Impact identification of composite cylinder based on improved deep metric learning model and weighted fusion Tikhonov regularized total least squares
Composite Structures(2022)
摘要
Composite materials are widely used in aerospace field due to the characteristics of light weight and high strength. As the composites are prone to internal damage that is not visible under low-velocity impact (LVI), the localization and reconstruction of impact force are of great importance to the health monitoring of composite structures. Inspired by the idea of deep metric learning and total least squares, a two-stage method is proposed for random impact force localization and reconstruction. In the first stage, circular neighbor negative sample mining and improved Multi-Similarity loss with adaptive additive margin softmax (IMS-AAM) are proposed in deep networks to extract the features of impact signals firstly. Then, Siamese network architecture combined cosine similarity is employed to give the coordinates of unknown impact forces. In the second stage, weighted fusion Tikhonov regularized total least squares(WF-TRTLS) is proposed to realize random impact reconstruction by fusing the results of neighbor training points. The proposed method is validated on a composite cylinder structure with various comparison tests. The results show that the proposed method can obtain quite low localization errors on the three-dimensional composite structure, while robust and accurate reconstruction can be achieved even under high noise level.
更多查看译文
关键词
Structural health monitoring,Impact localization,Reconstruction of impact force,Deep metric learning,Information fusion,Regularization
AI 理解论文
溯源树
样例
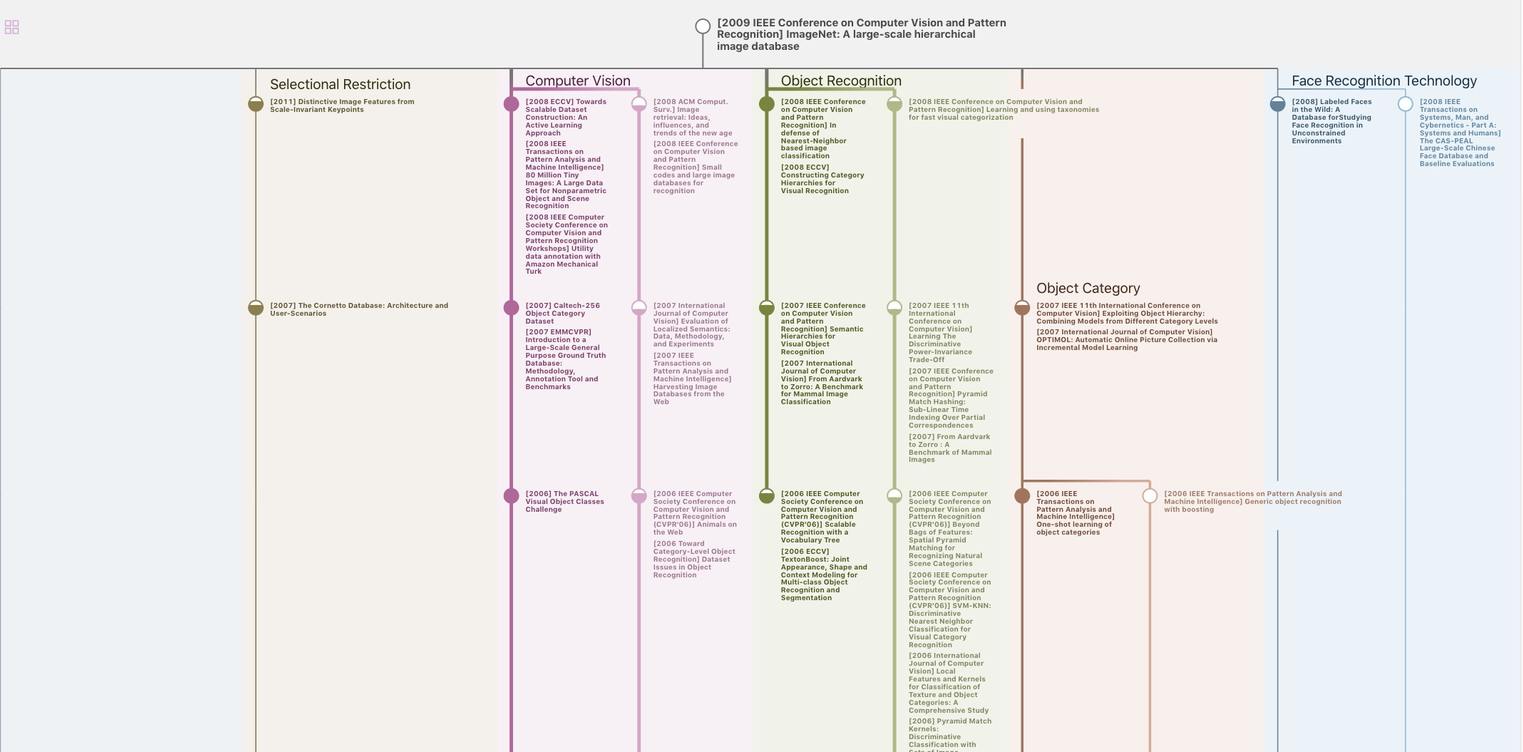
生成溯源树,研究论文发展脉络
Chat Paper
正在生成论文摘要