Grafnet: Using Graph Neural Networks to Create Table-Less Routers
IEEE transactions on network science and engineering(2022)
摘要
Grafnet, a Graph Neural-Network (GNN)-based scheme learns IP-address-to-port mapping, leading to forwarding table-less routers. GNNs allow mapping network-wide features like adjacencies and addresses to generate new representations. Grafnet converts network-wide IP addresses to a feature space using GNNs. GNNs extrapolate node adjacencies onto a feature matrix, whose output tells which address/subnet is connected to a node and port. To do so, we use a GNN in conjunction with an Artificial Neural Network (ANN), whose output transforms graph adjacencies to address-based adjacencies. We exploit the fact that IP addresses are present in contiguous groups (subnets) or ‘ranges’. Large range sizes imply a better likelihood of Grafnets’ approximation, though with enough learning Grafnet learns just about all network-wide IP addresses, irrespective of range sizes. Grafnet is evaluated as an SDN scheme on (1) 75-node US-core network and (2) 2000-node, 5 million IP address-based random WAN topology. Analytically, we show equivalence between Grafnet and a Feed-forward neural network implying exhaustiveness and correctness. The proposed Grafnet model is able to work as a direct address translator without the need for tables in the forwarding plane of a router. Engineering considerations to implement Grafnet are also discussed.
更多查看译文
关键词
IP networks,Routing protocols,Computational modeling,Training,Routing,Delays,Throughput,Table-less routers,machine learning in routing,SDN
AI 理解论文
溯源树
样例
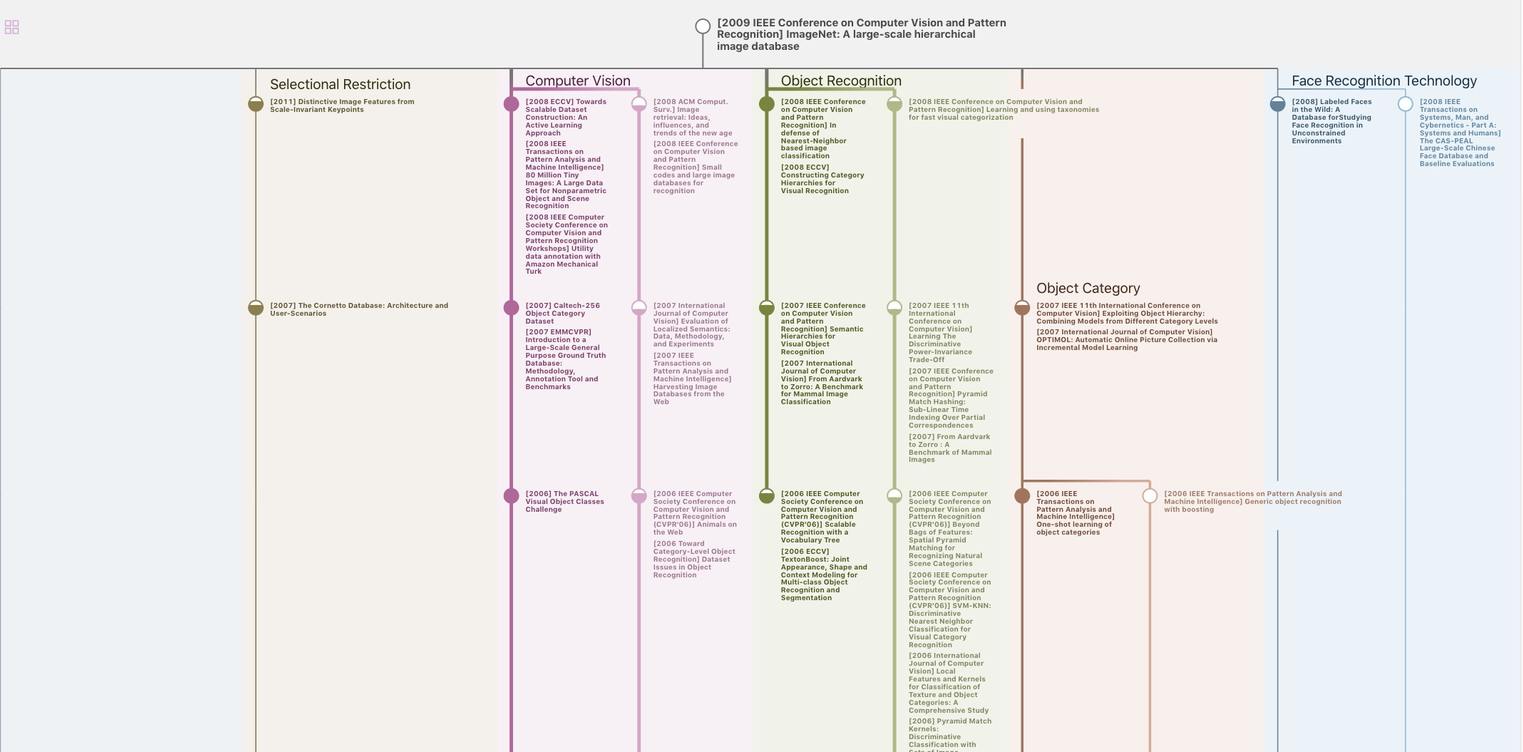
生成溯源树,研究论文发展脉络
Chat Paper
正在生成论文摘要