Monitoring of Inland Water Levels by Satellite Altimetry and Deep Learning
IEEE TRANSACTIONS ON GEOSCIENCE AND REMOTE SENSING(2022)
摘要
Deep convolutional neural networks (NNs) have proven their efficiency for image processing and are routinely used for image classification. In this article, we use them to convert radar measurements into water distance and ultimately into water levels of inland waterbodies. The measurements used are the successive echoes of the spaceborne radar altimeter signal on a waterbody, the radargram. We show that by using forward modeling with an accurate altimetry simulator, we can generate a sufficient amount of radargrams and train a deep NN accurately enough to obtain water level series from radargrams in a hydrology context. The method is validated at selected waterbodies by comparing these water level time series with in situ measurements on rivers whose width varied between 50 m and 4 km. The correlation of these time series with in situ data was over 0.95 with root mean square error (RMSE) between 26 and 43 cm. The results were also more robust than the Offset Center of Gravity (OCOG)/Ice-1 retracker time series of the same data. The validation shows that this automatic method performs generally as well as a carefully tuned manual method for removing outliers from the ranges provided by the state of the art classical retrackers used by the spatial hydrology community. This new tool is a big step toward a generic, global, and automated method to retrieve inland water levels from altimetry measurements. This goal is especially important in the context of continuously declining number of in situ measurements and of utmost importance for adequate water resources management at the global scale.
更多查看译文
关键词
Radar,Altimetry,Spaceborne radar,Satellites,Hydrology,Sea measurements,Radar tracking,Artificial intelligence (AI),deep learning,hydrology,Jason-3,neural network (NN),radar,satellite altimetry
AI 理解论文
溯源树
样例
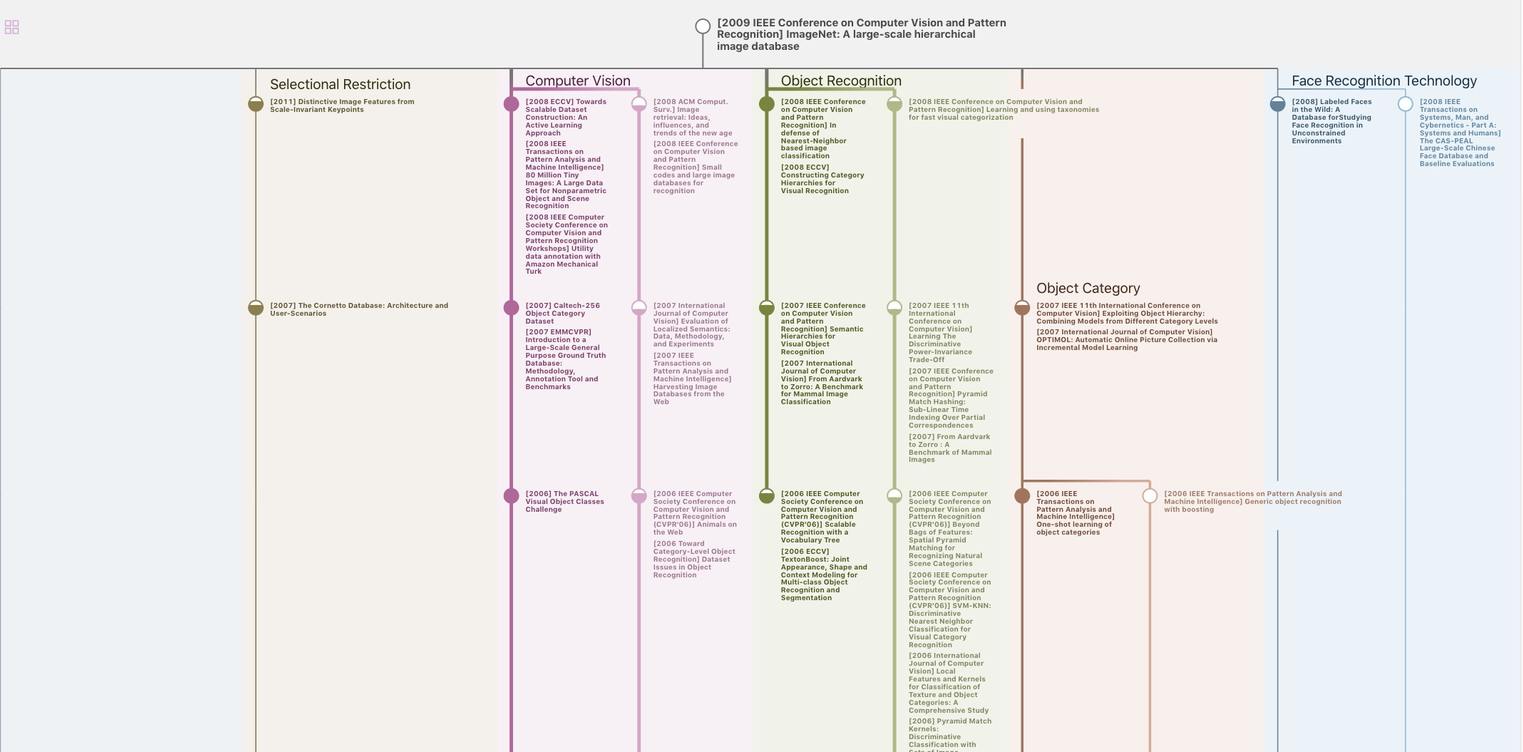
生成溯源树,研究论文发展脉络
Chat Paper
正在生成论文摘要