Toxicity prediction using locality-sensitive deep learner
Computational Toxicology(2022)
摘要
• Large chemical toxicity datasets may have locally-linear data structure. • LSDL uses attention to learn from dataset with many local chemical neighborhoods. • LSDL could use instance-based feature weighting to tackle locally-varying noise. • New algorithms for toxicity prediction add diversity to current modeling approaches. Toxicity prediction using linear QSAR models typically show good predictivity when trained on a small-scale, local level of similar chemicals, but not on a global level spanning a chemical library. We hypothesize that large chemical toxicity datasets generally have a locally-linear data structure, and propose the locality-sensitive deep learner (LSDL), a deep neural network with attention mechanism [1] and an optional instance-based feature weighting component, to tackle the challenges of heterogeneous classification space with locally-varying noise features. On carefully-constructed synthetic data with extremely unbalanced classes (10% positive), the locality-sensitive deep learner with learned feature weights retained high test performance (AUC > 0.9) in the presence of 60% cluster-specific feature noise, while feed-forward neural network appeared to over-fit the data (AUC < 0.6). For the Tox21 dataset [2] , locality-sensitive deep learner out-performed feed-forward neural network in 9 out of 12 labels. For acetylcholinesterase inhibition (AChEi) [3] , Collaborative Modeling Project for Androgen Receptor Activity (CoMPARA) [4] , and Acute Oral Toxicity (AOT) [5] datasets, we observed that the combination of locality-sensitive deep learner with feed-forward neural network showed improved test performance than individual models in almost all cases. Generalizing machine learning models to fit locally-linear data may potentially improve predictivity of chemical toxicity models. The proposed modeling approach could potentially complement and add diversity to the current suite of predictive toxicity algorithms for use in ensemble and/or consensus models.
更多查看译文
AI 理解论文
溯源树
样例
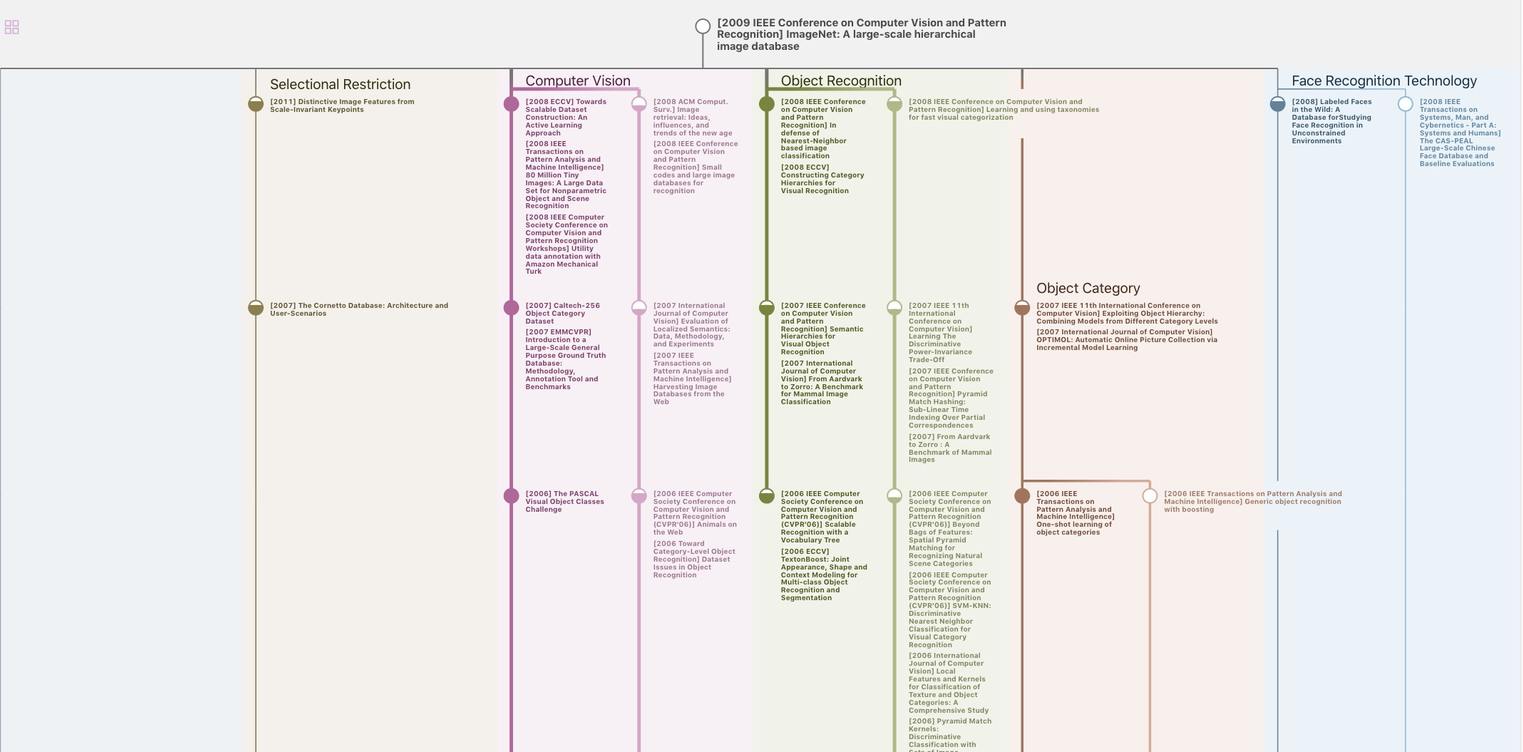
生成溯源树,研究论文发展脉络
Chat Paper
正在生成论文摘要