Learning-Based Genetic Algorithm to Schedule an Extended Flexible Job Shop
IEEE TRANSACTIONS ON CYBERNETICS(2024)
摘要
This work considers an extended flexible job-shop scheduling problem from a semiconductor manufacturing environment. To find its high-quality solution in a reasonable time, a learning-based genetic algorithm (LGA) that incorporates a parallel long short-term memory network-embedded autoencoder model is proposed. In it, genetic algorithm is selected as a main optimizer. A novel autoencoder model is trained offline via end-to-end unsupervised learning without relying on labeled data. This model captures the major linkages among decision variables and generates promising solutions in an informative low-dimensional space, striking a balance between computational efficiency and solution quality. To further improve its search ability, a co-evolving framework is designed, which includes both a network-embedded subpopulation and a regular one. The former focuses on its global search while the latter ensures LGA's convergence. An information exchange method between the two subpopulations balances global and local search, improving its overall optimization ability. This work conducts various numerical experiments to compare LGA with the CPLEX optimizer, several classical heuristics, and some popular methods. Results show that LGA outperforms its peers in finding high-quality solutions in a reasonable time.
更多查看译文
关键词
Metaheuristics,Optimization,Genetic algorithms,Schedules,Long short term memory,Job shop scheduling,Semiconductor device modeling,Autoencoder,flexible job shop scheduling,genetic algorithm (GA),long short term memory,machine learning
AI 理解论文
溯源树
样例
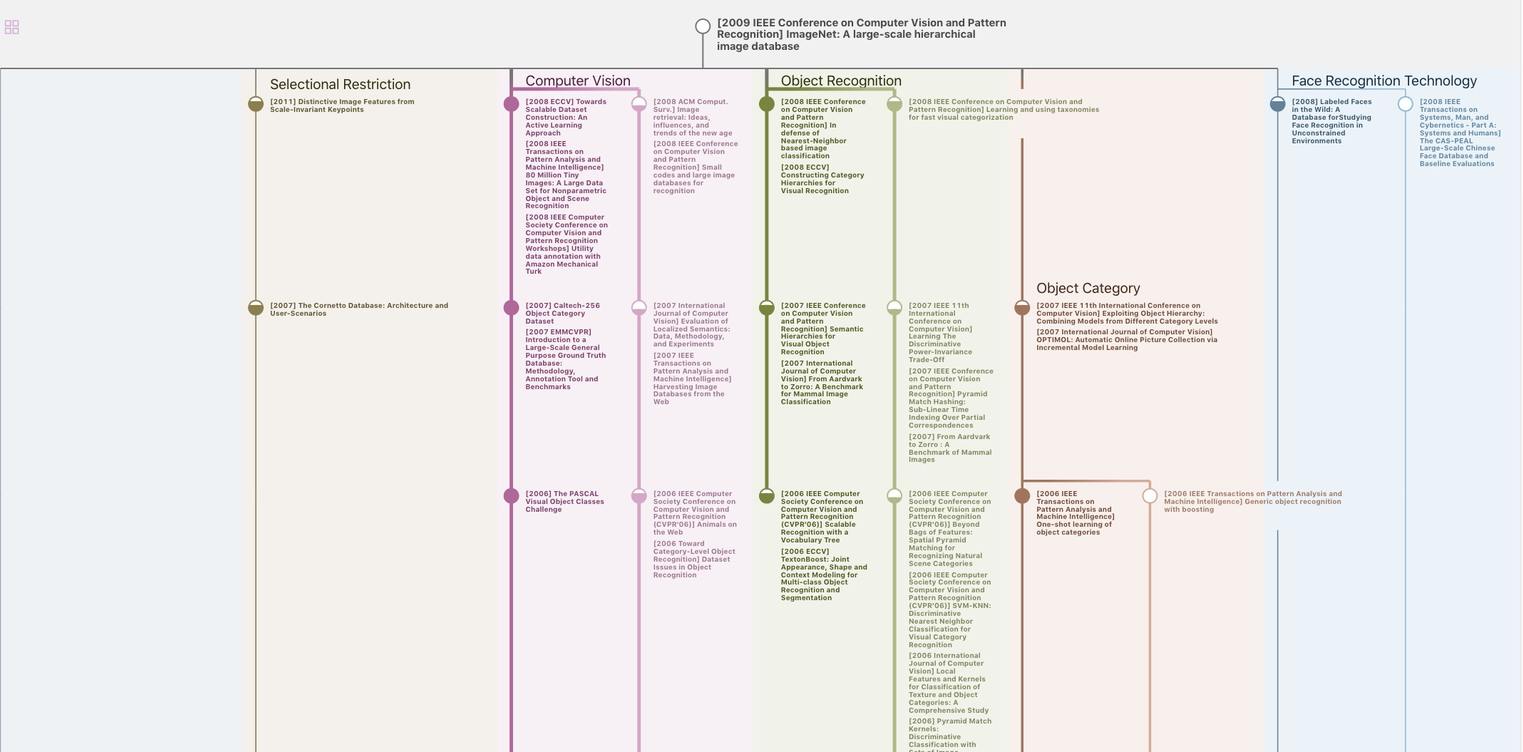
生成溯源树,研究论文发展脉络
Chat Paper
正在生成论文摘要