Fault-Prototypical Adapted Network for Cross-Domain Industrial Intelligent Diagnosis
IEEE Transactions on Automation Science and Engineering(2022)
摘要
Despite rapid advances in machine learning based fault diagnosis, their identical distribution assumption of the training (source domain) and testing data (target domain) is generally challenged in industrial applications due to the variation of working conditions. In this article, a fault-prototypical adapted network (FPAN) is proposed, which enables cross-domain industrial intelligent fault diagnosis aided by deep transfer learning. First, a similarity learning-based discrimination module is designed to learn fault prototypes (FPs) that are representative for each fault and discriminative across different faults. Then, a fault prototypical-adaptation module is developed, which adapts the multiple FPs to the target dataset and enables more precise category-wise domain invariance. The two modules are trained simultaneously to extract transferrable and discriminative FPs, by which the cross-domain intelligent diagnosis can be readily achieved. Experimental results on two industrial cases illustrate that the proposed approach learns transferable feature representations that better reduce domain discrepancy, and provides improved diagnosis performance on target data. Note to Practitioners—As the distribution of source and target domain data may differ due to the varying working conditions, cross-domain intelligent fault diagnosis plays an increasingly important role in practical industrial cases. This work presents the FPAN model, in which the fault discrimination and the category-wise adaptation are naturally connected and unified by exploring representative virtual FP for each category, irrespective of which domain that the category comes from. Connected by the adaptive FPs, the similarity learning-based discrimination and the fault-prototypical adaptation modules can benefit from each other to boost the target learning. Finally, the cross-domain fault diagnosis can be performed based on the similarities with different FP representations. The proposed method is readily applicable to various intelligent fault diagnosis problems, including the mechanical cases and the industrial processes.
更多查看译文
关键词
Cross-domain fault diagnosis,deep representation learning,fault prototypes,transfer learning
AI 理解论文
溯源树
样例
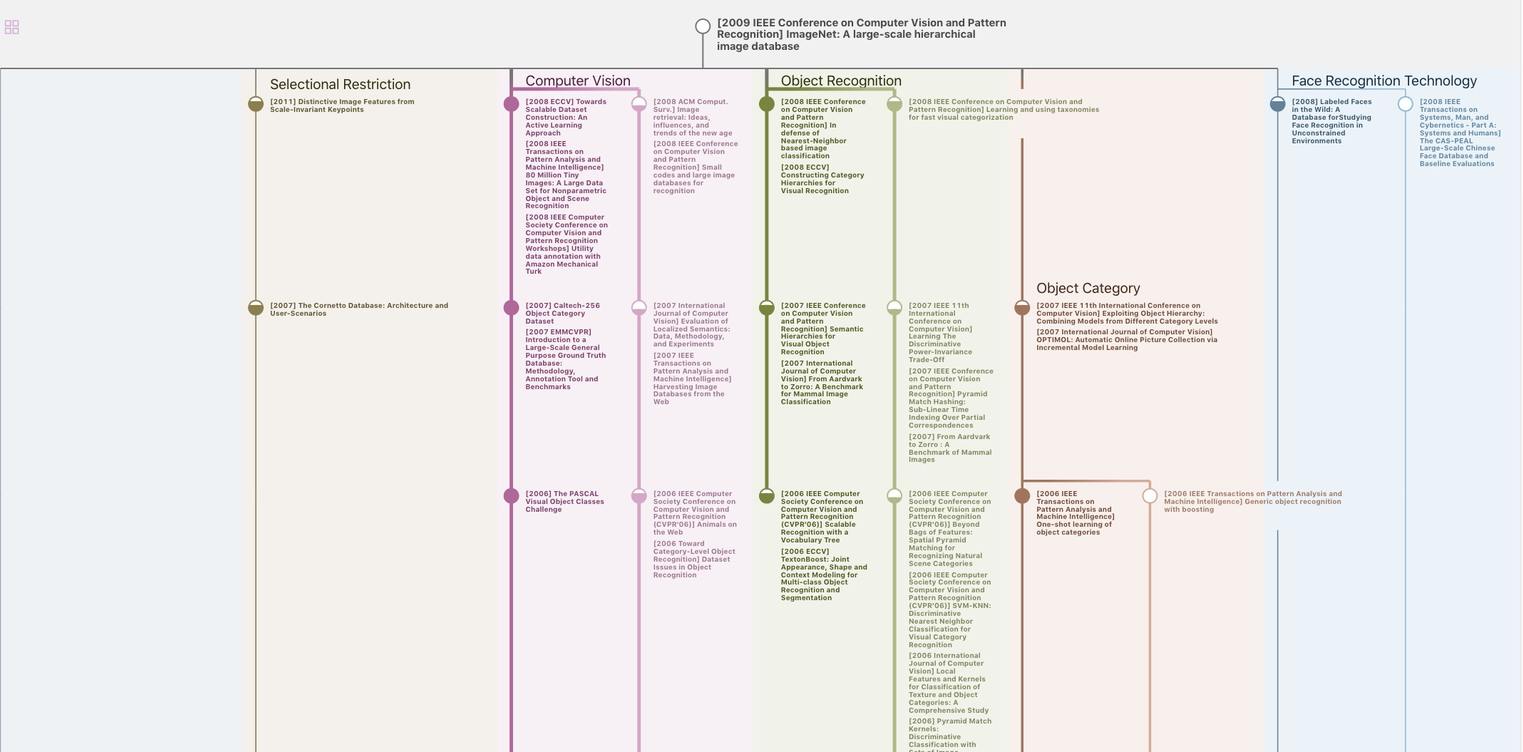
生成溯源树,研究论文发展脉络
Chat Paper
正在生成论文摘要