MRDFlow: Unsupervised Optical Flow Estimation Network With Multi-Scale Recurrent Decoder
IEEE Transactions on Circuits and Systems for Video Technology(2022)
摘要
Optical flow estimation is a fundamental task in computer vision and image processing. Due to the difficulty in obtaining the ground truth of flow field, unsupervised learning approaches attract more and more research interests in recent years. However, despite of their good generalization capability, unsupervised optical flow methods suffer in the scenarios with large displacement, small objects, and occlusions. In this work, we propose a novel optical flow network based on decoder with multi-scale kernels. Different from previous U-Net like or pyramidal methods, we design our network based on RAFT architecture that with a 4D correlation layer and recurrent decoder. More importantly, we incorporate three novel ideas with regard to the input, information processing and output of the update units improve the performance. Firstly, we utilize various motion-related information as input to the update units. Secondly, we propose a module of multi-scale update unit. Thirdly, for the final flow up-sampling procedure, we propose an image-guided up-sampling loss to guide the learning of up-sampling masks. Our model is trained by the occlusion-aware photometric loss, edge-aware smoothness loss, self-supervised loss, and image-guided up-sampling loss. Experimental results demonstrate that our model achieves the state-of-the-art performance on both Sintel and KITTI and outperforms other unsupervised optical flow methods remarkably.
更多查看译文
关键词
Optical flow,unsupervised learning,multi-scale decoder,recurrent decoder
AI 理解论文
溯源树
样例
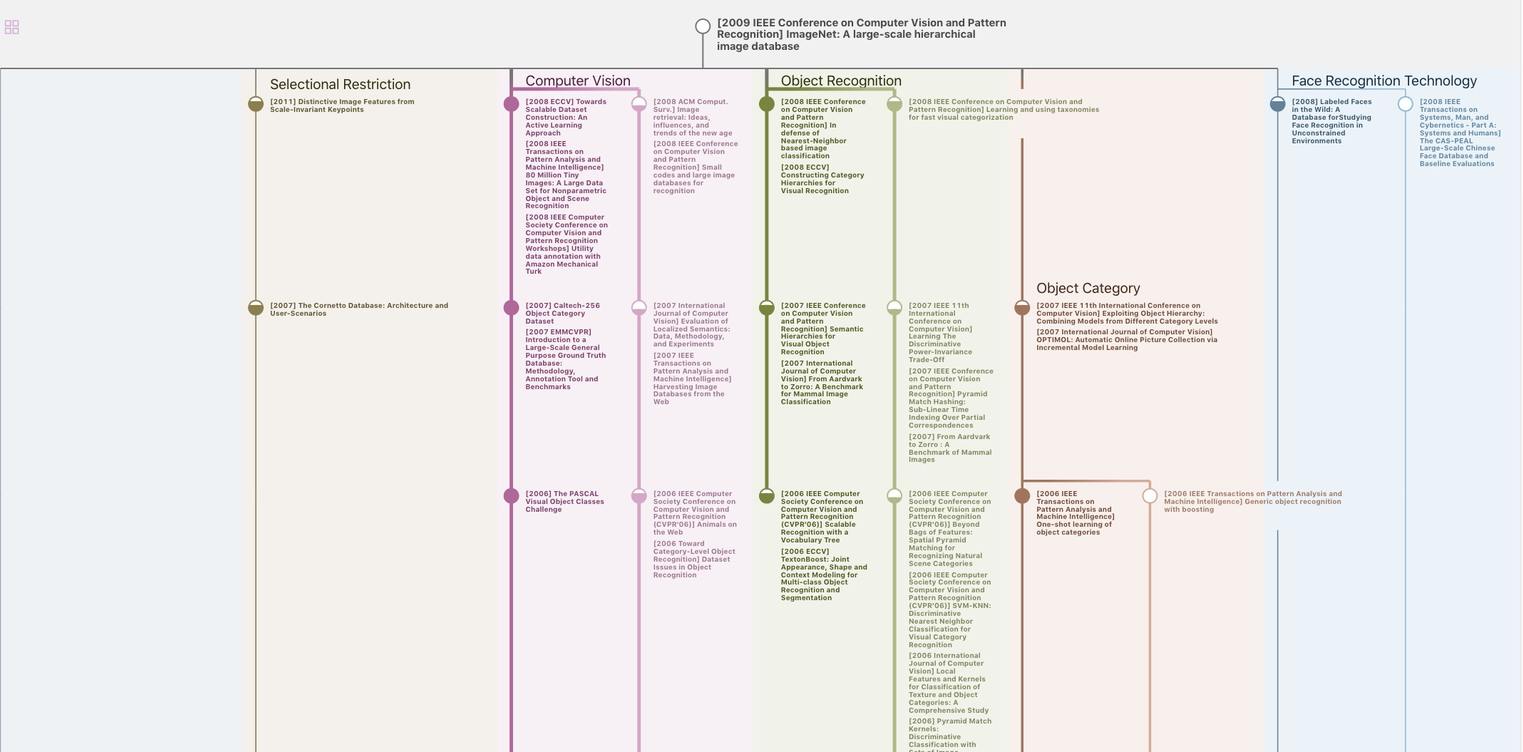
生成溯源树,研究论文发展脉络
Chat Paper
正在生成论文摘要