A Short-Term Traffic Flow Prediction Model Based on an Improved Gate Recurrent Unit Neural Network
IEEE TRANSACTIONS ON INTELLIGENT TRANSPORTATION SYSTEMS(2022)
摘要
With the increasing demand for intelligent transportation systems, short-term traffic flow prediction has become an important research direction. The memory unit of a Long Short-Term Memory (LSTM) neural network can store data characteristics over a certain period of time, hence the suitability of this network for time series processing. This paper uses an improved Gate Recurrent Unit (GRU) neural network to study the time series of traffic parameter flows. The LSTM short-term traffic flow prediction based on the flow series is first investigated, and then the GRU model is introduced. The GRU can be regarded as a simplified LSTM. After extracting the spatial and temporal characteristics of the flow matrix, an improved GRU with a bidirectional positive and negative feedback called the Bi-GRU prediction model is used to complete the short-term traffic flow prediction and study its characteristics. The Rectified Adaptive (RAdam) model is adopted to improve the shortcomings of the common optimizer. The cosine learning rate attenuation is also used for the model to avoid converging to the local optimal solution and for the appropriate convergence speed to be controlled. Furthermore, the scientific and reliable model learning rate is set together with the adaptive learning rate in RAdam. In this manner, the accuracy of network prediction can be further improved. Finally, an experiment of the Bi-GRU model is conducted. The comprehensive Bi-GRU prediction results demonstrate the effectiveness of the proposed method.
更多查看译文
关键词
Predictive models, Adaptation models, Time series analysis, Prediction algorithms, Mathematical model, Computational modeling, Recurrent neural networks, LSTM, GRU, RAdam, cosine learning rate, short-term traffic flow prediction
AI 理解论文
溯源树
样例
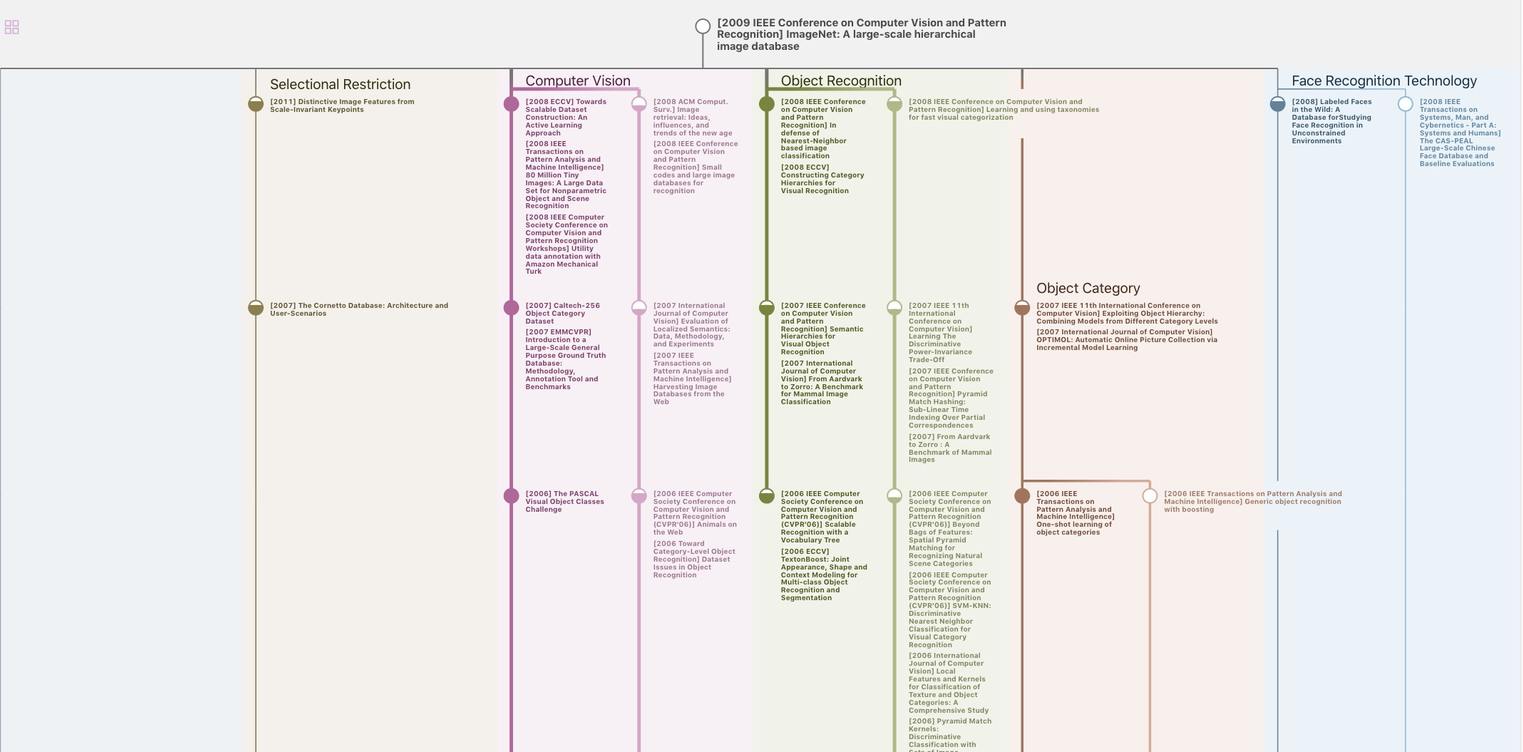
生成溯源树,研究论文发展脉络
Chat Paper
正在生成论文摘要