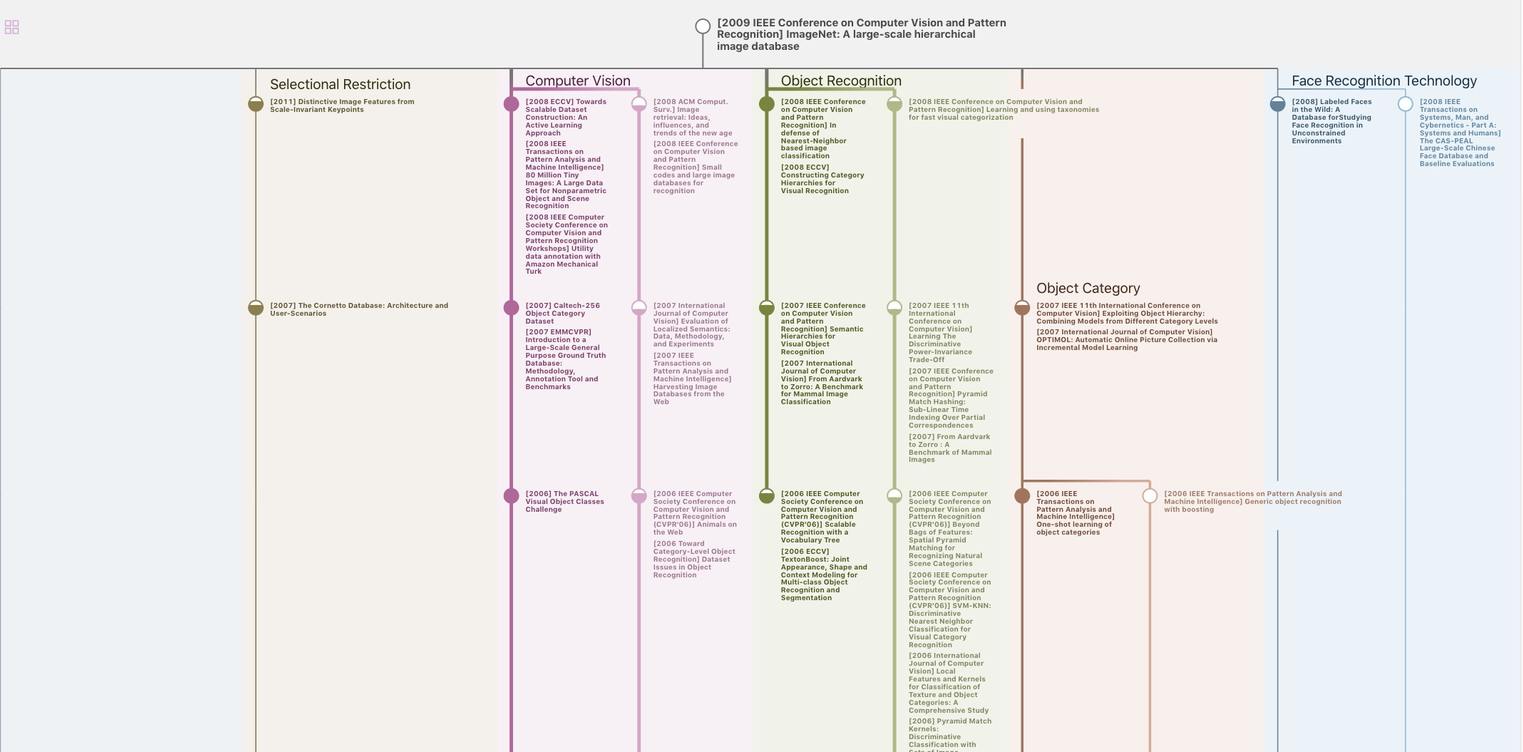
生成溯源树,研究论文发展脉络
Chat Paper
正在生成论文摘要